AAAR-1.0: Assessing AI's Potential to Assist Research
Renze Lou, Hanzi Xu, Sijia Wang, Jiangshu Du, Ryo Kamoi, Xiaoxin Lu, Jian Xie, Yuxuan Sun, Yusen Zhang, Jihyun Janice Ahn, Hongchao Fang, Zhuoyang Zou, Wenchao Ma, Xi Li, Kai Zhang, Congying Xia, Lifu Huang, Wenpeng Yin
2024-11-01
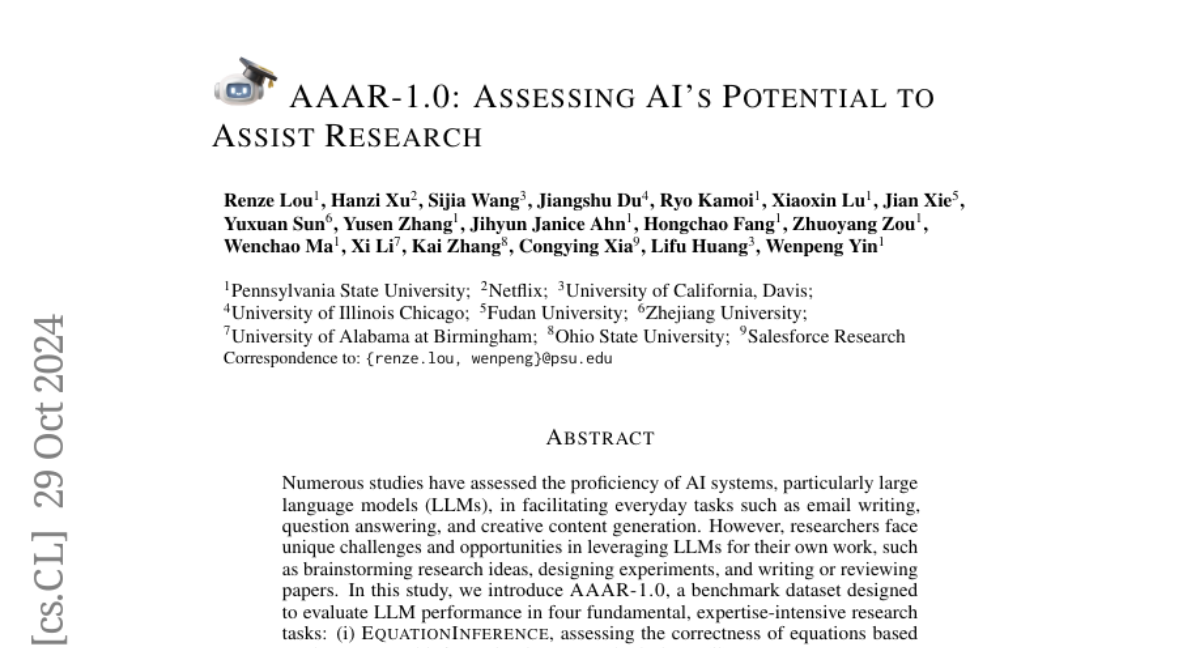
Summary
This paper introduces AAAR-1.0, a benchmark dataset designed to assess how well large language models (LLMs) can assist researchers in complex tasks like evaluating equations, designing experiments, and identifying weaknesses in research papers.
What's the problem?
While LLMs have shown they can help with everyday tasks like writing emails or answering questions, they struggle with more complex research tasks that require deep understanding and expertise. Existing training methods often focus on simpler tasks and do not effectively prepare LLMs for the nuanced work that researchers do.
What's the solution?
The authors developed AAAR-1.0, which includes specific tasks that reflect the real challenges researchers face. These tasks are: EquationInference (checking the correctness of equations), ExperimentDesign (creating experiments to test ideas), PaperWeakness (finding flaws in papers), and REVIEWCRITIQUE (evaluating reviews of papers). By using this benchmark, they evaluated various LLMs to see how well they performed these complex tasks, revealing both their strengths and limitations.
Why it matters?
This research is important because it provides a way to measure how effectively AI can support researchers in their work. By focusing on real-world research activities, AAAR-1.0 helps improve LLM training methods, making them more useful for academic and scientific purposes. This could lead to better tools for researchers, enhancing productivity and quality in scientific research.
Abstract
Numerous studies have assessed the proficiency of AI systems, particularly large language models (LLMs), in facilitating everyday tasks such as email writing, question answering, and creative content generation. However, researchers face unique challenges and opportunities in leveraging LLMs for their own work, such as brainstorming research ideas, designing experiments, and writing or reviewing papers. In this study, we introduce AAAR-1.0, a benchmark dataset designed to evaluate LLM performance in three fundamental, expertise-intensive research tasks: (i) EquationInference, assessing the correctness of equations based on the contextual information in paper submissions; (ii) ExperimentDesign, designing experiments to validate research ideas and solutions; (iii) PaperWeakness, identifying weaknesses in paper submissions; and (iv) REVIEWCRITIQUE, identifying each segment in human reviews is deficient or not. AAAR-1.0 differs from prior benchmarks in two key ways: first, it is explicitly research-oriented, with tasks requiring deep domain expertise; second, it is researcher-oriented, mirroring the primary activities that researchers engage in on a daily basis. An evaluation of both open-source and proprietary LLMs reveals their potential as well as limitations in conducting sophisticated research tasks. We will keep iterating AAAR-1.0 to new versions.