Apollo: An Exploration of Video Understanding in Large Multimodal Models
Orr Zohar, Xiaohan Wang, Yann Dubois, Nikhil Mehta, Tong Xiao, Philippe Hansen-Estruch, Licheng Yu, Xiaofang Wang, Felix Juefei-Xu, Ning Zhang, Serena Yeung-Levy, Xide Xia
2024-12-16
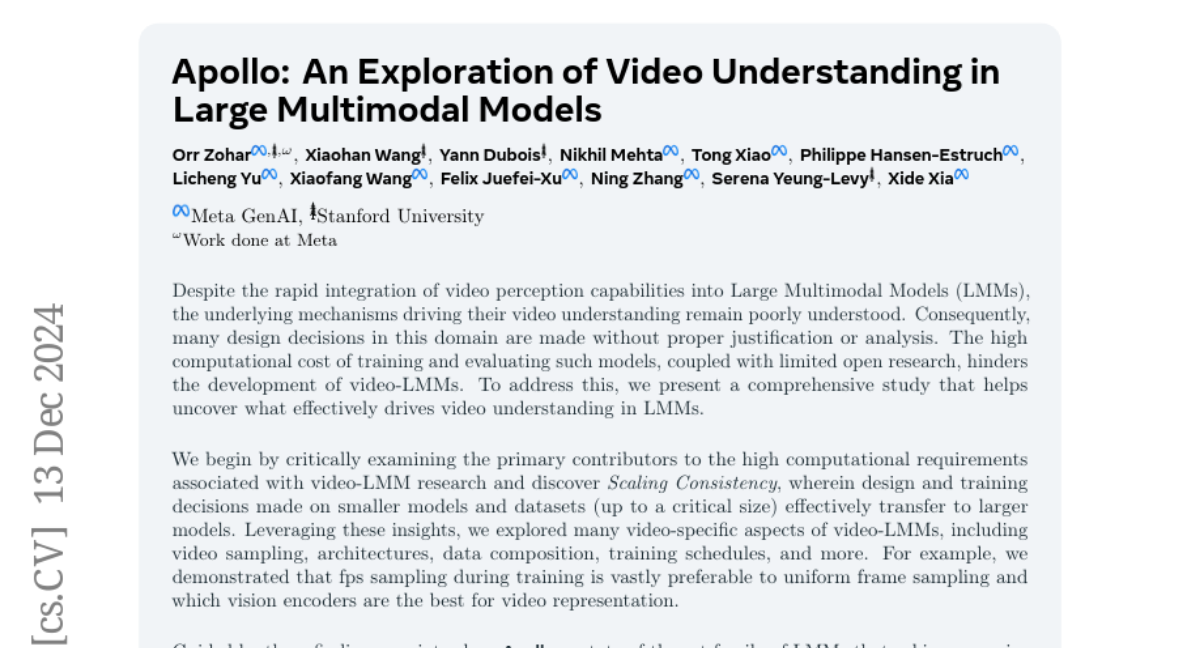
Summary
This paper talks about Apollo, a new family of models designed to help computers understand videos better by using advanced techniques that streamline the learning process.
What's the problem?
Even though AI has made progress in understanding videos, many existing models struggle with the high computational costs and complexities involved in processing video data. This makes it difficult to develop effective video understanding systems, and previous methods often lack clear reasoning behind their design choices.
What's the solution?
Apollo addresses these challenges by introducing a new framework that simplifies video understanding. It leverages insights from smaller models to make decisions about larger ones, a concept called Scaling Consistency. The researchers explored various aspects of video processing, such as how to sample frames efficiently and which model architectures work best for video representation. As a result, Apollo can analyze long videos effectively while maintaining high performance across different tasks.
Why it matters?
This research is significant because it sets a new standard for how AI can understand videos. By improving efficiency and performance, Apollo opens up new possibilities for applications in areas like video analysis, content creation, and interactive media. It also makes it easier for other researchers to build on this work without needing massive computing resources.
Abstract
Despite the rapid integration of video perception capabilities into Large Multimodal Models (LMMs), the underlying mechanisms driving their video understanding remain poorly understood. Consequently, many design decisions in this domain are made without proper justification or analysis. The high computational cost of training and evaluating such models, coupled with limited open research, hinders the development of video-LMMs. To address this, we present a comprehensive study that helps uncover what effectively drives video understanding in LMMs. We begin by critically examining the primary contributors to the high computational requirements associated with video-LMM research and discover Scaling Consistency, wherein design and training decisions made on smaller models and datasets (up to a critical size) effectively transfer to larger models. Leveraging these insights, we explored many video-specific aspects of video-LMMs, including video sampling, architectures, data composition, training schedules, and more. For example, we demonstrated that fps sampling during training is vastly preferable to uniform frame sampling and which vision encoders are the best for video representation. Guided by these findings, we introduce Apollo, a state-of-the-art family of LMMs that achieve superior performance across different model sizes. Our models can perceive hour-long videos efficiently, with Apollo-3B outperforming most existing 7B models with an impressive 55.1 on LongVideoBench. Apollo-7B is state-of-the-art compared to 7B LMMs with a 70.9 on MLVU, and 63.3 on Video-MME.