Adapting While Learning: Grounding LLMs for Scientific Problems with Intelligent Tool Usage Adaptation
Bohan Lyu, Yadi Cao, Duncan Watson-Parris, Leon Bergen, Taylor Berg-Kirkpatrick, Rose Yu
2024-11-04
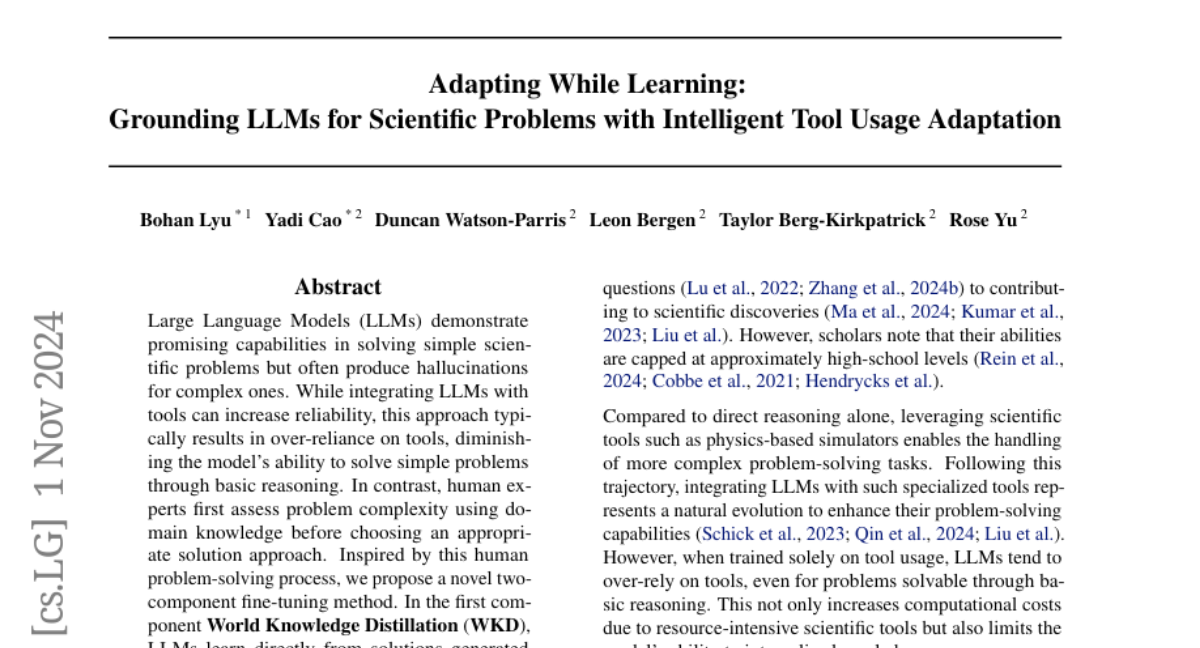
Summary
This paper discusses a new method for improving how Large Language Models (LLMs) solve scientific problems by adapting their use of tools based on the complexity of the task. It proposes a two-part training approach that helps LLMs decide when to use external tools and when to rely on their own reasoning.
What's the problem?
While LLMs can handle simple scientific problems well, they often struggle with more complex ones, sometimes producing incorrect answers or 'hallucinations.' When LLMs rely too much on tools for problem-solving, they can lose their ability to solve simpler problems independently, which is not how human experts typically work. Humans first assess how difficult a problem is before deciding whether to use a tool or solve it through reasoning.
What's the solution?
The authors propose a two-component fine-tuning method. The first part, called World Knowledge Distillation (WKD), allows LLMs to learn from accurate solutions generated using tools. The second part, Tool Usage Adaptation (TUA), helps the model categorize problems into easy or hard based on its ability to answer them correctly. For easier problems, the model continues to learn from WKD, while for harder problems, it learns when to switch to using tools. This method was tested on various scientific datasets and showed significant improvements in accuracy and tool usage.
Why it matters?
This research is important because it enhances the reliability and effectiveness of LLMs in solving scientific problems. By mimicking how human experts approach problem-solving, this method allows LLMs to become more versatile and accurate, making them better assistants in fields like mathematics, climate science, and epidemiology. The findings could lead to more robust AI systems that can tackle complex real-world challenges.
Abstract
Large Language Models (LLMs) demonstrate promising capabilities in solving simple scientific problems but often produce hallucinations for complex ones. While integrating LLMs with tools can increase reliability, this approach typically results in over-reliance on tools, diminishing the model's ability to solve simple problems through basic reasoning. In contrast, human experts first assess problem complexity using domain knowledge before choosing an appropriate solution approach. Inspired by this human problem-solving process, we propose a novel two-component fine-tuning method. In the first component World Knowledge Distillation (WKD), LLMs learn directly from solutions generated using tool's information to internalize domain knowledge. In the second component Tool Usage Adaptation (TUA), we partition problems into easy and hard categories based on the model's direct answering accuracy. While maintaining the same alignment target for easy problems as in WKD, we train the model to intelligently switch to tool usage for more challenging problems. We validate our method on six scientific benchmark datasets, spanning mathematics, climate science and epidemiology. On average, our models demonstrate a 28.18% improvement in answer accuracy and a 13.89% increase in tool usage precision across all datasets, surpassing state-of-the-art models including GPT-4o and Claude-3.5.