On the Compositional Generalization of Multimodal LLMs for Medical Imaging
Zhenyang Cai, Junying Chen, Rongsheng Wang, Weihong Wang, Yonglin Deng, Dingjie Song, Yize Chen, Zixu Zhang, Benyou Wang
2024-12-31
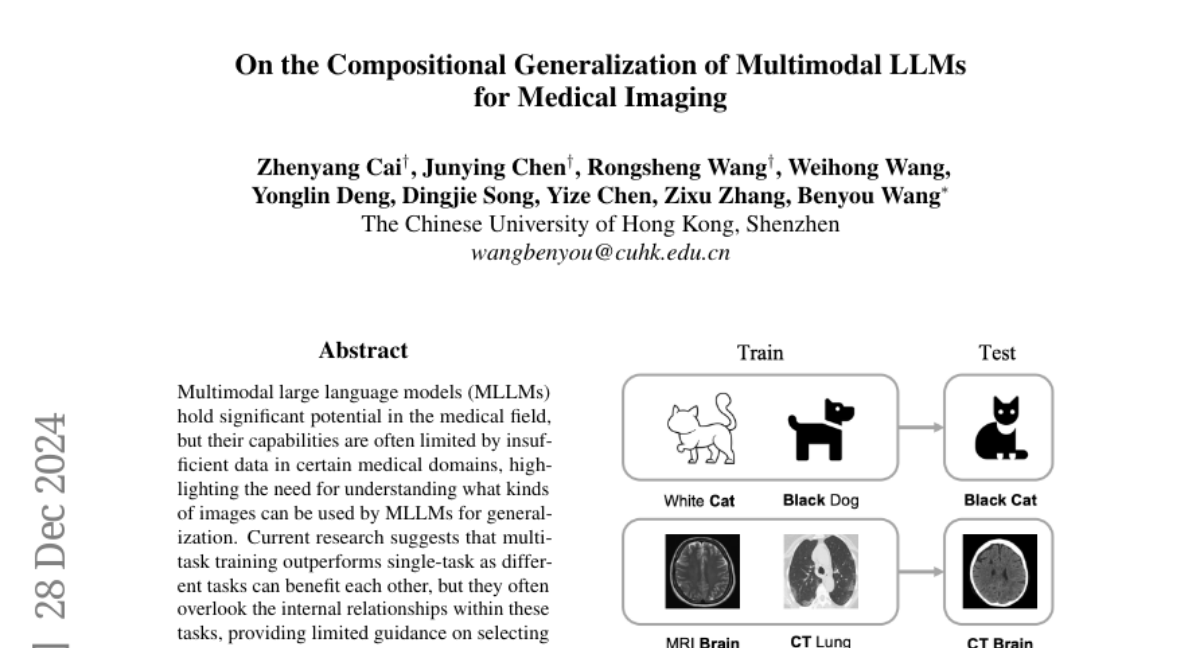
Summary
This paper talks about how to improve the ability of multimodal large language models (MLLMs) to understand and generalize across different medical imaging tasks by using a concept called compositional generalization.
What's the problem?
MLLMs have great potential in the medical field, but they often struggle to apply their knowledge to new tasks because they rely on strict definitions of tasks, like 'image segmentation.' This limits their ability to generalize and perform well on unfamiliar tasks, especially when there isn't enough data available for training.
What's the solution?
To address this issue, the authors propose using compositional generalization (CG), which is the ability of models to understand new combinations of learned elements. They created a dataset called Med-MAT, which consists of 106 medical datasets that help explore CG in medical imaging. By training MLLMs with this dataset, they found that these models could effectively understand and work with unseen medical images, improving their performance across various tasks without needing additional data.
Why it matters?
This research is important because it enhances how AI can be used in medicine by enabling models to better adapt to new situations and tasks. By improving the generalization capabilities of MLLMs, this approach could lead to better diagnostic tools and support for healthcare professionals, ultimately improving patient care and outcomes.
Abstract
Multimodal large language models (MLLMs) hold significant potential in the medical field, but their capabilities are often limited by insufficient data in certain medical domains, highlighting the need for understanding what kinds of images can be used by MLLMs for generalization. Current research suggests that multi-task training outperforms single-task as different tasks can benefit each other, but they often overlook the internal relationships within these tasks, providing limited guidance on selecting datasets to enhance specific tasks. To analyze this phenomenon, we attempted to employ compositional generalization (CG)-the ability of models to understand novel combinations by recombining learned elements-as a guiding framework. Since medical images can be precisely defined by Modality, Anatomical area, and Task, naturally providing an environment for exploring CG. Therefore, we assembled 106 medical datasets to create Med-MAT for comprehensive experiments. The experiments confirmed that MLLMs can use CG to understand unseen medical images and identified CG as one of the main drivers of the generalization observed in multi-task training. Additionally, further studies demonstrated that CG effectively supports datasets with limited data and delivers consistent performance across different backbones, highlighting its versatility and broad applicability. Med-MAT is publicly available at https://github.com/FreedomIntelligence/Med-MAT.