Apollo: Band-sequence Modeling for High-Quality Audio Restoration
Kai Li, Yi Luo
2024-09-16
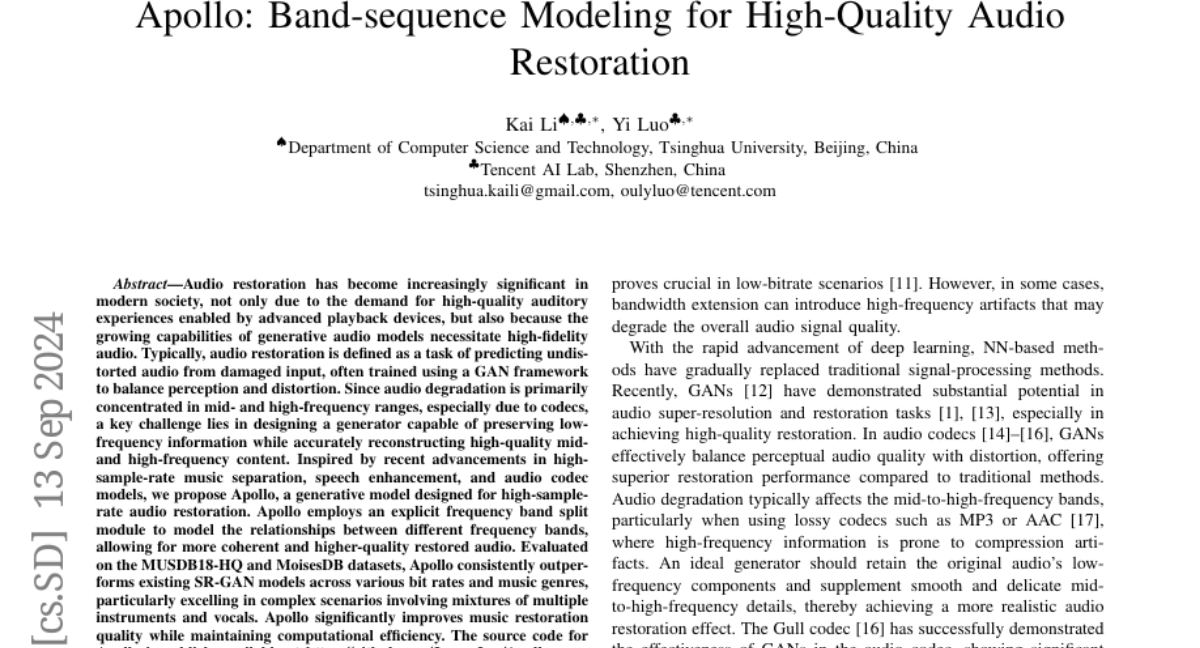
Summary
This paper introduces Apollo, a new method for restoring high-quality audio from damaged or compressed sources, making it sound clearer and more enjoyable.
What's the problem?
Audio restoration is important because people want high-quality sound, especially with advanced playback devices. However, existing methods struggle to fix audio that has been distorted by compression, particularly in the mid and high-frequency ranges where most issues occur. This makes it hard to get back the original sound quality.
What's the solution?
Apollo uses a special technique that separates audio into different frequency bands to better restore sound quality. It employs a frequency band split module that helps the model understand how different parts of the audio relate to each other. This allows Apollo to effectively restore both low and high-frequency sounds while maintaining clarity. The model was tested on various music datasets and consistently performed better than previous methods.
Why it matters?
This research matters because it significantly improves how we can repair and enhance audio quality, which is essential for music lovers, filmmakers, and anyone who relies on clear sound. By making audio restoration more effective, Apollo can help deliver a better listening experience in music streaming, movies, and other media.
Abstract
Audio restoration has become increasingly significant in modern society, not only due to the demand for high-quality auditory experiences enabled by advanced playback devices, but also because the growing capabilities of generative audio models necessitate high-fidelity audio. Typically, audio restoration is defined as a task of predicting undistorted audio from damaged input, often trained using a GAN framework to balance perception and distortion. Since audio degradation is primarily concentrated in mid- and high-frequency ranges, especially due to codecs, a key challenge lies in designing a generator capable of preserving low-frequency information while accurately reconstructing high-quality mid- and high-frequency content. Inspired by recent advancements in high-sample-rate music separation, speech enhancement, and audio codec models, we propose Apollo, a generative model designed for high-sample-rate audio restoration. Apollo employs an explicit frequency band split module to model the relationships between different frequency bands, allowing for more coherent and higher-quality restored audio. Evaluated on the MUSDB18-HQ and MoisesDB datasets, Apollo consistently outperforms existing SR-GAN models across various bit rates and music genres, particularly excelling in complex scenarios involving mixtures of multiple instruments and vocals. Apollo significantly improves music restoration quality while maintaining computational efficiency. The source code for Apollo is publicly available at https://github.com/JusperLee/Apollo.