Adapting General Disentanglement-Based Speaker Anonymization for Enhanced Emotion Preservation
Xiaoxiao Miao, Yuxiang Zhang, Xin Wang, Natalia Tomashenko, Donny Cheng Lock Soh, Ian Mcloughlin
2024-08-14
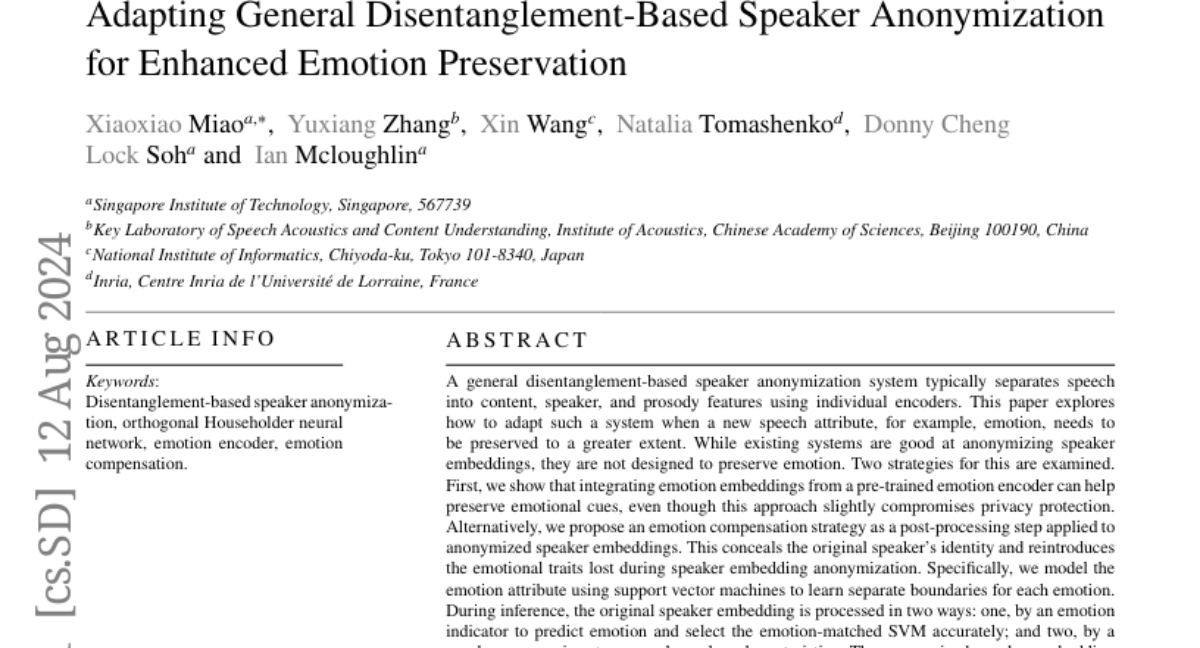
Summary
This paper discusses how to improve speaker anonymization systems so that they can better preserve emotions while still hiding the speaker's identity.
What's the problem?
Current systems that anonymize speakers in audio recordings do a good job of hiding who is speaking, but they often lose important emotional cues in the process. This means that while the speaker's identity is protected, the emotional tone of their speech can be lost, which is important for understanding the context and meaning.
What's the solution?
The authors propose two strategies to address this issue. The first strategy involves using an emotion encoder to add emotional information back into the anonymized speech, although this can slightly reduce privacy. The second strategy, called emotion compensation, adjusts the anonymized speech to restore emotional traits that were lost during the anonymization process. This involves using machine learning techniques to predict emotions and modify the speech accordingly, ensuring that the emotional content is preserved without revealing the speaker's identity.
Why it matters?
This research is significant because it helps create better audio anonymization systems that maintain both privacy and emotional richness in speech. This is particularly important in fields like healthcare or law enforcement, where understanding emotions can be crucial while still needing to protect individuals' identities.
Abstract
A general disentanglement-based speaker anonymization system typically separates speech into content, speaker, and prosody features using individual encoders. This paper explores how to adapt such a system when a new speech attribute, for example, emotion, needs to be preserved to a greater extent. While existing systems are good at anonymizing speaker embeddings, they are not designed to preserve emotion. Two strategies for this are examined. First, we show that integrating emotion embeddings from a pre-trained emotion encoder can help preserve emotional cues, even though this approach slightly compromises privacy protection. Alternatively, we propose an emotion compensation strategy as a post-processing step applied to anonymized speaker embeddings. This conceals the original speaker's identity and reintroduces the emotional traits lost during speaker embedding anonymization. Specifically, we model the emotion attribute using support vector machines to learn separate boundaries for each emotion. During inference, the original speaker embedding is processed in two ways: one, by an emotion indicator to predict emotion and select the emotion-matched SVM accurately; and two, by a speaker anonymizer to conceal speaker characteristics. The anonymized speaker embedding is then modified along the corresponding SVM boundary towards an enhanced emotional direction to save the emotional cues. The proposed strategies are also expected to be useful for adapting a general disentanglement-based speaker anonymization system to preserve other target paralinguistic attributes, with potential for a range of downstream tasks.