Aligning Teacher with Student Preferences for Tailored Training Data Generation
Yantao Liu, Zhao Zhang, Zijun Yao, Shulin Cao, Lei Hou, Juanzi Li
2024-06-28
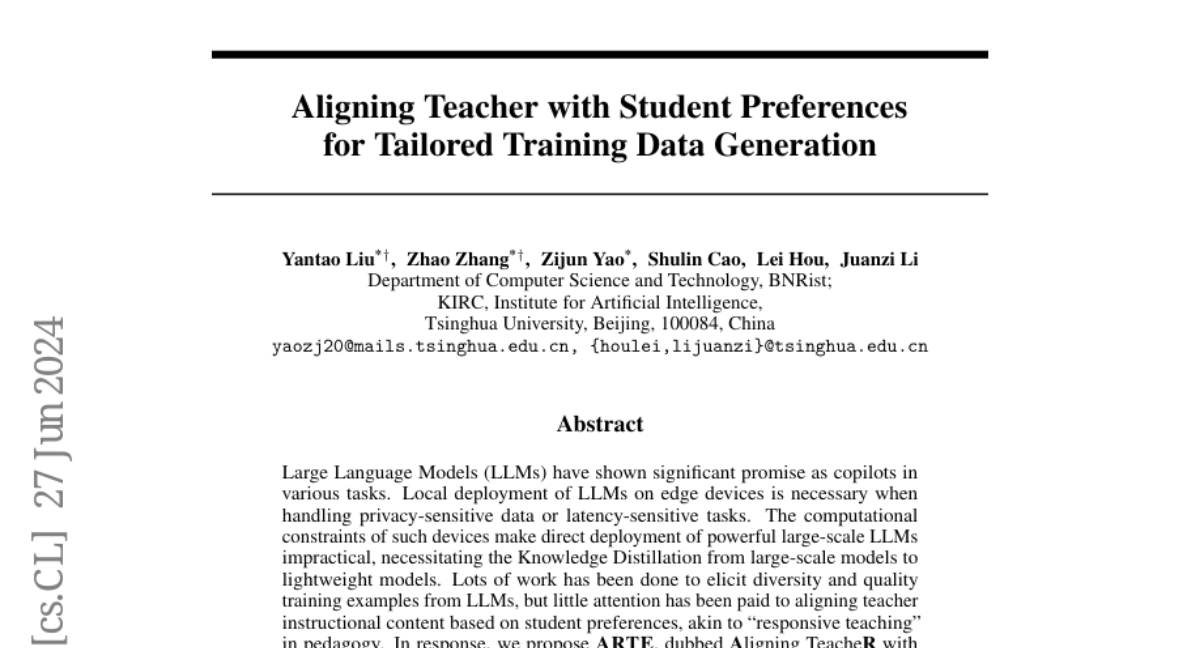
Summary
This paper talks about ARTE, a new framework designed to improve how large language models (LLMs) generate training data by aligning the content created by a 'teacher' model with the preferences of 'students.' This approach aims to make the training process more effective and personalized.
What's the problem?
When using LLMs for tasks, especially on devices with limited processing power, it's important to create smaller, more efficient models that can still perform well. However, most existing methods for generating training examples focus on diversity and quality without considering what students actually prefer or need. This lack of alignment can lead to less effective training and learning experiences.
What's the solution?
To address this issue, the authors developed ARTE (Aligning TeacheR with StudenT PreferencEs). This framework works by first having a teacher model generate draft questions and explanations. Then, it collects feedback from students based on their performance in learning tasks to understand their preferences. The teacher model is then adjusted to better align with these preferences, allowing it to produce tailored training examples that are more relevant to the students' needs. The process is repeated to ensure that the generated examples are as effective as possible for teaching.
Why it matters?
This research is important because it enhances the way training data is generated for LLMs, making it more responsive to student needs. By aligning the instructional content with student preferences, ARTE can improve learning outcomes and help create more effective educational tools. This approach not only benefits individual learners but also contributes to the development of more capable and adaptable AI systems in education.
Abstract
Large Language Models (LLMs) have shown significant promise as copilots in various tasks. Local deployment of LLMs on edge devices is necessary when handling privacy-sensitive data or latency-sensitive tasks. The computational constraints of such devices make direct deployment of powerful large-scale LLMs impractical, necessitating the Knowledge Distillation from large-scale models to lightweight models. Lots of work has been done to elicit diversity and quality training examples from LLMs, but little attention has been paid to aligning teacher instructional content based on student preferences, akin to "responsive teaching" in pedagogy. Thus, we propose ARTE, dubbed Aligning TeacheR with StudenT PreferencEs, a framework that aligns the teacher model with student preferences to generate tailored training examples for Knowledge Distillation. Specifically, we elicit draft questions and rationales from the teacher model, then collect student preferences on these questions and rationales using students' performance with in-context learning as a proxy, and finally align the teacher model with student preferences. In the end, we repeat the first step with the aligned teacher model to elicit tailored training examples for the student model on the target task. Extensive experiments on academic benchmarks demonstrate the superiority of ARTE over existing instruction-tuning datasets distilled from powerful LLMs. Moreover, we thoroughly investigate the generalization of ARTE, including the generalization of fine-tuned student models in reasoning ability and the generalization of aligned teacher models to generate tailored training data across tasks and students. In summary, our contributions lie in proposing a novel framework for tailored training example generation, demonstrating its efficacy in experiments, and investigating the generalization of both student & aligned teacher models in ARTE.