AIM 2024 Sparse Neural Rendering Challenge: Dataset and Benchmark
Michal Nazarczuk, Thomas Tanay, Sibi Catley-Chandar, Richard Shaw, Radu Timofte, Eduardo Pérez-Pellitero
2024-09-26
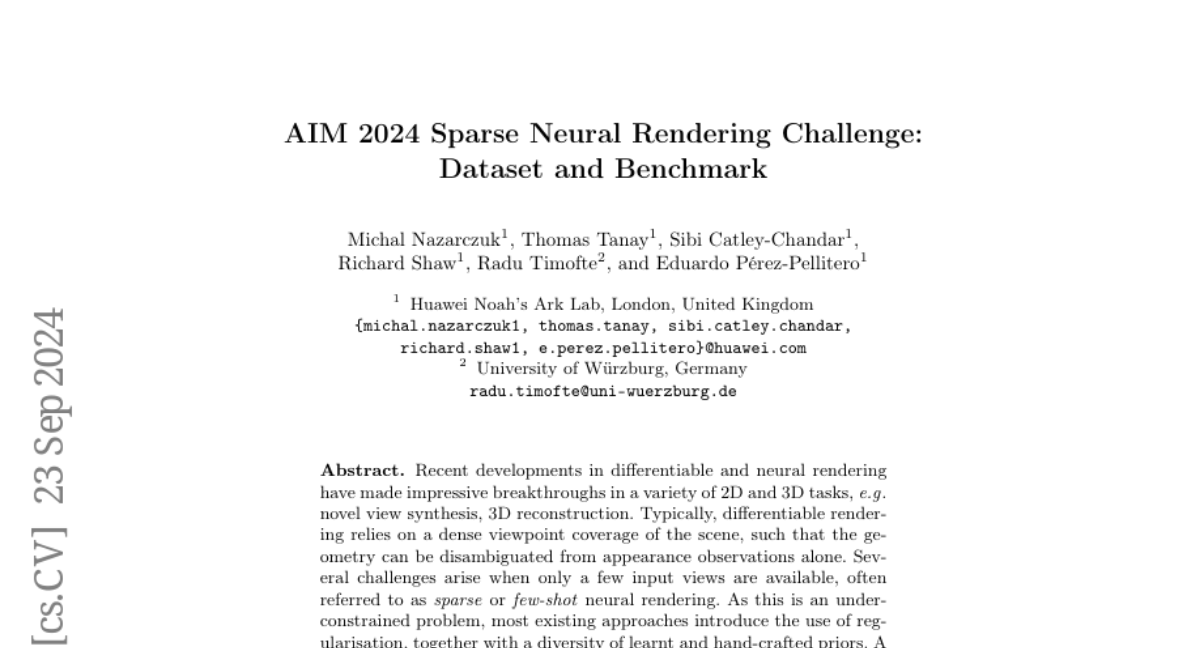
Summary
This paper presents the AIM 2024 Sparse Neural Rendering Challenge, which focuses on improving techniques for rendering 3D scenes using only a few images. It introduces a new dataset and benchmark to help researchers develop better methods for creating high-quality 3D visuals from limited data.
What's the problem?
Rendering 3D scenes typically requires many images to create detailed and accurate representations. However, when only a few images are available, it becomes challenging to generate high-quality results. Existing methods often rely on low-resolution images and inconsistent evaluation standards, making it difficult to compare different approaches effectively.
What's the solution?
To address these issues, the researchers created the Sparse Rendering (SpaRe) dataset, which includes 97 new scenes with high-quality assets and multiple camera views. They set up a benchmark that allows researchers to test their methods against this dataset using two configurations: one with 3 input images and another with 9. This setup provides a standardized way to evaluate how well different models perform in generating 3D visuals from sparse data.
Why it matters?
This research is important because it helps advance the field of 3D rendering by providing a clear framework for testing new methods. By focusing on sparse neural rendering, the challenge encourages innovation in creating high-quality visuals with less data, which can lead to more efficient rendering techniques in various applications, such as video games, virtual reality, and simulations.
Abstract
Recent developments in differentiable and neural rendering have made impressive breakthroughs in a variety of 2D and 3D tasks, e.g. novel view synthesis, 3D reconstruction. Typically, differentiable rendering relies on a dense viewpoint coverage of the scene, such that the geometry can be disambiguated from appearance observations alone. Several challenges arise when only a few input views are available, often referred to as sparse or few-shot neural rendering. As this is an underconstrained problem, most existing approaches introduce the use of regularisation, together with a diversity of learnt and hand-crafted priors. A recurring problem in sparse rendering literature is the lack of an homogeneous, up-to-date, dataset and evaluation protocol. While high-resolution datasets are standard in dense reconstruction literature, sparse rendering methods often evaluate with low-resolution images. Additionally, data splits are inconsistent across different manuscripts, and testing ground-truth images are often publicly available, which may lead to over-fitting. In this work, we propose the Sparse Rendering (SpaRe) dataset and benchmark. We introduce a new dataset that follows the setup of the DTU MVS dataset. The dataset is composed of 97 new scenes based on synthetic, high-quality assets. Each scene has up to 64 camera views and 7 lighting configurations, rendered at 1600x1200 resolution. We release a training split of 82 scenes to foster generalizable approaches, and provide an online evaluation platform for the validation and test sets, whose ground-truth images remain hidden. We propose two different sparse configurations (3 and 9 input images respectively). This provides a powerful and convenient tool for reproducible evaluation, and enable researchers easy access to a public leaderboard with the state-of-the-art performance scores. Available at: https://sparebenchmark.github.io/