ARKit LabelMaker: A New Scale for Indoor 3D Scene Understanding
Guangda Ji, Silvan Weder, Francis Engelmann, Marc Pollefeys, Hermann Blum
2024-10-24
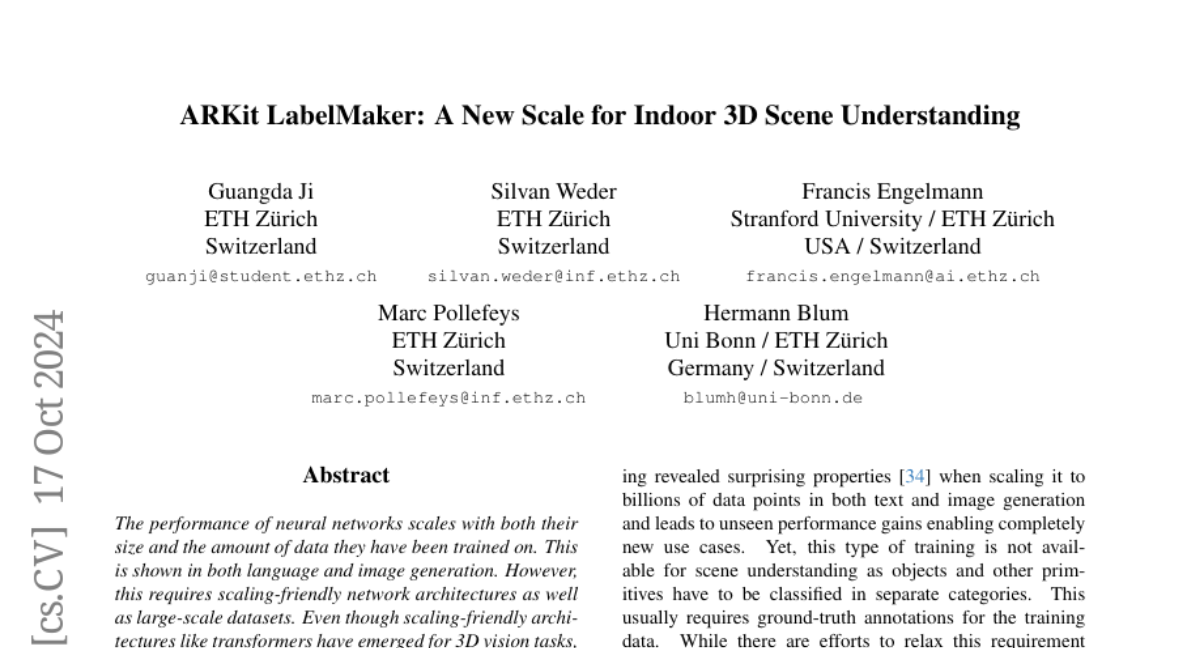
Summary
This paper introduces ARKit LabelMaker, a new system that creates a large-scale dataset for understanding indoor 3D scenes by automatically labeling them with detailed descriptions.
What's the problem?
Training neural networks to understand 3D scenes requires large amounts of labeled data, but creating this data is time-consuming and expensive. Most existing datasets are limited in size and variety, making it hard to train effective models for tasks like recognizing objects or understanding environments.
What's the solution?
The authors developed ARKit LabelMaker, which enhances the existing ARKitScenes dataset by adding dense semantic annotations automatically. They improved an annotation pipeline called LabelMaker to efficiently generate these labels using advanced segmentation models. This allows for the creation of a comprehensive dataset with detailed descriptions of various indoor scenes, making it easier to train models on a larger scale.
Why it matters?
This research is important because it provides a valuable resource for training AI models in understanding 3D environments. By automating the labeling process, it significantly reduces the time and effort needed to create high-quality datasets, which can lead to better performance in applications like robotics, virtual reality, and augmented reality.
Abstract
The performance of neural networks scales with both their size and the amount of data they have been trained on. This is shown in both language and image generation. However, this requires scaling-friendly network architectures as well as large-scale datasets. Even though scaling-friendly architectures like transformers have emerged for 3D vision tasks, the GPT-moment of 3D vision remains distant due to the lack of training data. In this paper, we introduce ARKit LabelMaker, the first large-scale, real-world 3D dataset with dense semantic annotations. Specifically, we complement ARKitScenes dataset with dense semantic annotations that are automatically generated at scale. To this end, we extend LabelMaker, a recent automatic annotation pipeline, to serve the needs of large-scale pre-training. This involves extending the pipeline with cutting-edge segmentation models as well as making it robust to the challenges of large-scale processing. Further, we push forward the state-of-the-art performance on ScanNet and ScanNet200 dataset with prevalent 3D semantic segmentation models, demonstrating the efficacy of our generated dataset.