A Survey on the Honesty of Large Language Models
Siheng Li, Cheng Yang, Taiqiang Wu, Chufan Shi, Yuji Zhang, Xinyu Zhu, Zesen Cheng, Deng Cai, Mo Yu, Lemao Liu, Jie Zhou, Yujiu Yang, Ngai Wong, Xixin Wu, Wai Lam
2024-09-30
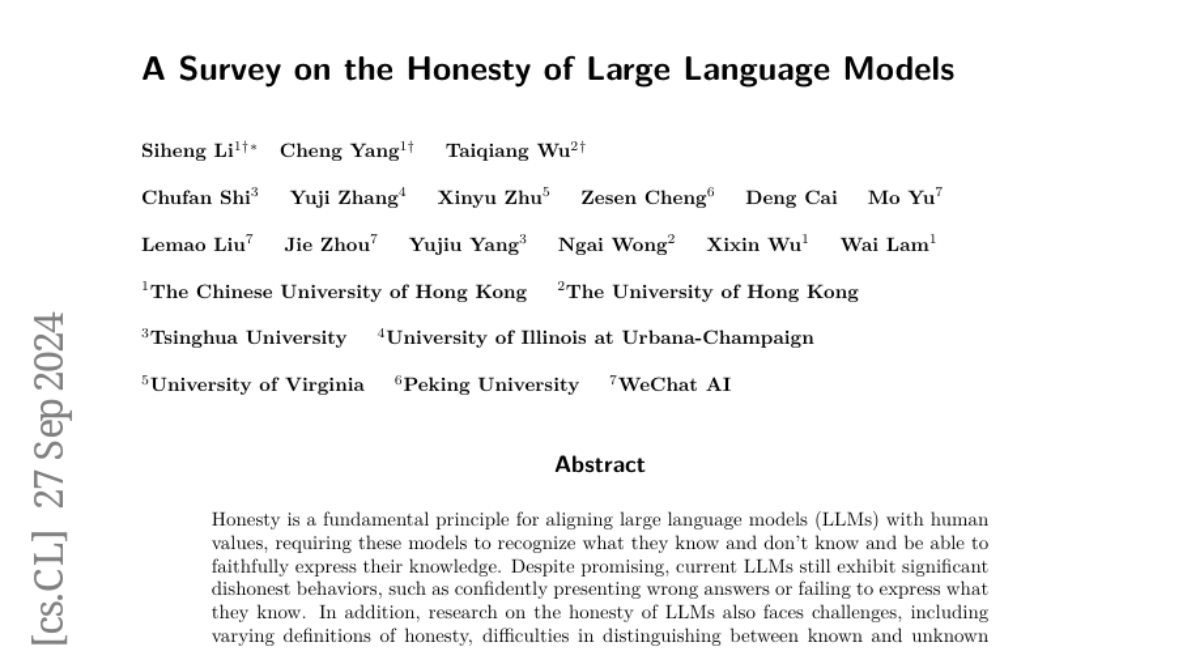
Summary
This paper reviews the honesty of Large Language Models (LLMs), discussing their ability to recognize and express what they know accurately and the challenges they face in doing so.
What's the problem?
Many LLMs often provide incorrect answers confidently or fail to indicate when they don't know something. This dishonesty can lead to misinformation and confusion, making it crucial to understand how these models can align better with human values regarding honesty.
What's the solution?
The authors conducted a survey that clarifies what honesty means for LLMs, evaluates how current models measure up, and suggests ways to improve their honesty. They highlight the need for better definitions of honesty and improved methods for assessing and enhancing LLMs' self-awareness and expression.
Why it matters?
Understanding and improving the honesty of LLMs is essential because it helps ensure that these models can be trusted to provide accurate information, which is increasingly important as they are used in more critical applications like education, healthcare, and customer service.
Abstract
Honesty is a fundamental principle for aligning large language models (LLMs) with human values, requiring these models to recognize what they know and don't know and be able to faithfully express their knowledge. Despite promising, current LLMs still exhibit significant dishonest behaviors, such as confidently presenting wrong answers or failing to express what they know. In addition, research on the honesty of LLMs also faces challenges, including varying definitions of honesty, difficulties in distinguishing between known and unknown knowledge, and a lack of comprehensive understanding of related research. To address these issues, we provide a survey on the honesty of LLMs, covering its clarification, evaluation approaches, and strategies for improvement. Moreover, we offer insights for future research, aiming to inspire further exploration in this important area.