Artist: Aesthetically Controllable Text-Driven Stylization without Training
Ruixiang Jiang, Changwen Chen
2024-07-23
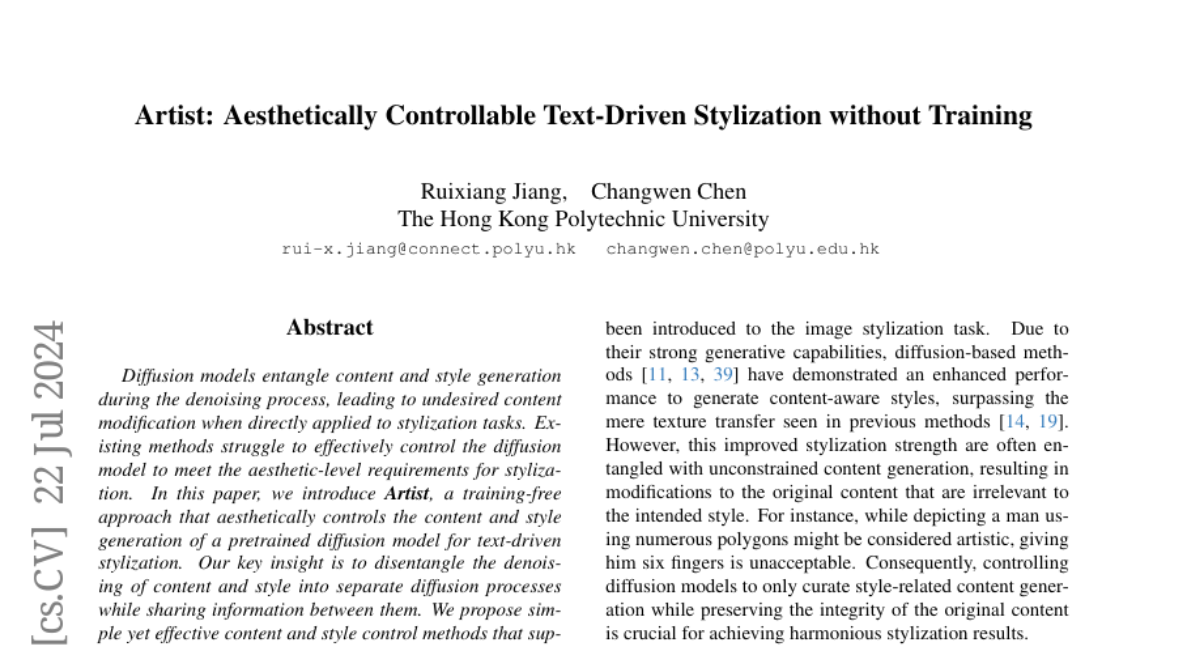
Summary
This paper introduces Artist, a new method that allows users to create stylized images from text descriptions without needing to train any models. It focuses on separating the content and style of images to produce high-quality results that match the user's aesthetic preferences.
What's the problem?
Traditional methods for generating stylized images often mix the content and style together during the image creation process. This can lead to unwanted changes in the original content, making it difficult to achieve the desired artistic effect. Additionally, many existing techniques require extensive training on large datasets, which can be time-consuming and complex.
What's the solution?
Artist solves this problem by using a unique approach that separates the processes of content generation and style application. Instead of training a new model, Artist utilizes a pretrained diffusion model and applies simple methods to control how content and style are combined. This allows it to generate images that maintain important details while aligning well with the specified style from the text prompt. The authors conducted extensive experiments to show that their method effectively meets aesthetic requirements without compromising the quality of the images.
Why it matters?
This research is important because it makes it easier for anyone to create beautiful images just by describing them in words, without needing technical knowledge or access to powerful computers for training. By providing a tool that can generate high-quality stylized images quickly and efficiently, Artist opens up new creative possibilities for artists, designers, and anyone interested in visual content creation.
Abstract
Diffusion models entangle content and style generation during the denoising process, leading to undesired content modification when directly applied to stylization tasks. Existing methods struggle to effectively control the diffusion model to meet the aesthetic-level requirements for stylization. In this paper, we introduce Artist, a training-free approach that aesthetically controls the content and style generation of a pretrained diffusion model for text-driven stylization. Our key insight is to disentangle the denoising of content and style into separate diffusion processes while sharing information between them. We propose simple yet effective content and style control methods that suppress style-irrelevant content generation, resulting in harmonious stylization results. Extensive experiments demonstrate that our method excels at achieving aesthetic-level stylization requirements, preserving intricate details in the content image and aligning well with the style prompt. Furthermore, we showcase the highly controllability of the stylization strength from various perspectives. Code will be released, project home page: https://DiffusionArtist.github.io