A Common Pitfall of Margin-based Language Model Alignment: Gradient Entanglement
Hui Yuan, Yifan Zeng, Yue Wu, Huazheng Wang, Mengdi Wang, Liu Leqi
2024-10-21
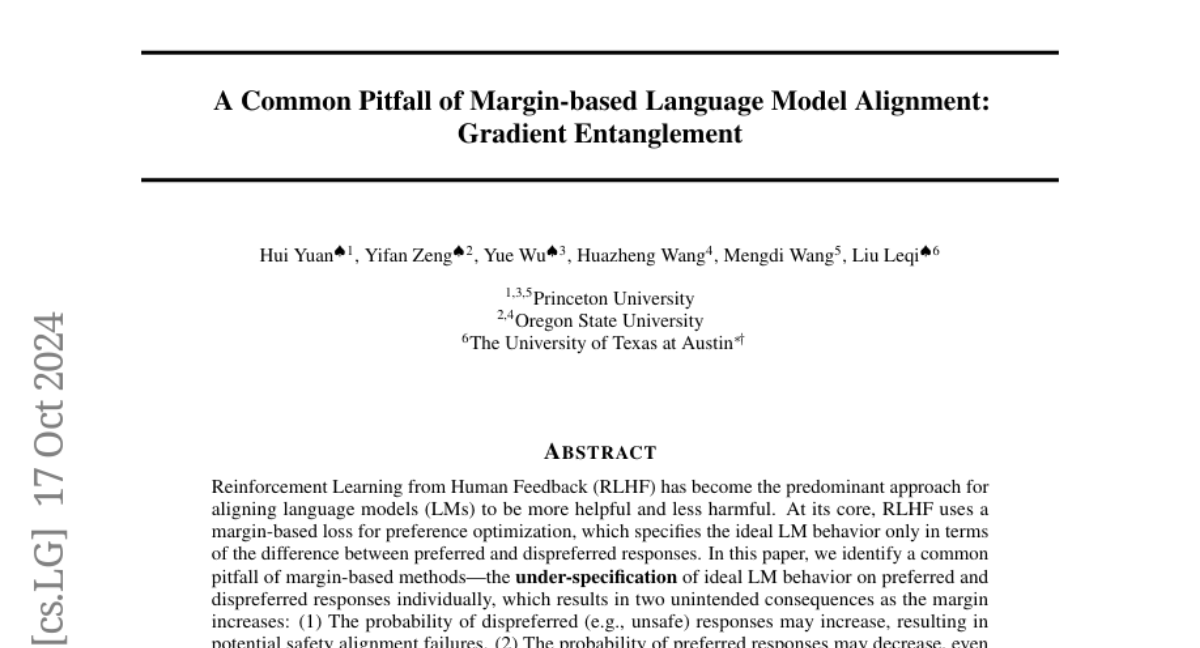
Summary
This paper discusses a problem with how language models are trained using a method called Reinforcement Learning from Human Feedback (RLHF), specifically focusing on an issue known as gradient entanglement.
What's the problem?
When training language models, researchers use a method called margin-based loss to help the model learn what responses are preferred and which ones are not. However, this approach can lead to problems where the model might start giving more unsafe or unwanted responses while also reducing the quality of its preferred responses. This happens because the way the model's learning process is structured can cause it to struggle to improve in one area without negatively impacting another, a phenomenon referred to as gradient entanglement.
What's the solution?
To address this issue, the authors of the paper investigated how margin-based methods affect the model's ability to learn from its own responses. They found that when the model tries to improve its preferred responses, it often unintentionally affects its dispreferred responses as well, leading to a situation where both types of responses become entangled. They provided theoretical insights into this problem and suggested ways to design better training methods that could reduce these negative effects and improve the alignment of language models with human preferences.
Why it matters?
This research is important because it helps improve how we train AI language models, ensuring they provide safer and more accurate responses. By understanding and addressing issues like gradient entanglement, developers can create more reliable AI systems that better align with human values and expectations, which is crucial for applications in customer service, education, and many other fields.
Abstract
Reinforcement Learning from Human Feedback (RLHF) has become the predominant approach for language model (LM) alignment. At its core, RLHF uses a margin-based loss for preference optimization, specifying ideal LM behavior only by the difference between preferred and dispreferred responses. In this paper, we identify a common pitfall of margin-based methods -- the under-specification of ideal LM behavior on preferred and dispreferred responses individually, which leads to two unintended consequences as the margin increases: (1) The probability of dispreferred (e.g., unsafe) responses may increase, resulting in potential safety alignment failures. (2) The probability of preferred responses may decrease, even when those responses are ideal. We demystify the reasons behind these problematic behaviors: margin-based losses couple the change in the preferred probability to the gradient of the dispreferred one, and vice versa, often preventing the preferred probability from increasing while the dispreferred one decreases, and thus causing a synchronized increase or decrease in both probabilities. We term this effect, inherent in margin-based objectives, gradient entanglement. Formally, we derive conditions for general margin-based alignment objectives under which gradient entanglement becomes concerning: the inner product of the gradients of preferred and dispreferred log-probabilities is large relative to the individual gradient norms. We theoretically investigate why such inner products can be large when aligning language models and empirically validate our findings. Empirical implications of our framework extend to explaining important differences in the training dynamics of various preference optimization algorithms, and suggesting potential algorithm designs to mitigate the under-specification issue of margin-based methods and thereby improving language model alignment.