3DGS-LM: Faster Gaussian-Splatting Optimization with Levenberg-Marquardt
Lukas Höllein, Aljaž Božič, Michael Zollhöfer, Matthias Nießner
2024-09-20
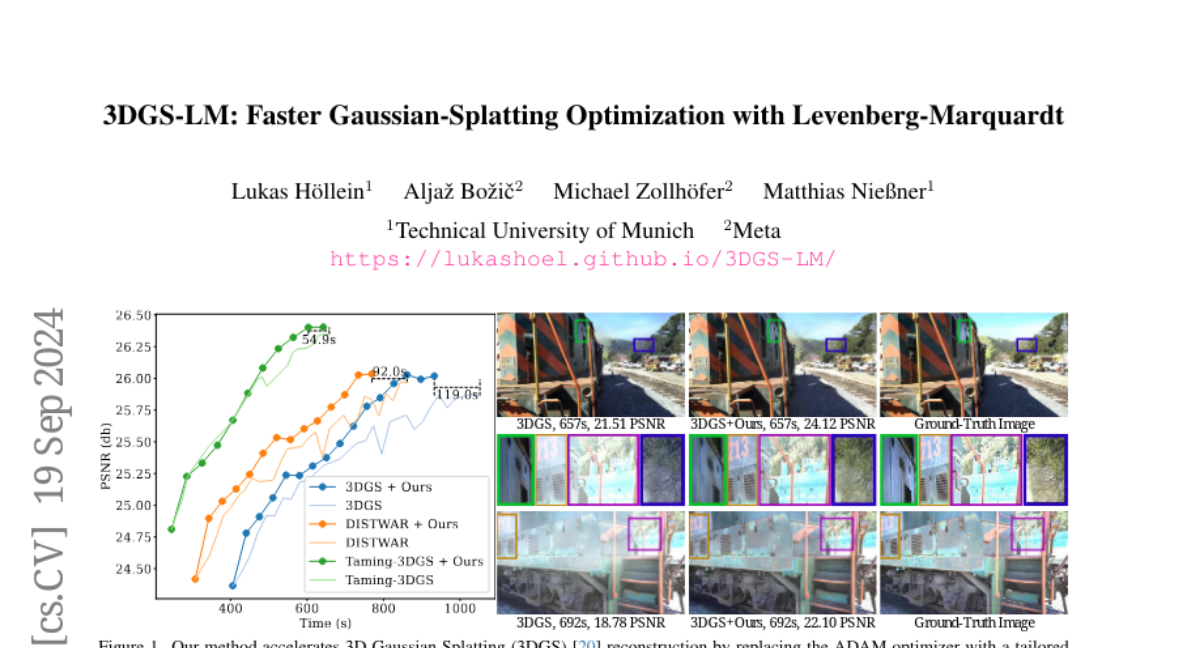
Summary
This paper presents a new method called 3DGS-LM that makes the process of creating 3D images faster and more efficient by using a different optimization technique. It focuses on improving the speed of Gaussian splatting, a technique used in computer graphics to represent 3D scenes.
What's the problem?
The main issue with existing methods for Gaussian splatting is that they take a long time to optimize the parameters needed to create accurate 3D images. Traditional approaches rely on an optimizer called ADAM, which can require thousands of iterations and take up to an hour to complete, making it inefficient for real-time applications.
What's the solution?
To solve this problem, the researchers replaced the ADAM optimizer with a method called Levenberg-Marquardt (LM), which is specifically designed to speed up optimization processes. They also developed a new way to handle data on graphics processing units (GPUs) that allows for faster calculations during the optimization process. As a result, their method, 3DGS-LM, is able to produce high-quality 3D images about 30% faster than previous methods while maintaining the same level of accuracy.
Why it matters?
This improvement is significant because it allows for quicker rendering of 3D scenes, which is crucial for applications like video games, virtual reality, and robotics where speed and efficiency are essential. By making this process faster, it opens up new possibilities for creating realistic and interactive 3D environments in real-time.
Abstract
We present 3DGS-LM, a new method that accelerates the reconstruction of 3D Gaussian Splatting (3DGS) by replacing its ADAM optimizer with a tailored Levenberg-Marquardt (LM). Existing methods reduce the optimization time by decreasing the number of Gaussians or by improving the implementation of the differentiable rasterizer. However, they still rely on the ADAM optimizer to fit Gaussian parameters of a scene in thousands of iterations, which can take up to an hour. To this end, we change the optimizer to LM that runs in conjunction with the 3DGS differentiable rasterizer. For efficient GPU parallization, we propose a caching data structure for intermediate gradients that allows us to efficiently calculate Jacobian-vector products in custom CUDA kernels. In every LM iteration, we calculate update directions from multiple image subsets using these kernels and combine them in a weighted mean. Overall, our method is 30% faster than the original 3DGS while obtaining the same reconstruction quality. Our optimization is also agnostic to other methods that acclerate 3DGS, thus enabling even faster speedups compared to vanilla 3DGS.