3DGS-Enhancer: Enhancing Unbounded 3D Gaussian Splatting with View-consistent 2D Diffusion Priors
Xi Liu, Chaoyi Zhou, Siyu Huang
2024-10-23
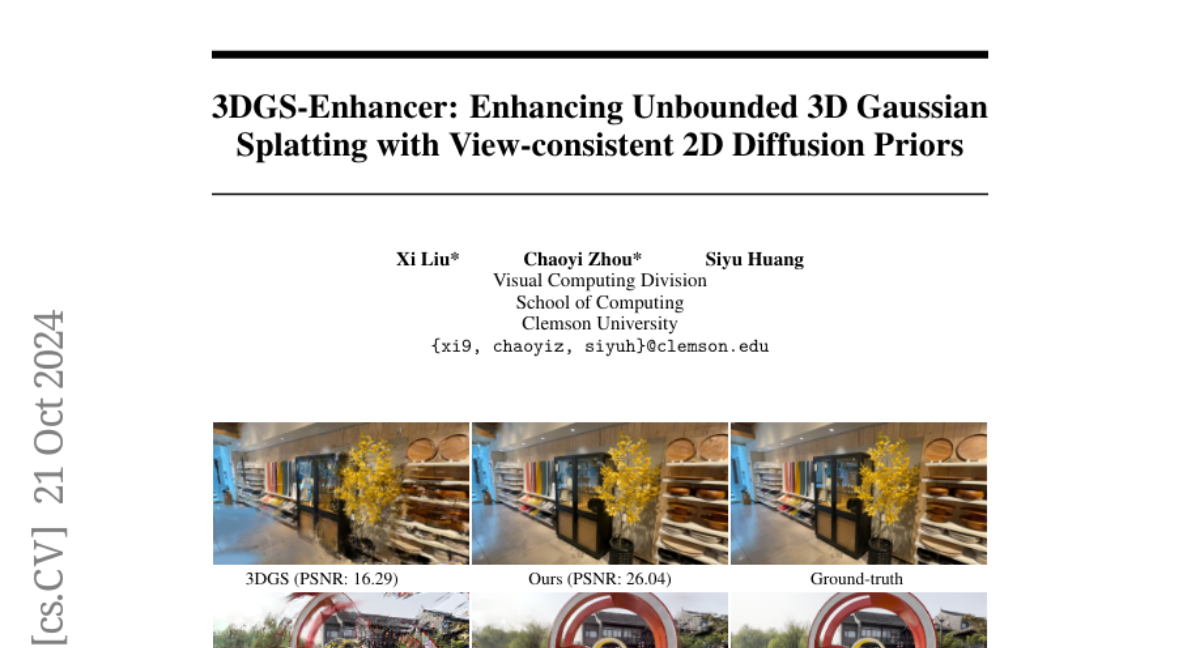
Summary
This paper presents 3DGS-Enhancer, a new method that improves the quality of 3D images generated from multiple input images or videos by making them more consistent and realistic.
What's the problem?
Creating new views of a scene (like a 3D image) from just a few pictures can be challenging, especially when there aren't enough images to provide complete information. This often leads to poor-quality images with noticeable flaws or artifacts, particularly in areas where the input views are sparse.
What's the solution?
The authors developed 3DGS-Enhancer, which uses advanced techniques to enhance the rendering of 3D images. It incorporates 2D video diffusion priors to ensure that the generated views remain consistent and realistic across different angles. The method improves how the model understands and integrates information from the input images, resulting in higher quality outputs. By fine-tuning the original 3D Gaussian splatting model with these enhanced views, they significantly improve its performance.
Why it matters?
This research is important because it helps create better and more realistic 3D renderings from limited input data. Improved rendering techniques can be applied in various fields, such as gaming, virtual reality, and film, making the visual experiences more immersive and convincing.
Abstract
Novel-view synthesis aims to generate novel views of a scene from multiple input images or videos, and recent advancements like 3D Gaussian splatting (3DGS) have achieved notable success in producing photorealistic renderings with efficient pipelines. However, generating high-quality novel views under challenging settings, such as sparse input views, remains difficult due to insufficient information in under-sampled areas, often resulting in noticeable artifacts. This paper presents 3DGS-Enhancer, a novel pipeline for enhancing the representation quality of 3DGS representations. We leverage 2D video diffusion priors to address the challenging 3D view consistency problem, reformulating it as achieving temporal consistency within a video generation process. 3DGS-Enhancer restores view-consistent latent features of rendered novel views and integrates them with the input views through a spatial-temporal decoder. The enhanced views are then used to fine-tune the initial 3DGS model, significantly improving its rendering performance. Extensive experiments on large-scale datasets of unbounded scenes demonstrate that 3DGS-Enhancer yields superior reconstruction performance and high-fidelity rendering results compared to state-of-the-art methods. The project webpage is https://xiliu8006.github.io/3DGS-Enhancer-project .