AMEX: Android Multi-annotation Expo Dataset for Mobile GUI Agents
Yuxiang Chai, Siyuan Huang, Yazhe Niu, Han Xiao, Liang Liu, Dingyu Zhang, Peng Gao, Shuai Ren, Hongsheng Li
2024-07-26
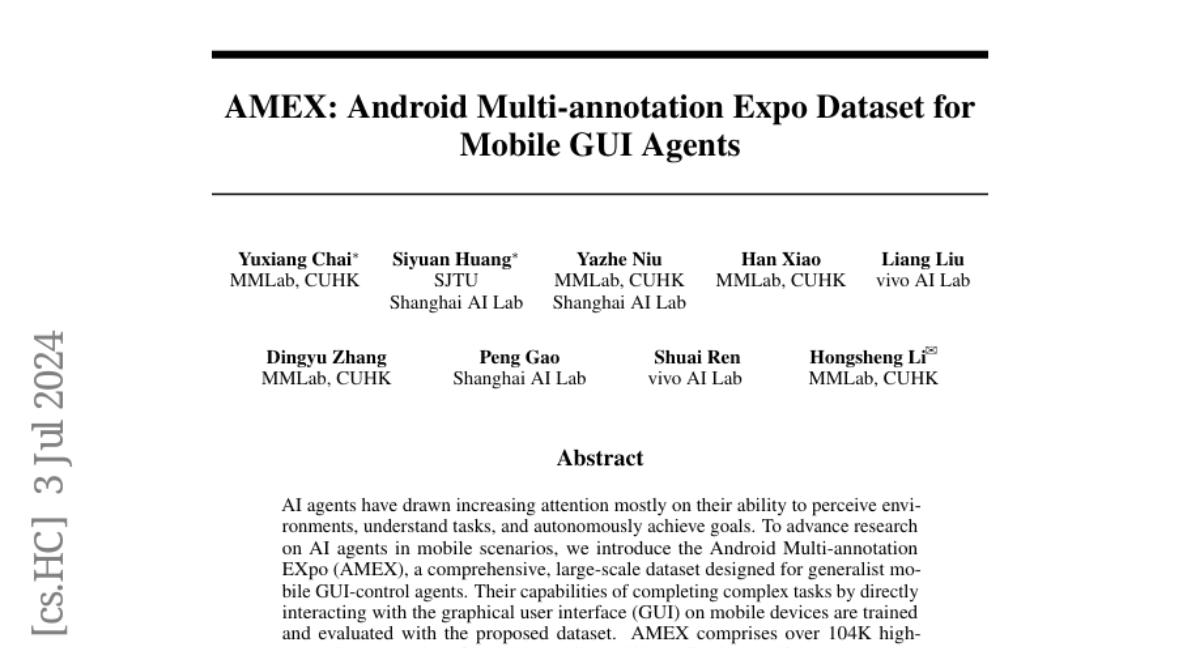
Summary
This paper introduces AMEX, a large dataset designed to help AI agents learn how to interact with mobile app interfaces. It includes thousands of annotated screenshots from popular Android apps to improve the way AI understands and controls graphical user interfaces (GUIs).
What's the problem?
AI agents need to be able to understand and interact with mobile apps effectively, but existing datasets often lack the detailed information needed for training. Many datasets do not provide enough context or instructions for how to perform tasks within these apps, making it hard for AI to learn how to navigate and use them properly.
What's the solution?
The researchers created AMEX, which contains over 104,000 high-quality screenshots from 110 popular Android applications. Each screenshot is annotated at three levels: identifying interactive elements (like buttons and text fields), describing what those elements do, and providing complex natural language instructions that explain how to use them. This detailed structure helps train AI agents to perform tasks by directly interacting with the app interfaces. Additionally, they developed a baseline model called SPHINX Agent to test the effectiveness of this dataset against other existing datasets.
Why it matters?
AMEX is important because it provides a rich resource for researchers working on AI agents that can control mobile applications. By offering detailed annotations and real-world examples, it helps improve the capabilities of AI in understanding and interacting with GUIs, which can lead to better virtual assistants and more efficient automated systems in mobile technology.
Abstract
AI agents have drawn increasing attention mostly on their ability to perceive environments, understand tasks, and autonomously achieve goals. To advance research on AI agents in mobile scenarios, we introduce the Android Multi-annotation EXpo (AMEX), a comprehensive, large-scale dataset designed for generalist mobile GUI-control agents. Their capabilities of completing complex tasks by directly interacting with the graphical user interface (GUI) on mobile devices are trained and evaluated with the proposed dataset. AMEX comprises over 104K high-resolution screenshots from 110 popular mobile applications, which are annotated at multiple levels. Unlike existing mobile device-control datasets, e.g., MoTIF, AitW, etc., AMEX includes three levels of annotations: GUI interactive element grounding, GUI screen and element functionality descriptions, and complex natural language instructions, each averaging 13 steps with stepwise GUI-action chains. We develop this dataset from a more instructive and detailed perspective, complementing the general settings of existing datasets. Additionally, we develop a baseline model SPHINX Agent and compare its performance across state-of-the-art agents trained on other datasets. To facilitate further research, we open-source our dataset, models, and relevant evaluation tools. The project is available at https://yuxiangchai.github.io/AMEX/