Animate3D: Animating Any 3D Model with Multi-view Video Diffusion
Yanqin Jiang, Chaohui Yu, Chenjie Cao, Fan Wang, Weiming Hu, Jin Gao
2024-07-17
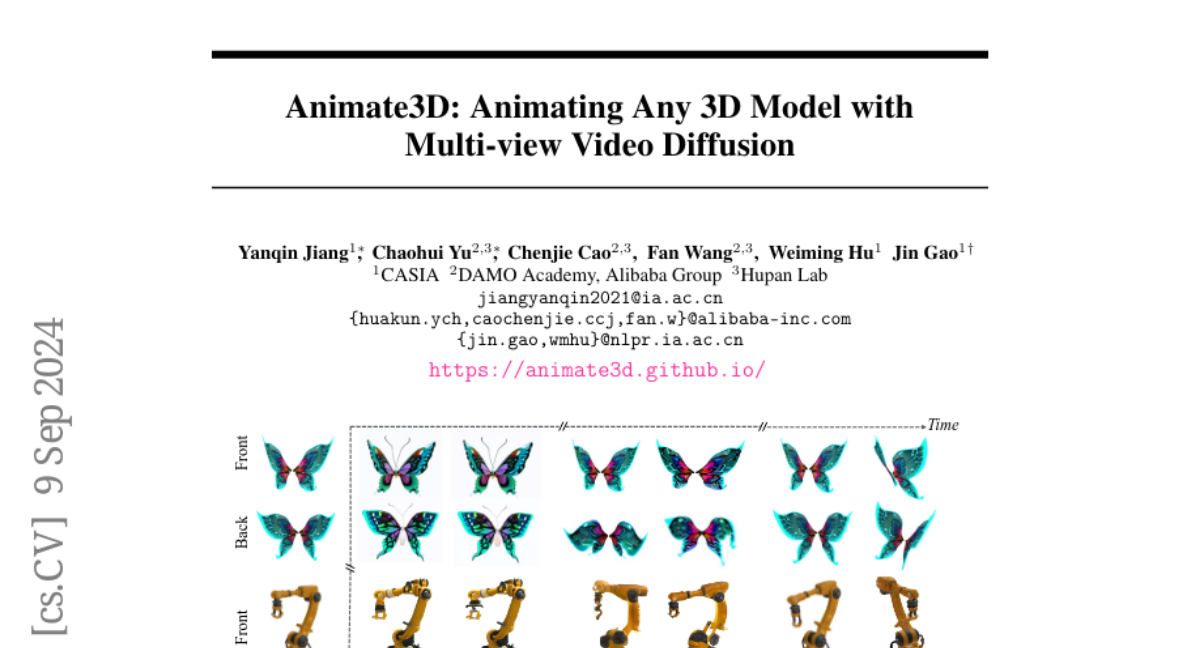
Summary
This paper introduces Animate3D, a new framework that allows users to animate any static 3D model using advanced techniques that combine video and 3D rendering.
What's the problem?
Most existing methods for animating 3D models struggle with consistency and quality, especially when using single images or text prompts. These methods often cannot effectively utilize the rich details available from multiple views of a model, leading to animations that don't look realistic or coherent over time.
What's the solution?
Animate3D addresses these challenges by using a multi-view video diffusion model (MV-VDM) that takes advantage of multiple angles of a static 3D object. The framework is built on a large dataset of multi-view videos, allowing it to create smoother and more realistic animations. It uses a two-step process: first, it reconstructs motion from generated videos, and then it refines the animation using a technique called 4D Score Distillation Sampling (4D-SDS). This ensures that both the movement and appearance of the animated model are high quality and consistent.
Why it matters?
This research is important because it provides an innovative way to animate 3D models that can be used in various fields like gaming, film, and virtual reality. By improving the quality and efficiency of animations, Animate3D can help creators produce more engaging and lifelike characters and scenes, enhancing the overall experience for audiences.
Abstract
Recent advances in 4D generation mainly focus on generating 4D content by distilling pre-trained text or single-view image-conditioned models. It is inconvenient for them to take advantage of various off-the-shelf 3D assets with multi-view attributes, and their results suffer from spatiotemporal inconsistency owing to the inherent ambiguity in the supervision signals. In this work, we present Animate3D, a novel framework for animating any static 3D model. The core idea is two-fold: 1) We propose a novel multi-view video diffusion model (MV-VDM) conditioned on multi-view renderings of the static 3D object, which is trained on our presented large-scale multi-view video dataset (MV-Video). 2) Based on MV-VDM, we introduce a framework combining reconstruction and 4D Score Distillation Sampling (4D-SDS) to leverage the multi-view video diffusion priors for animating 3D objects. Specifically, for MV-VDM, we design a new spatiotemporal attention module to enhance spatial and temporal consistency by integrating 3D and video diffusion models. Additionally, we leverage the static 3D model's multi-view renderings as conditions to preserve its identity. For animating 3D models, an effective two-stage pipeline is proposed: we first reconstruct motions directly from generated multi-view videos, followed by the introduced 4D-SDS to refine both appearance and motion. Qualitative and quantitative experiments demonstrate that Animate3D significantly outperforms previous approaches. Data, code, and models will be open-released.