AnySat: An Earth Observation Model for Any Resolutions, Scales, and Modalities
Guillaume Astruc, Nicolas Gonthier, Clement Mallet, Loic Landrieu
2024-12-19
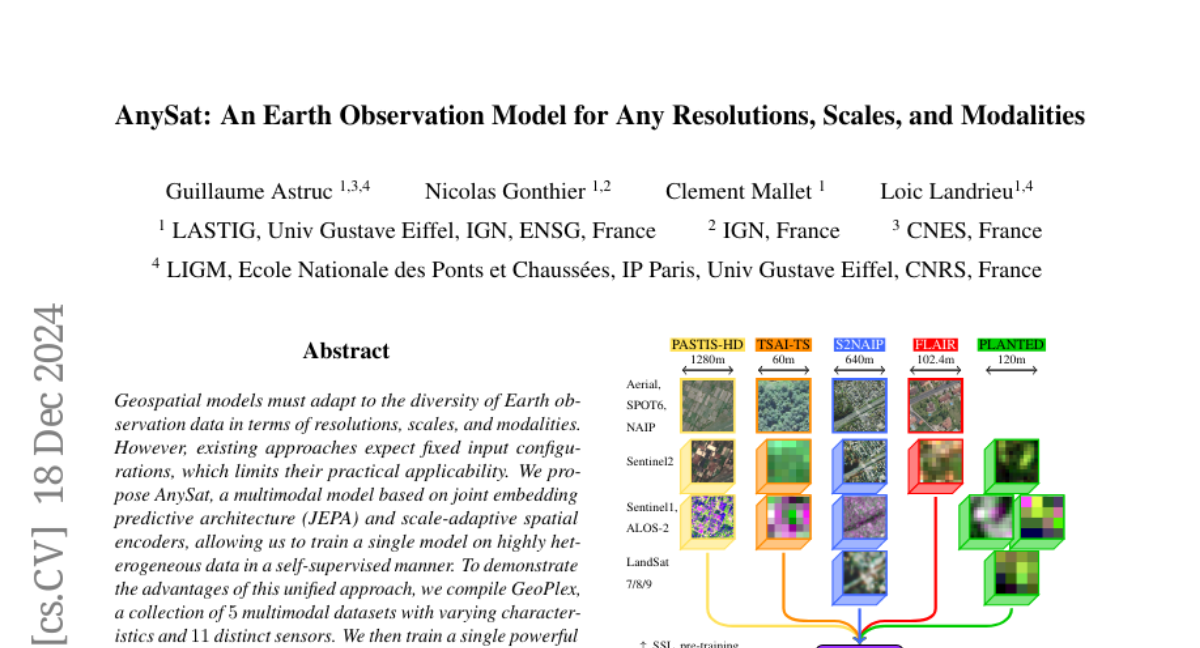
Summary
This paper talks about AnySat, a new model designed for analyzing Earth observation data that can adapt to different resolutions, scales, and types of data all at once.
What's the problem?
Current models for analyzing Earth observation data often require fixed input settings, which limits their ability to work with the diverse types of data collected from satellites and other sources. This makes it difficult to apply these models effectively in real-world situations where data can vary widely in quality and format.
What's the solution?
The authors introduce AnySat, a multimodal model that uses a special architecture called joint embedding predictive architecture (JEPA) along with resolution-adaptive spatial encoders. This allows AnySat to train on a wide range of data types without needing to change its structure. They created a dataset called GeoPlex, which includes five different types of data from 11 sensors, and trained AnySat on this diverse set. After fine-tuning, the model showed improved performance on various tasks related to monitoring the environment, such as mapping land use and identifying tree species.
Why it matters?
This research is important because it enhances our ability to monitor and understand the Earth's environment using advanced technology. By allowing a single model to handle different types of data effectively, AnySat can lead to better insights in fields like agriculture, forestry, and disaster management, ultimately helping us make more informed decisions about our planet.
Abstract
Geospatial models must adapt to the diversity of Earth observation data in terms of resolutions, scales, and modalities. However, existing approaches expect fixed input configurations, which limits their practical applicability. We propose AnySat, a multimodal model based on joint embedding predictive architecture (JEPA) and resolution-adaptive spatial encoders, allowing us to train a single model on highly heterogeneous data in a self-supervised manner. To demonstrate the advantages of this unified approach, we compile GeoPlex, a collection of 5 multimodal datasets with varying characteristics and 11 distinct sensors. We then train a single powerful model on these diverse datasets simultaneously. Once fine-tuned, we achieve better or near state-of-the-art results on the datasets of GeoPlex and 4 additional ones for 5 environment monitoring tasks: land cover mapping, tree species identification, crop type classification, change detection, and flood segmentation. The code and models are available at https://github.com/gastruc/AnySat.