A Simple and Effective $L_2$ Norm-Based Strategy for KV Cache Compression
Alessio Devoto, Yu Zhao, Simone Scardapane, Pasquale Minervini
2024-06-18
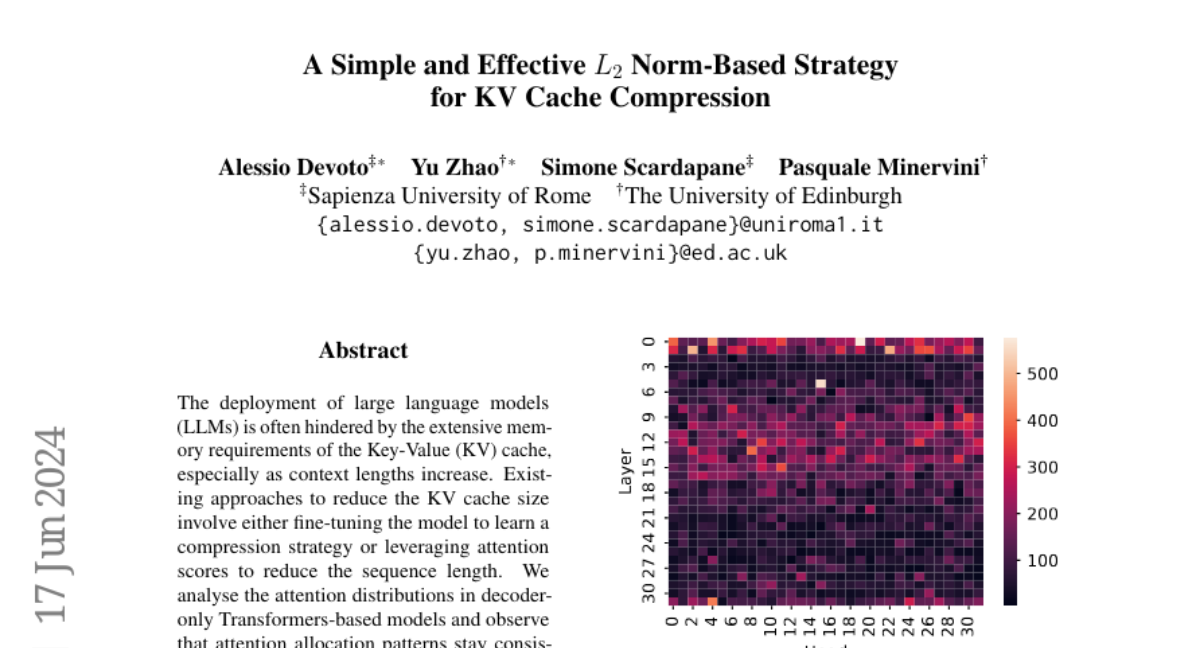
Summary
This paper presents a new method for compressing the Key-Value (KV) cache used in large language models (LLMs). The method focuses on using the $L_2$ norm, which is a mathematical way to measure the size of key embeddings, to effectively reduce the memory needed for storing these caches.
What's the problem?
Large language models require a lot of memory to store their KV cache, which is essential for quickly retrieving information during processing. As the amount of context (the text or data being processed) increases, the memory demands grow significantly. Current methods to reduce this memory usage either involve complex adjustments to the model or focus on shortening the sequence length, which can be inefficient and may not always work well.
What's the solution?
The authors discovered that there is a consistent pattern in how attention scores are allocated across different layers of decoder-only transformer models. They found that key embeddings with a low $L_2$ norm often correspond to higher attention scores during decoding, meaning that these keys are more influential. Based on this insight, they developed a straightforward compression strategy that reduces the KV cache size by focusing on the $L_2$ norm of key embeddings. Their experiments showed that this approach can cut the KV cache size by 50% for general language tasks and even by 90% for specific tasks like passkey retrieval, all while maintaining accuracy.
Why it matters?
This research is important because it provides a simple yet effective way to reduce the memory footprint of large language models without sacrificing performance. By making it easier to manage memory requirements, this method can help improve the deployment and efficiency of LLMs in various applications, such as chatbots and virtual assistants, where quick response times are crucial.
Abstract
The deployment of large language models (LLMs) is often hindered by the extensive memory requirements of the Key-Value (KV) cache, especially as context lengths increase. Existing approaches to reduce the KV cache size involve either fine-tuning the model to learn a compression strategy or leveraging attention scores to reduce the sequence length. We analyse the attention distributions in decoder-only Transformers-based models and observe that attention allocation patterns stay consistent across most layers. Surprisingly, we find a clear correlation between the L_2 and the attention scores over cached KV pairs, where a low L_2 of a key embedding usually leads to a high attention score during decoding. This finding indicates that the influence of a KV pair is potentially determined by the key embedding itself before being queried. Based on this observation, we compress the KV cache based on the L_2 of key embeddings. Our experimental results show that this simple strategy can reduce the KV cache size by 50% on language modelling and needle-in-a-haystack tasks and 90% on passkey retrieval tasks without losing accuracy.