3D Reconstruction with Spatial Memory
Hengyi Wang, Lourdes Agapito
2024-08-30
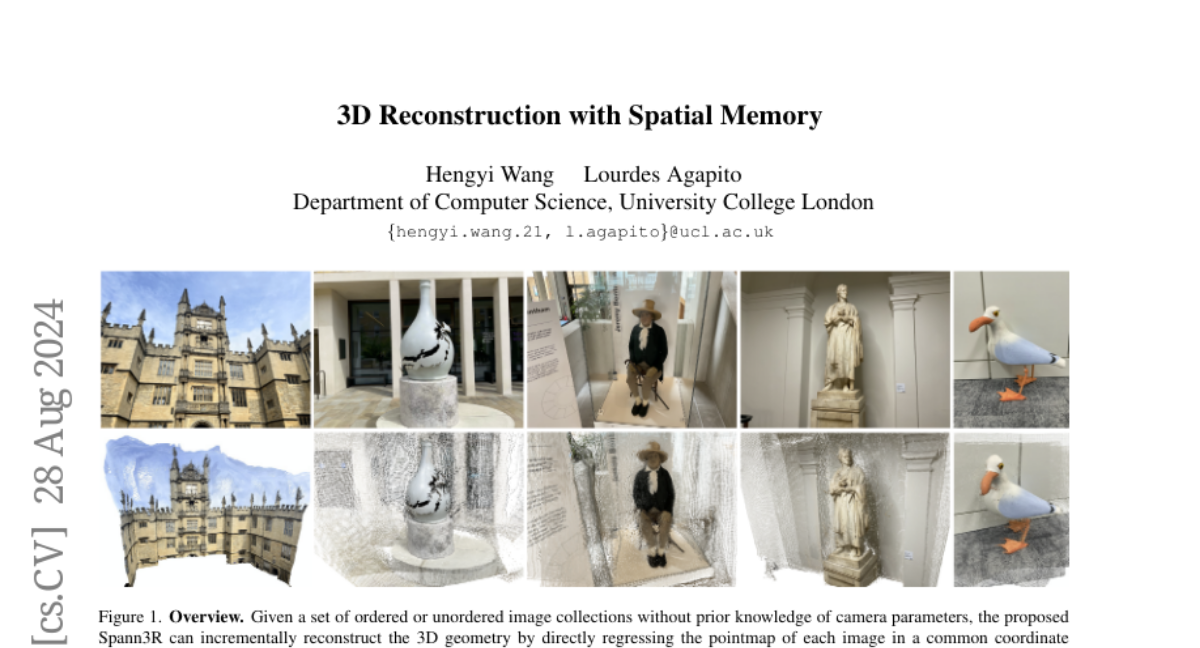
Summary
This paper presents Spann3R, a new method for creating detailed 3D models from images, using a transformer-based approach that remembers previous 3D information.
What's the problem?
Creating accurate 3D models from images can be difficult, especially when the images are not organized or when there aren't enough of them. Existing methods often require complex calculations to align different images, which can lead to errors and inconsistencies in the final 3D model.
What's the solution?
Spann3R solves this problem by using a transformer architecture that can directly create pointmaps (representations of points in 3D space) from images without needing to know the camera settings or scene details beforehand. It manages an external memory that keeps track of all the relevant 3D information from previous images. This allows Spann3R to generate a consistent 3D structure for each new image based on the overall scene rather than just individual images.
Why it matters?
This research is important because it simplifies the process of building 3D models, making it faster and more efficient. This can be useful in various fields such as virtual reality, gaming, and robotics, where accurate 3D representations are essential for creating immersive experiences.
Abstract
We present Spann3R, a novel approach for dense 3D reconstruction from ordered or unordered image collections. Built on the DUSt3R paradigm, Spann3R uses a transformer-based architecture to directly regress pointmaps from images without any prior knowledge of the scene or camera parameters. Unlike DUSt3R, which predicts per image-pair pointmaps each expressed in its local coordinate frame, Spann3R can predict per-image pointmaps expressed in a global coordinate system, thus eliminating the need for optimization-based global alignment. The key idea of Spann3R is to manage an external spatial memory that learns to keep track of all previous relevant 3D information. Spann3R then queries this spatial memory to predict the 3D structure of the next frame in a global coordinate system. Taking advantage of DUSt3R's pre-trained weights, and further fine-tuning on a subset of datasets, Spann3R shows competitive performance and generalization ability on various unseen datasets and can process ordered image collections in real time. Project page: https://hengyiwang.github.io/projects/spanner