Arboretum: A Large Multimodal Dataset Enabling AI for Biodiversity
Chih-Hsuan Yang, Benjamin Feuer, Zaki Jubery, Zi K. Deng, Andre Nakkab, Md Zahid Hasan, Shivani Chiranjeevi, Kelly Marshall, Nirmal Baishnab, Asheesh K Singh, Arti Singh, Soumik Sarkar, Nirav Merchant, Chinmay Hegde, Baskar Ganapathysubramanian
2024-07-01
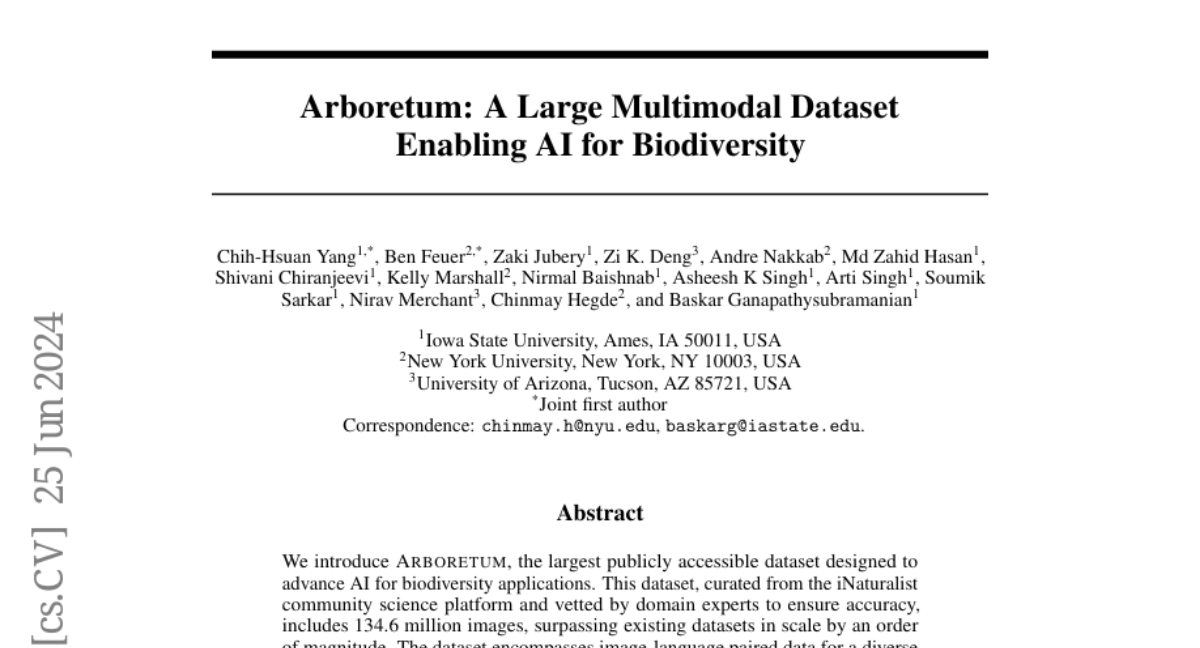
Summary
This paper talks about Arboretum, the largest publicly available dataset designed to help artificial intelligence (AI) understand and work with biodiversity. It includes a massive collection of images and information about various species, making it a valuable resource for researchers and developers.
What's the problem?
In the field of biodiversity research, there is a need for high-quality datasets that can help AI models learn to recognize and classify different species. Existing datasets are often too small or lack detailed information, which limits the effectiveness of AI applications in areas like agriculture and environmental conservation. Without comprehensive data, it becomes challenging to develop tools that can monitor ecosystems and support biodiversity efforts.
What's the solution?
To address this issue, the authors created the Arboretum dataset, which contains 134.6 million images paired with descriptive text about a wide range of species, including birds, insects, plants, fungi, and more. This dataset was curated from the iNaturalist community science platform and reviewed by experts to ensure accuracy. Each image includes scientific names and taxonomic details, allowing AI models to learn from high-quality data. The authors also released several AI models trained on this dataset to demonstrate its effectiveness.
Why it matters?
This research is important because it provides a comprehensive resource that can significantly enhance AI's ability to analyze and understand biodiversity. By making this dataset publicly available, researchers can create better tools for pest control, crop monitoring, and conservation efforts. These advancements are crucial for addressing global challenges like food security and climate change, as they enable more effective management of natural resources and protection of ecosystems.
Abstract
We introduce Arboretum, the largest publicly accessible dataset designed to advance AI for biodiversity applications. This dataset, curated from the iNaturalist community science platform and vetted by domain experts to ensure accuracy, includes 134.6 million images, surpassing existing datasets in scale by an order of magnitude. The dataset encompasses image-language paired data for a diverse set of species from birds (Aves), spiders/ticks/mites (Arachnida), insects (Insecta), plants (Plantae), fungus/mushrooms (Fungi), snails (Mollusca), and snakes/lizards (Reptilia), making it a valuable resource for multimodal vision-language AI models for biodiversity assessment and agriculture research. Each image is annotated with scientific names, taxonomic details, and common names, enhancing the robustness of AI model training. We showcase the value of Arboretum by releasing a suite of CLIP models trained using a subset of 40 million captioned images. We introduce several new benchmarks for rigorous assessment, report accuracy for zero-shot learning, and evaluations across life stages, rare species, confounding species, and various levels of the taxonomic hierarchy. We anticipate that Arboretum will spur the development of AI models that can enable a variety of digital tools ranging from pest control strategies, crop monitoring, and worldwide biodiversity assessment and environmental conservation. These advancements are critical for ensuring food security, preserving ecosystems, and mitigating the impacts of climate change. Arboretum is publicly available, easily accessible, and ready for immediate use. Please see the https://baskargroup.github.io/Arboretum/{project website} for links to our data, models, and code.