A Web-Based Solution for Federated Learning with LLM-Based Automation
Chamith Mawela, Chaouki Ben Issaid, Mehdi Bennis
2024-08-26
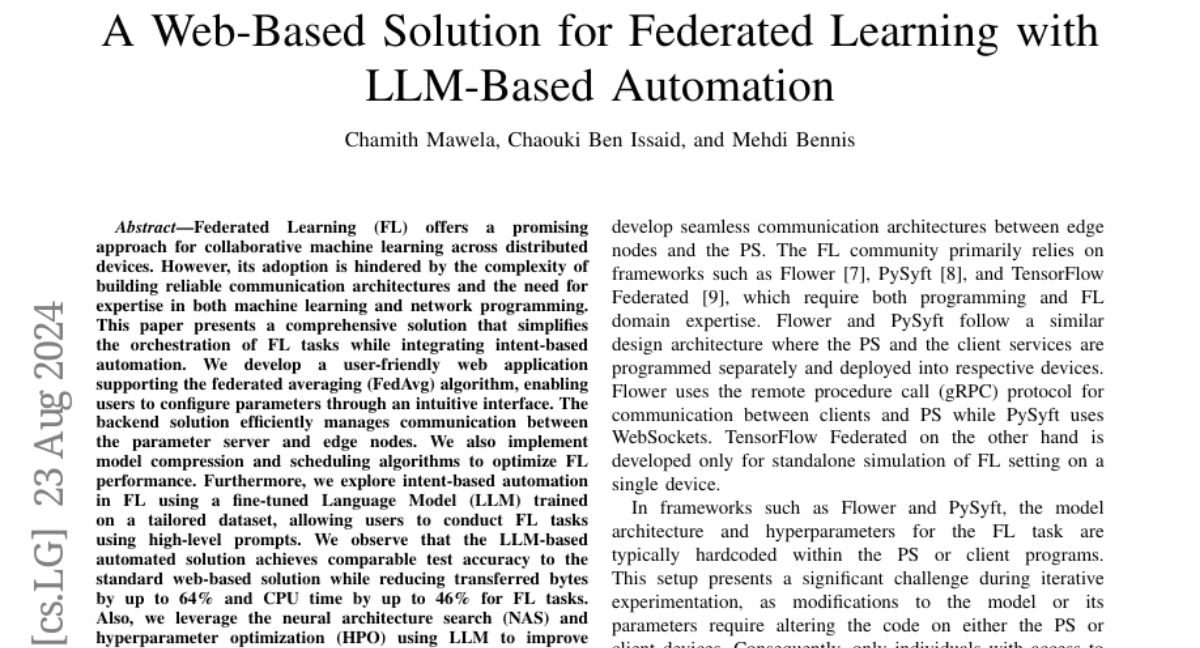
Summary
This paper discusses a new web-based solution for Federated Learning (FL) that simplifies the process of training machine learning models across different devices using automation.
What's the problem?
Federated Learning is a way to train machine learning models collaboratively across multiple devices without sharing sensitive data. However, it can be complicated to set up and requires expertise in both machine learning and networking. This complexity makes it hard for many people to use FL effectively.
What's the solution?
The authors developed a user-friendly web application that helps manage FL tasks more easily. This application uses the federated averaging (FedAvg) algorithm and allows users to adjust settings through a simple interface. They also integrated an automated system using a fine-tuned language model (LLM), which lets users perform FL tasks using easy-to-understand prompts. Their approach reduces the amount of data transferred and speeds up processing times while maintaining accuracy.
Why it matters?
This research is important because it makes Federated Learning more accessible to a wider audience, allowing more people to take advantage of collaborative machine learning without needing deep technical knowledge. By simplifying the process, it could lead to better privacy in data handling and more effective machine learning applications across various fields.
Abstract
Federated Learning (FL) offers a promising approach for collaborative machine learning across distributed devices. However, its adoption is hindered by the complexity of building reliable communication architectures and the need for expertise in both machine learning and network programming. This paper presents a comprehensive solution that simplifies the orchestration of FL tasks while integrating intent-based automation. We develop a user-friendly web application supporting the federated averaging (FedAvg) algorithm, enabling users to configure parameters through an intuitive interface. The backend solution efficiently manages communication between the parameter server and edge nodes. We also implement model compression and scheduling algorithms to optimize FL performance. Furthermore, we explore intent-based automation in FL using a fine-tuned Language Model (LLM) trained on a tailored dataset, allowing users to conduct FL tasks using high-level prompts. We observe that the LLM-based automated solution achieves comparable test accuracy to the standard web-based solution while reducing transferred bytes by up to 64% and CPU time by up to 46% for FL tasks. Also, we leverage the neural architecture search (NAS) and hyperparameter optimization (HPO) using LLM to improve the performance. We observe that by using this approach test accuracy can be improved by 10-20% for the carried out FL tasks.