Advancing Molecular Machine (Learned) Representations with Stereoelectronics-Infused Molecular Graphs
Daniil A. Boiko, Thiago Reschützegger, Benjamin Sanchez-Lengeling, Samuel M. Blau, Gabe Gomes
2024-08-09
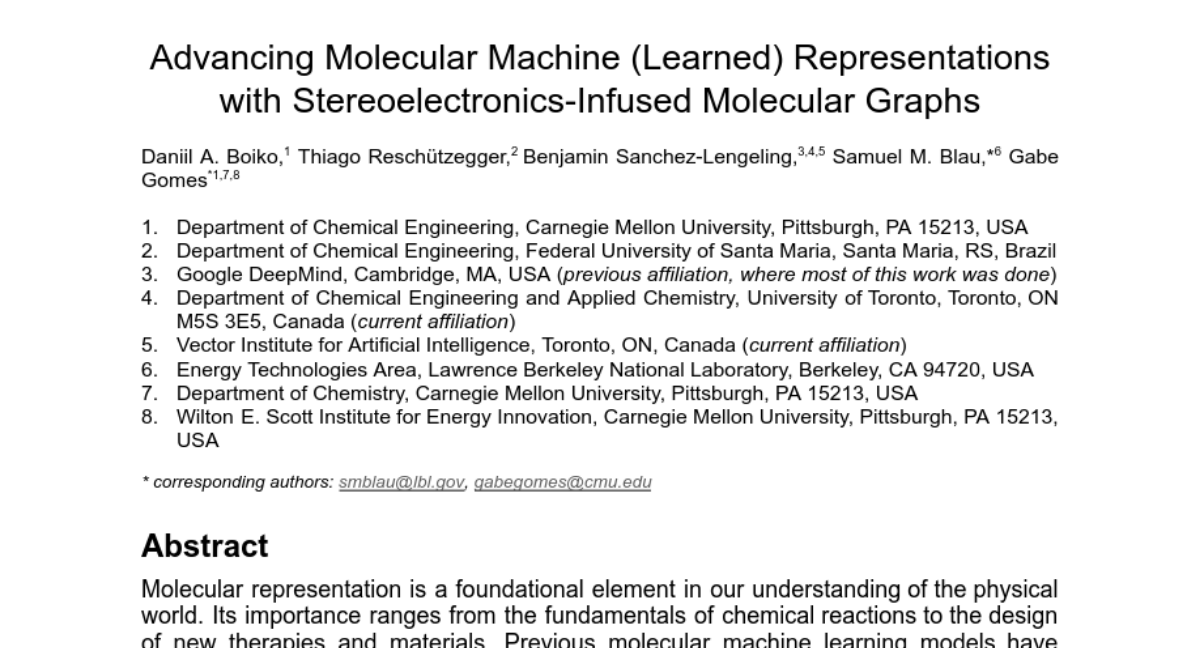
Summary
This paper discusses a new approach to improving how we represent and understand molecules by incorporating stereoelectronic effects into molecular graphs, which are used in machine learning models.
What's the problem?
Understanding molecules is crucial for many fields, including chemistry and medicine. However, traditional methods of representing molecules often lack detailed information, making it hard for machine learning models to accurately predict chemical behaviors or design new materials. These simple representations can lead to less effective models that struggle with complex tasks.
What's the solution?
The authors propose a method that enhances molecular graphs by adding information about stereoelectronic interactions, which are the effects of the spatial arrangement of electrons in molecules. They developed a new way to create these enriched molecular representations using a double graph neural network, allowing the models to learn from this detailed data. Their approach shows that including stereoelectronic effects significantly improves the performance of machine learning models in predicting molecular properties and behaviors.
Why it matters?
This research is important because it provides a more accurate way to represent molecules, which can lead to better predictions in drug discovery, material science, and other applications. By improving how we model molecular interactions, this work can help scientists design more effective therapies and materials, ultimately advancing our understanding of chemistry and its practical applications.
Abstract
Molecular representation is a foundational element in our understanding of the physical world. Its importance ranges from the fundamentals of chemical reactions to the design of new therapies and materials. Previous molecular machine learning models have employed strings, fingerprints, global features, and simple molecular graphs that are inherently information-sparse representations. However, as the complexity of prediction tasks increases, the molecular representation needs to encode higher fidelity information. This work introduces a novel approach to infusing quantum-chemical-rich information into molecular graphs via stereoelectronic effects. We show that the explicit addition of stereoelectronic interactions significantly improves the performance of molecular machine learning models. Furthermore, stereoelectronics-infused representations can be learned and deployed with a tailored double graph neural network workflow, enabling its application to any downstream molecular machine learning task. Finally, we show that the learned representations allow for facile stereoelectronic evaluation of previously intractable systems, such as entire proteins, opening new avenues of molecular design.