PERSE: Personalized 3D Generative Avatars from A Single Portrait
Hyunsoo Cha, Inhee Lee, Hanbyul Joo
2024-12-31
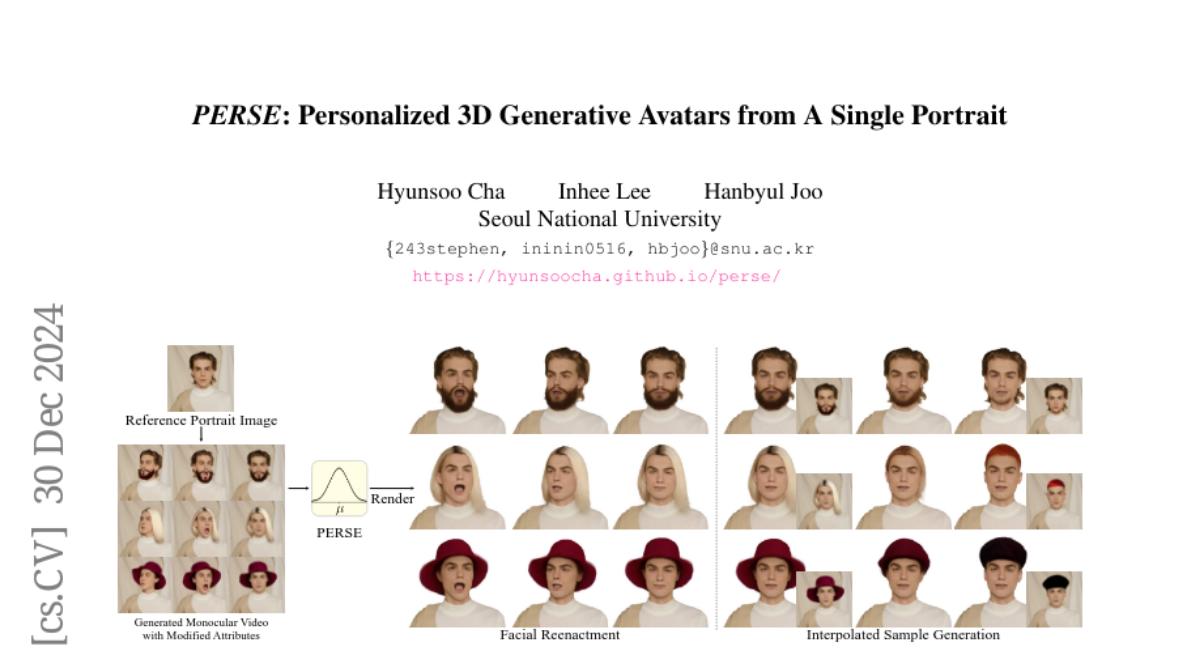
Summary
This paper talks about PERSE, a new method for creating personalized 3D avatars from a single portrait photo, allowing users to animate and customize their avatars easily.
What's the problem?
Creating realistic 3D avatars typically requires multiple images or complex 3D scans, which can be difficult and time-consuming. Existing methods often struggle to capture the unique features of a person while allowing for easy customization of facial attributes like hair and expressions.
What's the solution?
To solve this problem, the authors developed PERSE, which uses a single portrait to generate an animatable 3D avatar. They create a large dataset of synthetic videos that show different facial expressions and angles based on the original photo. This dataset helps the model learn how to manipulate facial features while keeping the person's identity intact. The method allows for smooth transitions between different facial attributes and makes it easy to edit the avatar's appearance.
Why it matters?
This research is important because it simplifies the process of creating personalized avatars, making it accessible to more people. The ability to generate realistic avatars from just one photo opens up new possibilities in areas like gaming, virtual reality, and online communication, where users can represent themselves more accurately and interactively.
Abstract
We present PERSE, a method for building an animatable personalized generative avatar from a reference portrait. Our avatar model enables facial attribute editing in a continuous and disentangled latent space to control each facial attribute, while preserving the individual's identity. To achieve this, our method begins by synthesizing large-scale synthetic 2D video datasets, where each video contains consistent changes in the facial expression and viewpoint, combined with a variation in a specific facial attribute from the original input. We propose a novel pipeline to produce high-quality, photorealistic 2D videos with facial attribute editing. Leveraging this synthetic attribute dataset, we present a personalized avatar creation method based on the 3D Gaussian Splatting, learning a continuous and disentangled latent space for intuitive facial attribute manipulation. To enforce smooth transitions in this latent space, we introduce a latent space regularization technique by using interpolated 2D faces as supervision. Compared to previous approaches, we demonstrate that PERSE generates high-quality avatars with interpolated attributes while preserving identity of reference person.