AceMath: Advancing Frontier Math Reasoning with Post-Training and Reward Modeling
Zihan Liu, Yang Chen, Mohammad Shoeybi, Bryan Catanzaro, Wei Ping
2024-12-20
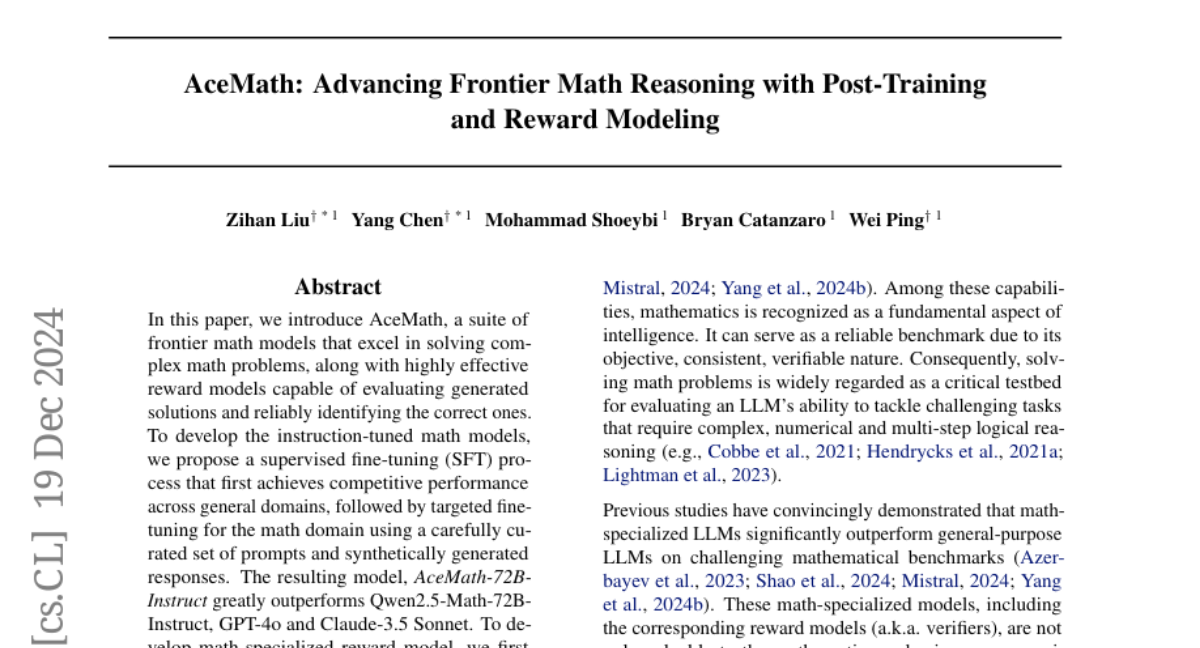
Summary
This paper introduces AceMath, a new set of advanced math models designed to solve complex math problems more effectively. It also includes reward models that help evaluate and identify correct solutions.
What's the problem?
Many existing AI models struggle with advanced math reasoning, which limits their ability to solve complex problems accurately. There is a need for better training methods and evaluation systems to improve these models' performance in mathematics.
What's the solution?
The authors developed AceMath by first creating a general model that performs well across various subjects and then fine-tuning it specifically for math using a curated set of prompts and responses. They also built a benchmark called AceMath-RewardBench to evaluate the performance of their reward models on different math problems. The resulting model, AceMath-72B-Instruct, significantly outperforms other leading models in solving math problems.
Why it matters?
This research is important because it advances the capabilities of AI in understanding and solving complex mathematical problems. By improving how AI models are trained and evaluated, AceMath can enhance applications in education, research, and any field where advanced math reasoning is essential.
Abstract
In this paper, we introduce AceMath, a suite of frontier math models that excel in solving complex math problems, along with highly effective reward models capable of evaluating generated solutions and reliably identifying the correct ones. To develop the instruction-tuned math models, we propose a supervised fine-tuning (SFT) process that first achieves competitive performance across general domains, followed by targeted fine-tuning for the math domain using a carefully curated set of prompts and synthetically generated responses. The resulting model, AceMath-72B-Instruct greatly outperforms Qwen2.5-Math-72B-Instruct, GPT-4o and Claude-3.5 Sonnet. To develop math-specialized reward model, we first construct AceMath-RewardBench, a comprehensive and robust benchmark for evaluating math reward models across diverse problems and difficulty levels. After that, we present a systematic approach to build our math reward models. The resulting model, AceMath-72B-RM, consistently outperforms state-of-the-art reward models. Furthermore, when combining AceMath-72B-Instruct with AceMath-72B-RM, we achieve the highest average rm@8 score across the math reasoning benchmarks. We will release model weights, training data, and evaluation benchmarks at: https://research.nvidia.com/labs/adlr/acemath