An Object is Worth 64x64 Pixels: Generating 3D Object via Image Diffusion
Xingguang Yan, Han-Hung Lee, Ziyu Wan, Angel X. Chang
2024-08-07
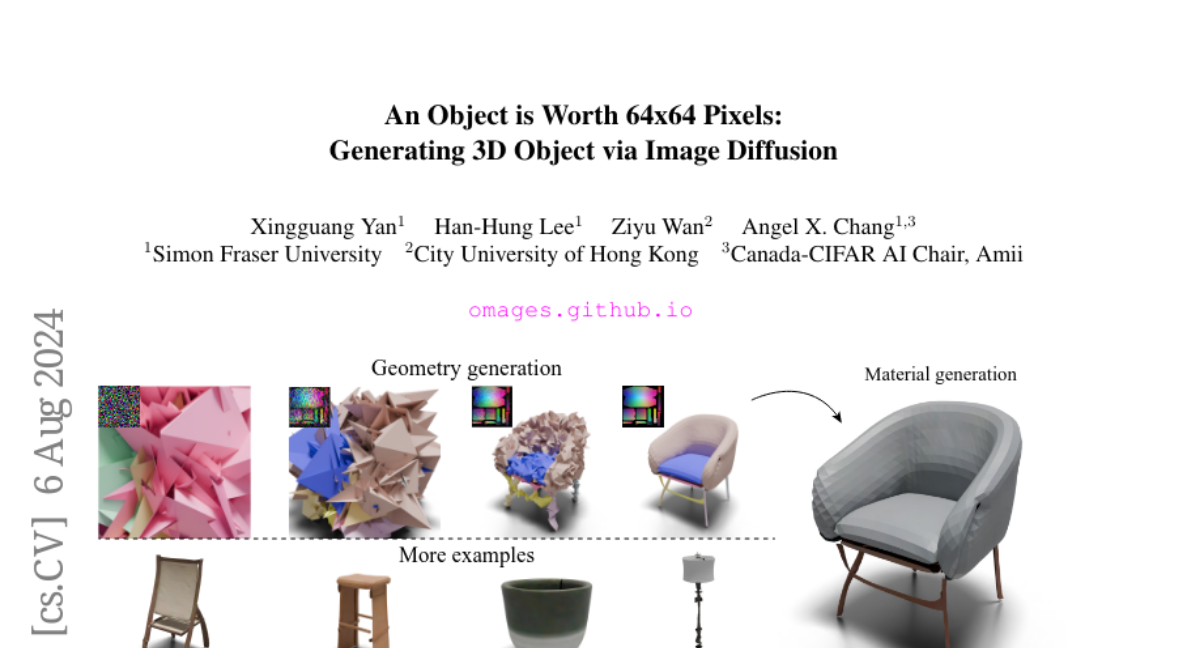
Summary
This paper discusses a new method for creating realistic 3D models from images using a technique called 'Object Images,' which simplifies complex 3D shapes into manageable 2D representations.
What's the problem?
Generating 3D models from images is challenging because traditional methods often struggle with the irregular shapes and complex details of real objects. Many existing models treat 3D shapes like simple statues, which makes them hard to edit or animate and doesn't capture the intricate details of actual objects.
What's the solution?
The authors propose a new approach that represents 3D objects as 64x64 pixel images, called 'Object Images' or 'omages.' This method encodes the shape, appearance, and materials of an object in a way that allows advanced image generation models to create realistic 3D shapes. By using image diffusion techniques, they can generate these low-resolution images that later reveal detailed structures during the process of refining the image. The results show that this approach can produce high-quality models comparable to existing advanced 3D generation methods.
Why it matters?
This research is important because it makes it easier to create detailed and realistic 3D models from images. By simplifying the process and improving how models can understand and generate complex shapes, this method can benefit various fields, such as gaming, animation, and virtual reality, where high-quality 3D assets are essential.
Abstract
We introduce a new approach for generating realistic 3D models with UV maps through a representation termed "Object Images." This approach encapsulates surface geometry, appearance, and patch structures within a 64x64 pixel image, effectively converting complex 3D shapes into a more manageable 2D format. By doing so, we address the challenges of both geometric and semantic irregularity inherent in polygonal meshes. This method allows us to use image generation models, such as Diffusion Transformers, directly for 3D shape generation. Evaluated on the ABO dataset, our generated shapes with patch structures achieve point cloud FID comparable to recent 3D generative models, while naturally supporting PBR material generation.