A Comparative Study on Automatic Coding of Medical Letters with Explainability
Jamie Glen, Lifeng Han, Paul Rayson, Goran Nenadic
2024-07-19
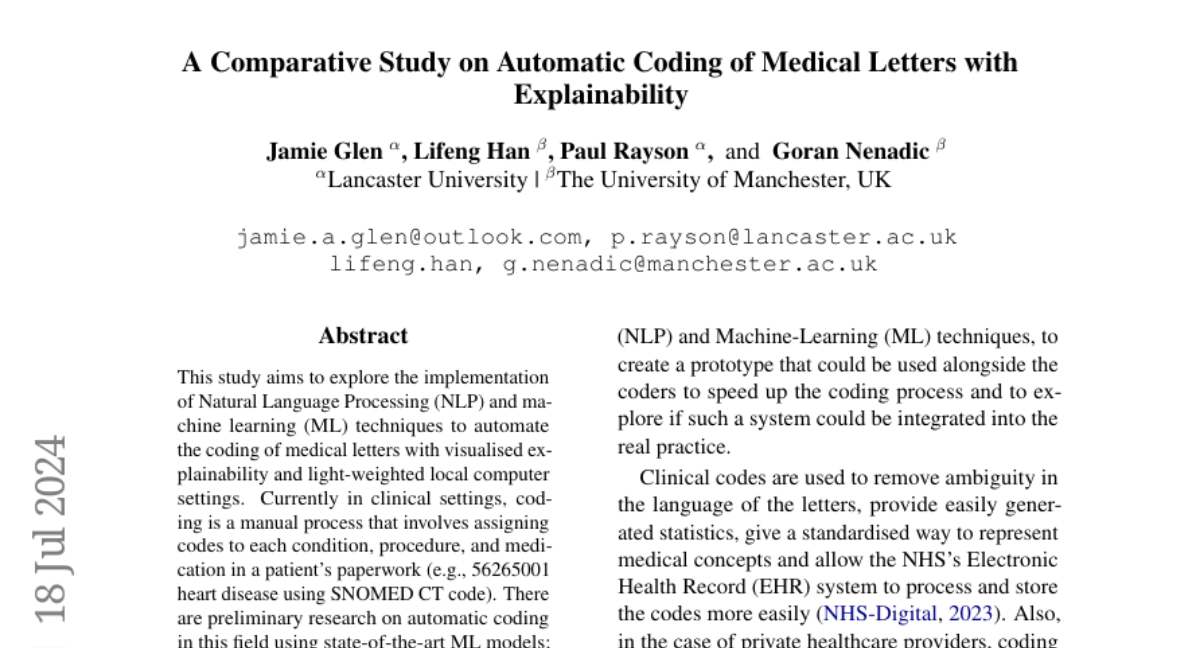
Summary
This paper explores how to automate the coding of medical letters using Natural Language Processing (NLP) and machine learning (ML) techniques. It focuses on creating a system that can accurately assign medical codes to various conditions and procedures while providing explanations for its decisions.
What's the problem?
Currently, coding medical letters is a manual process where healthcare professionals must assign specific codes to each diagnosis, treatment, and medication in patient records. This process is time-consuming and prone to errors. Existing automated coding systems often struggle due to the complexity of medical language and the lack of high-quality training data, making it difficult to implement them effectively in real-world clinical settings.
What's the solution?
The authors of the study developed a system that uses NLP and ML to automate the coding of medical letters. They utilized the MIMIC-III database, which contains a wealth of medical information, to train their models. Their approach includes visualizing how the model makes decisions (explainability) so that users can understand why certain codes are assigned. In their experiments, the models were able to provide accurate coding for 97.98% of cases, showing that their method is effective and practical for clinical use.
Why it matters?
This research is significant because it addresses a critical need in healthcare for more efficient coding processes. By automating this task, healthcare providers can save time and reduce errors, ultimately leading to better patient care. The findings also pave the way for future advancements in automated medical coding, which could improve overall efficiency in hospitals and clinics.
Abstract
This study aims to explore the implementation of Natural Language Processing (NLP) and machine learning (ML) techniques to automate the coding of medical letters with visualised explainability and light-weighted local computer settings. Currently in clinical settings, coding is a manual process that involves assigning codes to each condition, procedure, and medication in a patient's paperwork (e.g., 56265001 heart disease using SNOMED CT code). There are preliminary research on automatic coding in this field using state-of-the-art ML models; however, due to the complexity and size of the models, the real-world deployment is not achieved. To further facilitate the possibility of automatic coding practice, we explore some solutions in a local computer setting; in addition, we explore the function of explainability for transparency of AI models. We used the publicly available MIMIC-III database and the HAN/HLAN network models for ICD code prediction purposes. We also experimented with the mapping between ICD and SNOMED CT knowledge bases. In our experiments, the models provided useful information for 97.98\% of codes. The result of this investigation can shed some light on implementing automatic clinical coding in practice, such as in hospital settings, on the local computers used by clinicians , project page https://github.com/Glenj01/Medical-Coding.