Aria: An Open Multimodal Native Mixture-of-Experts Model
Dongxu Li, Yudong Liu, Haoning Wu, Yue Wang, Zhiqi Shen, Bowen Qu, Xinyao Niu, Guoyin Wang, Bei Chen, Junnan Li
2024-10-10
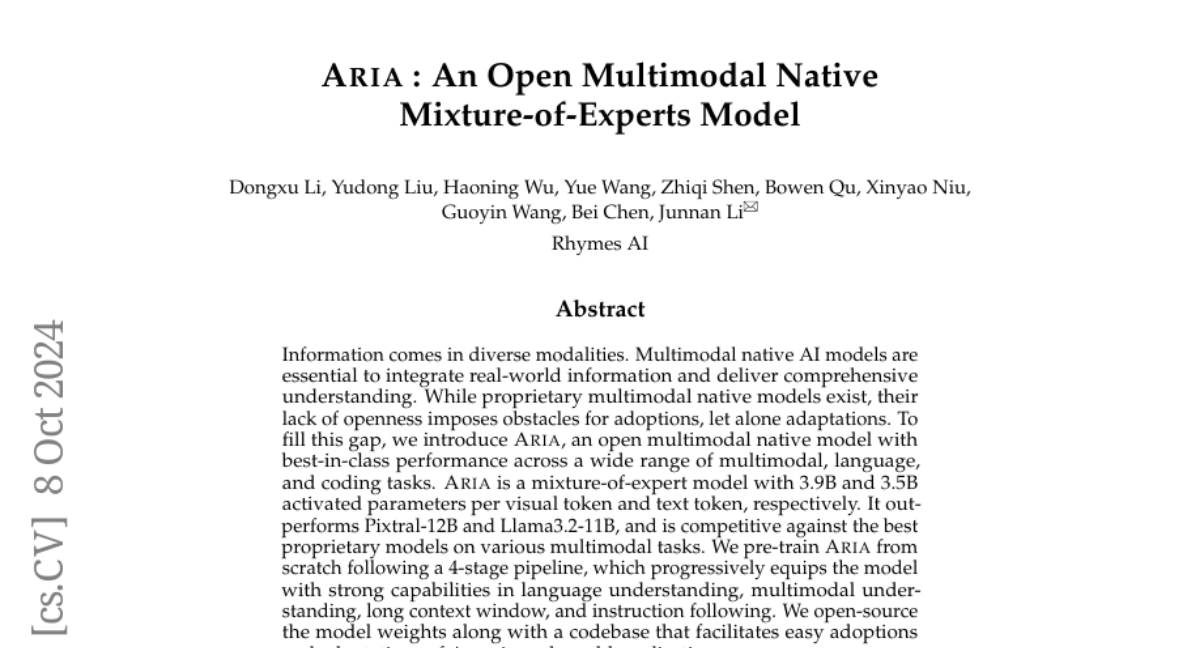
Summary
This paper introduces Aria, an open-source multimodal model that can effectively process and understand various types of information, such as text, images, and videos, using a mixture-of-experts architecture.
What's the problem?
Many existing multimodal models are proprietary, meaning their inner workings and training methods are not shared with the public. This lack of openness makes it difficult for researchers and developers to use, adapt, or improve these models for their own applications. Additionally, there is a need for models that can seamlessly integrate different types of data while maintaining high performance.
What's the solution?
To address these issues, the authors developed Aria, which is designed to be open and accessible. It uses a mixture-of-experts (MoE) architecture that allows it to activate only a portion of its parameters at any time, making it efficient in processing different types of data. Aria was trained in a four-stage process to enhance its capabilities in understanding language and multimodal information. The model has been shown to outperform other models on various tasks while being competitive with top proprietary models.
Why it matters?
This research is significant because it provides an open-source solution that allows other researchers and developers to access advanced AI technology without the restrictions of proprietary systems. By offering Aria as an open model, the authors aim to foster collaboration and innovation in the field of AI, enabling more people to build applications that require understanding complex information from multiple sources.
Abstract
Information comes in diverse modalities. Multimodal native AI models are essential to integrate real-world information and deliver comprehensive understanding. While proprietary multimodal native models exist, their lack of openness imposes obstacles for adoptions, let alone adaptations. To fill this gap, we introduce Aria, an open multimodal native model with best-in-class performance across a wide range of multimodal, language, and coding tasks. Aria is a mixture-of-expert model with 3.9B and 3.5B activated parameters per visual token and text token, respectively. It outperforms Pixtral-12B and Llama3.2-11B, and is competitive against the best proprietary models on various multimodal tasks. We pre-train Aria from scratch following a 4-stage pipeline, which progressively equips the model with strong capabilities in language understanding, multimodal understanding, long context window, and instruction following. We open-source the model weights along with a codebase that facilitates easy adoptions and adaptations of Aria in real-world applications.