A Comprehensive Survey of Mamba Architectures for Medical Image Analysis: Classification, Segmentation, Restoration and Beyond
Shubhi Bansal, Sreeharish A, Madhava Prasath J, Manikandan S, Sreekanth Madisetty, Mohammad Zia Ur Rehman, Chandravardhan Singh Raghaw, Gaurav Duggal, Nagendra Kumar
2024-10-07
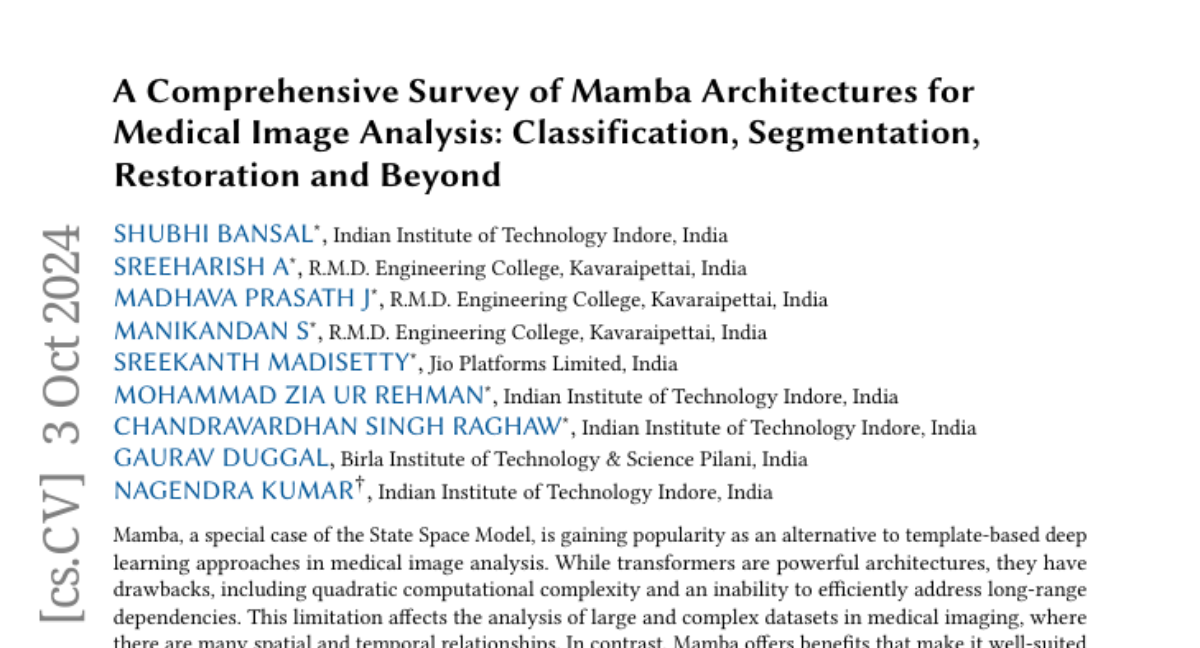
Summary
This paper provides a comprehensive overview of Mamba architectures, which are a new type of deep learning model used for analyzing medical images. It discusses their advantages over traditional methods and explores various applications in medical imaging tasks like classification, segmentation, and restoration.
What's the problem?
Traditional deep learning models, like transformers, often struggle with processing large and complex medical images due to their high computational demands and inability to efficiently manage long-range dependencies in the data. This can lead to slower performance and less accurate results when analyzing medical images, which is critical for accurate diagnoses and patient care.
What's the solution?
The authors propose Mamba architectures as a solution to these challenges. Mamba models have linear time complexity, meaning they can process longer sequences of data more quickly and with less memory than transformers. The paper reviews different types of Mamba architectures, including pure Mamba models and hybrid models that combine Mamba with other techniques like convolutional neural networks (CNNs) and graph neural networks (GNNs). By using these architectures, researchers can improve the accuracy of medical image analysis while reducing the computational burden.
Why it matters?
This research is important because it highlights a promising new approach to medical image analysis that could lead to better diagnostic tools and improved patient outcomes. By overcoming the limitations of existing deep learning methods, Mamba architectures have the potential to transform how medical images are analyzed, making it easier for healthcare professionals to make informed decisions based on accurate data.
Abstract
Mamba, a special case of the State Space Model, is gaining popularity as an alternative to template-based deep learning approaches in medical image analysis. While transformers are powerful architectures, they have drawbacks, including quadratic computational complexity and an inability to address long-range dependencies efficiently. This limitation affects the analysis of large and complex datasets in medical imaging, where there are many spatial and temporal relationships. In contrast, Mamba offers benefits that make it well-suited for medical image analysis. It has linear time complexity, which is a significant improvement over transformers. Mamba processes longer sequences without attention mechanisms, enabling faster inference and requiring less memory. Mamba also demonstrates strong performance in merging multimodal data, improving diagnosis accuracy and patient outcomes. The organization of this paper allows readers to appreciate the capabilities of Mamba in medical imaging step by step. We begin by defining core concepts of SSMs and models, including S4, S5, and S6, followed by an exploration of Mamba architectures such as pure Mamba, U-Net variants, and hybrid models with convolutional neural networks, transformers, and Graph Neural Networks. We also cover Mamba optimizations, techniques and adaptations, scanning, datasets, applications, experimental results, and conclude with its challenges and future directions in medical imaging. This review aims to demonstrate the transformative potential of Mamba in overcoming existing barriers within medical imaging while paving the way for innovative advancements in the field. A comprehensive list of Mamba architectures applied in the medical field, reviewed in this work, is available at Github.