3DTopia-XL: Scaling High-quality 3D Asset Generation via Primitive Diffusion
Zhaoxi Chen, Jiaxiang Tang, Yuhao Dong, Ziang Cao, Fangzhou Hong, Yushi Lan, Tengfei Wang, Haozhe Xie, Tong Wu, Shunsuke Saito, Liang Pan, Dahua Lin, Ziwei Liu
2024-09-20
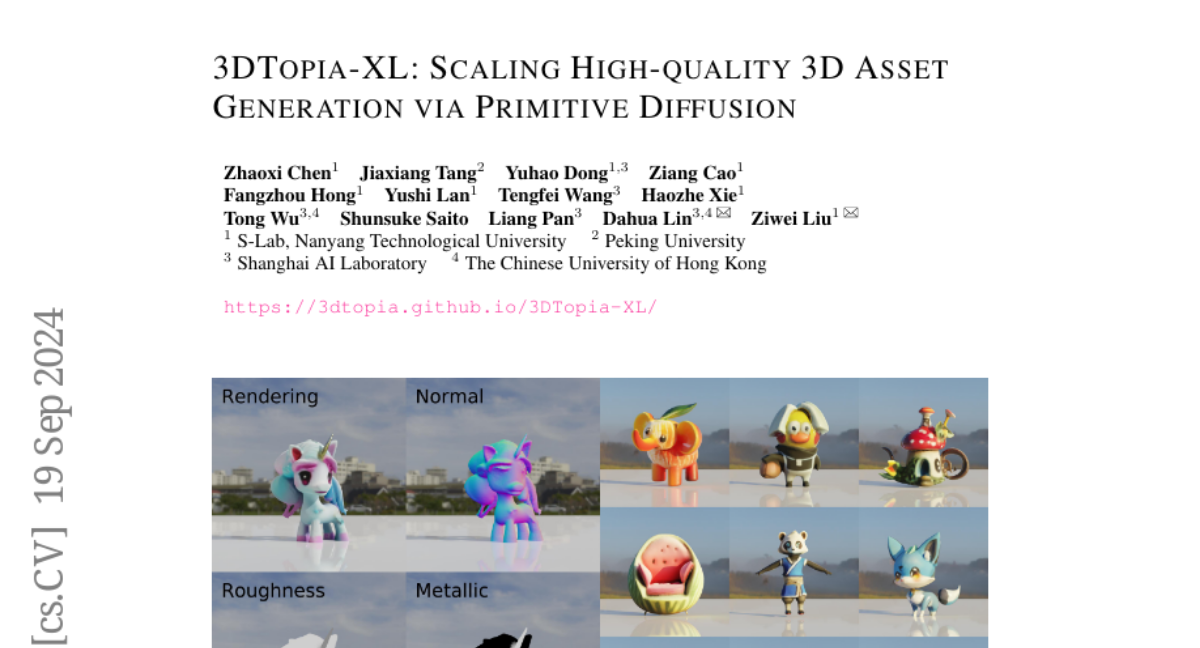
Summary
This paper introduces 3DTopia-XL, a new model designed to create high-quality 3D assets more efficiently by using a novel approach called primitive diffusion.
What's the problem?
As the demand for high-quality 3D models grows in various industries, creating these assets can be slow and costly. Current methods struggle with speed, the quality of the shapes they produce, and often lack the necessary details for realistic rendering. This makes it hard to keep up with the need for detailed and accurate 3D content.
What's the solution?
The authors developed 3DTopia-XL, which uses a unique way to represent 3D shapes called PrimX. This method encodes detailed information about the shape and materials into a compact format, making it easier to generate high-resolution models. They also created a new generative framework based on Diffusion Transformer (DiT) that learns to create 3D assets from both text and visual inputs. Their extensive testing shows that 3DTopia-XL produces much better quality 3D models compared to existing methods, especially in terms of texture and detail.
Why it matters?
This research is important because it addresses the challenges of creating high-quality 3D models quickly and efficiently. By improving the process of generating 3D assets, 3DTopia-XL can benefit industries like gaming, film, and virtual reality, where detailed and realistic models are essential for creating immersive experiences.
Abstract
The increasing demand for high-quality 3D assets across various industries necessitates efficient and automated 3D content creation. Despite recent advancements in 3D generative models, existing methods still face challenges with optimization speed, geometric fidelity, and the lack of assets for physically based rendering (PBR). In this paper, we introduce 3DTopia-XL, a scalable native 3D generative model designed to overcome these limitations. 3DTopia-XL leverages a novel primitive-based 3D representation, PrimX, which encodes detailed shape, albedo, and material field into a compact tensorial format, facilitating the modeling of high-resolution geometry with PBR assets. On top of the novel representation, we propose a generative framework based on Diffusion Transformer (DiT), which comprises 1) Primitive Patch Compression, 2) and Latent Primitive Diffusion. 3DTopia-XL learns to generate high-quality 3D assets from textual or visual inputs. We conduct extensive qualitative and quantitative experiments to demonstrate that 3DTopia-XL significantly outperforms existing methods in generating high-quality 3D assets with fine-grained textures and materials, efficiently bridging the quality gap between generative models and real-world applications.