A Preliminary Study of o1 in Medicine: Are We Closer to an AI Doctor?
Yunfei Xie, Juncheng Wu, Haoqin Tu, Siwei Yang, Bingchen Zhao, Yongshuo Zong, Qiao Jin, Cihang Xie, Yuyin Zhou
2024-09-24
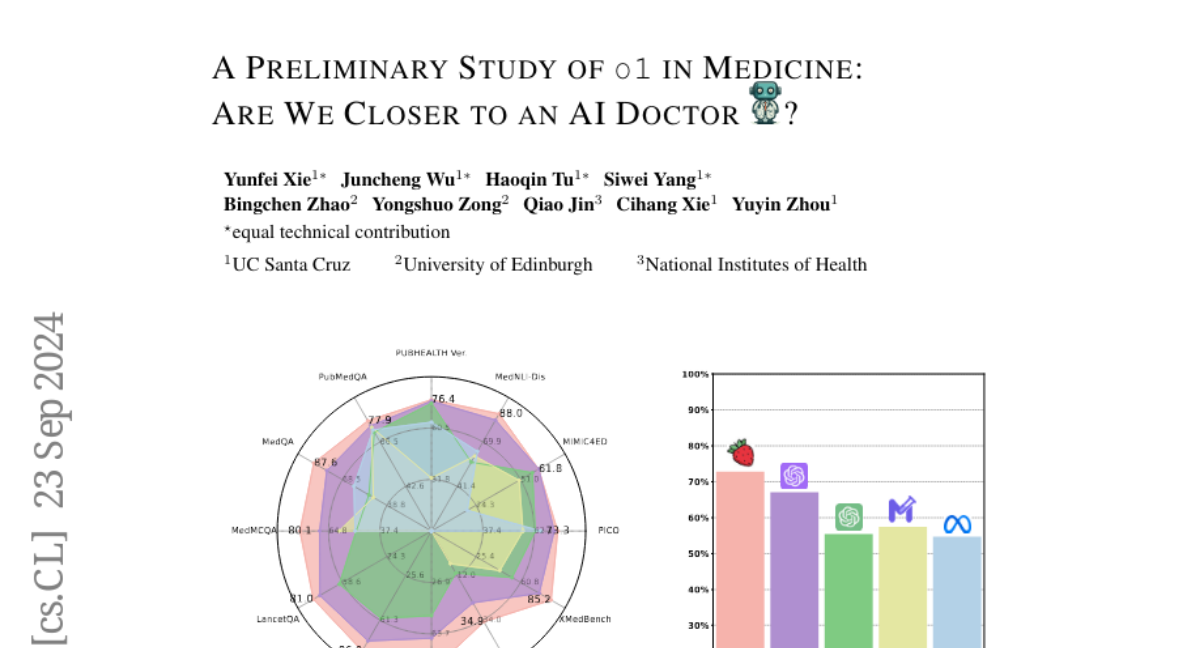
Summary
This paper discusses a study on the AI model called o1, exploring its potential to function as an 'AI doctor' in the medical field. The research evaluates how well o1 can understand medical information, reason through complex scenarios, and communicate effectively in multiple languages.
What's the problem?
While large language models (LLMs) like o1 have shown great abilities in general tasks, their effectiveness in specialized areas like medicine is still unclear. There are concerns about whether these models can accurately diagnose patients, recommend treatments, and interact with patients in a meaningful way. Additionally, existing models may struggle with understanding the nuances of medical contexts.
What's the solution?
To investigate o1's capabilities, the researchers conducted a comprehensive evaluation using 37 medical datasets, including two newly created question-answering tasks based on professional medical quizzes. They focused on three key aspects: understanding medical instructions, reasoning through clinical scenarios, and handling multilingual communication. The study found that o1 outperformed previous models like GPT-4 in accuracy and reasoning ability, but also identified weaknesses such as generating incorrect information (hallucinations) and inconsistent performance across languages.
Why it matters?
This research is significant because it moves us closer to the idea of an AI doctor that can assist or even replace human physicians in certain tasks. If successful, this technology could lead to more efficient healthcare delivery and better patient outcomes. However, it also raises important ethical questions about relying on AI for critical medical decisions, highlighting the need for further research and development in this area.
Abstract
Large language models (LLMs) have exhibited remarkable capabilities across various domains and tasks, pushing the boundaries of our knowledge in learning and cognition. The latest model, OpenAI's o1, stands out as the first LLM with an internalized chain-of-thought technique using reinforcement learning strategies. While it has demonstrated surprisingly strong capabilities on various general language tasks, its performance in specialized fields such as medicine remains unknown. To this end, this report provides a comprehensive exploration of o1 on different medical scenarios, examining 3 key aspects: understanding, reasoning, and multilinguality. Specifically, our evaluation encompasses 6 tasks using data from 37 medical datasets, including two newly constructed and more challenging question-answering (QA) tasks based on professional medical quizzes from the New England Journal of Medicine (NEJM) and The Lancet. These datasets offer greater clinical relevance compared to standard medical QA benchmarks such as MedQA, translating more effectively into real-world clinical utility. Our analysis of o1 suggests that the enhanced reasoning ability of LLMs may (significantly) benefit their capability to understand various medical instructions and reason through complex clinical scenarios. Notably, o1 surpasses the previous GPT-4 in accuracy by an average of 6.2% and 6.6% across 19 datasets and two newly created complex QA scenarios. But meanwhile, we identify several weaknesses in both the model capability and the existing evaluation protocols, including hallucination, inconsistent multilingual ability, and discrepant metrics for evaluation. We release our raw data and model outputs at https://ucsc-vlaa.github.io/o1_medicine/ for future research.