Are Your LLMs Capable of Stable Reasoning?
Junnan Liu, Hongwei Liu, Linchen Xiao, Ziyi Wang, Kuikun Liu, Songyang Gao, Wenwei Zhang, Songyang Zhang, Kai Chen
2024-12-18
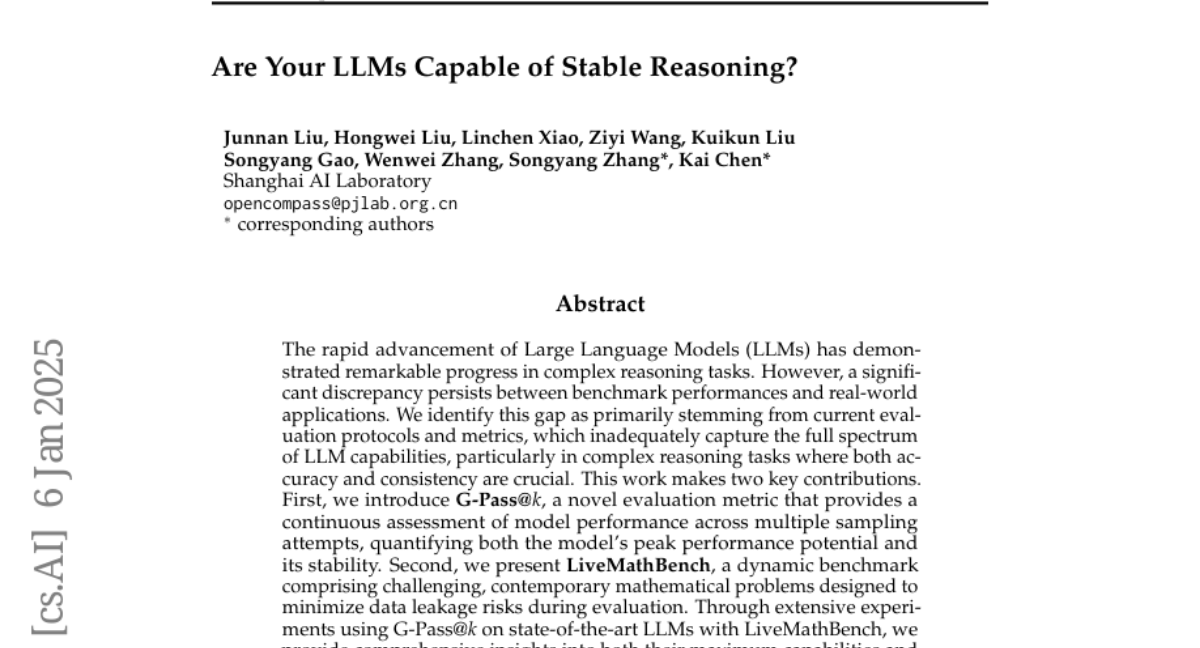
Summary
This paper explores the reasoning abilities of Large Language Models (LLMs) and introduces new methods to better evaluate and enhance their performance in complex reasoning tasks.
What's the problem?
While LLMs have made great strides in understanding and generating text, they often struggle with reasoning tasks that require multiple steps or deep understanding. Current evaluation methods do not fully capture how well these models can reason, leading to a gap between their performance on tests and their effectiveness in real-world applications.
What's the solution?
The authors propose two key innovations: the G-Pass@k evaluation metric, which measures how well models perform across multiple attempts, and LiveMathBench, a new set of challenging math problems designed to test LLMs' reasoning abilities without leaking information. These tools help assess both the peak performance and consistency of LLMs, providing a clearer picture of their capabilities.
Why it matters?
This research is significant because it highlights the need for better evaluation methods for LLMs, particularly in reasoning tasks. By improving how we assess these models, we can develop more effective AI systems that can handle complex problems, which is crucial for applications in education, science, and technology.
Abstract
The rapid advancement of Large Language Models (LLMs) has demonstrated remarkable progress in complex reasoning tasks. However, a significant discrepancy persists between benchmark performances and real-world applications. We identify this gap as primarily stemming from current evaluation protocols and metrics, which inadequately capture the full spectrum of LLM capabilities, particularly in complex reasoning tasks where both accuracy and consistency are crucial. This work makes two key contributions. First, we introduce G-Pass@k, a novel evaluation metric that provides a continuous assessment of model performance across multiple sampling attempts, quantifying both the model's peak performance potential and its stability. Second, we present LiveMathBench, a dynamic benchmark comprising challenging, contemporary mathematical problems designed to minimize data leakage risks during evaluation. Through extensive experiments using G-Pass@k on state-of-the-art LLMs with LiveMathBench, we provide comprehensive insights into both their maximum capabilities and operational consistency. Our findings reveal substantial room for improvement in LLMs' "realistic" reasoning capabilities, highlighting the need for more robust evaluation methods. The benchmark and detailed results are available at: https://github.com/open-compass/GPassK.