Are LLMs Better than Reported? Detecting Label Errors and Mitigating Their Effect on Model Performance
Omer Nahum, Nitay Calderon, Orgad Keller, Idan Szpektor, Roi Reichart
2024-10-28
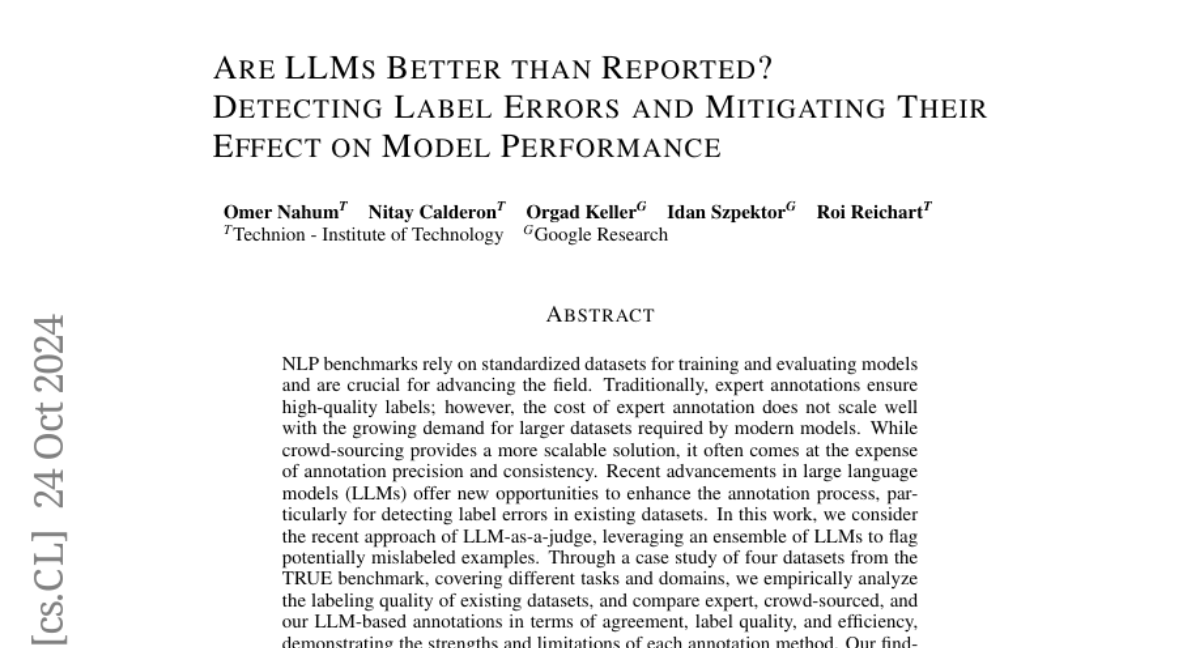
Summary
This paper explores how to improve the performance of large language models (LLMs) by identifying and correcting label errors in datasets used for training and evaluation.
What's the problem?
High-quality datasets are essential for training and evaluating language models, but creating these datasets can be expensive and time-consuming. While expert annotations ensure quality, they don't scale well with the increasing demand for larger datasets. Crowdsourcing is a more scalable option, but it often leads to less accurate labels. Consequently, many datasets contain errors that can mislead models and affect their performance.
What's the solution?
The authors propose using LLMs as a tool to detect label errors in existing datasets. They analyze four different datasets to compare the quality of labels provided by experts, crowdsourced workers, and their LLM-based annotations. Their findings reveal that there are many mislabeled examples in the datasets. By correcting these errors, they show that the performance of language models can improve significantly. They also discuss methods to reduce the impact of mislabeled data during training.
Why it matters?
This research is important because it highlights how label errors can distort the perceived performance of language models. By improving the accuracy of dataset annotations, we can enhance the reliability of AI systems that rely on these models, leading to better outcomes in applications like natural language processing and machine learning.
Abstract
NLP benchmarks rely on standardized datasets for training and evaluating models and are crucial for advancing the field. Traditionally, expert annotations ensure high-quality labels; however, the cost of expert annotation does not scale well with the growing demand for larger datasets required by modern models. While crowd-sourcing provides a more scalable solution, it often comes at the expense of annotation precision and consistency. Recent advancements in large language models (LLMs) offer new opportunities to enhance the annotation process, particularly for detecting label errors in existing datasets. In this work, we consider the recent approach of LLM-as-a-judge, leveraging an ensemble of LLMs to flag potentially mislabeled examples. Through a case study of four datasets from the TRUE benchmark, covering different tasks and domains, we empirically analyze the labeling quality of existing datasets, and compare expert, crowd-sourced, and our LLM-based annotations in terms of agreement, label quality, and efficiency, demonstrating the strengths and limitations of each annotation method. Our findings reveal a substantial number of label errors, which, when corrected, induce a significant upward shift in reported model performance. This suggests that many of the LLMs so-called mistakes are due to label errors rather than genuine model failures. Additionally, we discuss the implications of mislabeled data and propose methods to mitigate them in training to improve model performance.