Atlas-Chat: Adapting Large Language Models for Low-Resource Moroccan Arabic Dialect
Guokan Shang, Hadi Abdine, Yousef Khoubrane, Amr Mohamed, Yassine Abbahaddou, Sofiane Ennadir, Imane Momayiz, Xuguang Ren, Eric Moulines, Preslav Nakov, Michalis Vazirgiannis, Eric Xing
2024-10-02
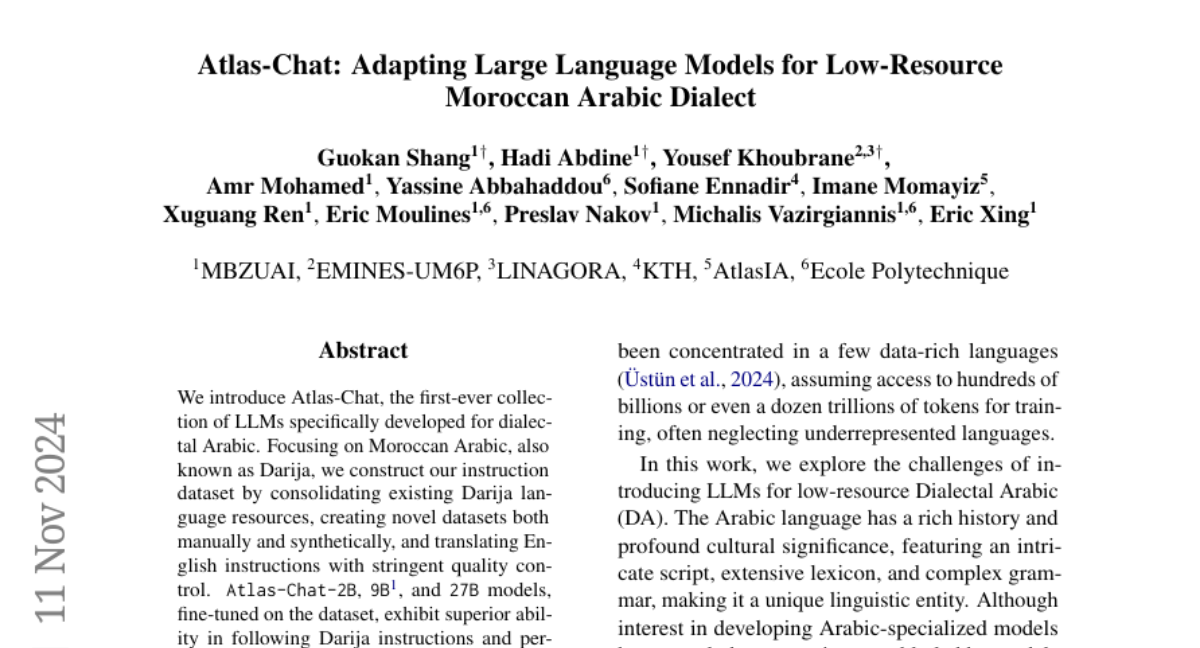
Summary
This paper introduces Atlas-Chat, the first set of large language models specifically designed for Moroccan Arabic (Darija), aimed at improving how AI understands and interacts in this dialect.
What's the problem?
Many AI models excel in widely spoken languages but struggle with less-represented dialects like Moroccan Arabic. This is mainly due to a lack of training data and resources, which makes it difficult for these models to understand and generate text in dialects that are not well-documented.
What's the solution?
The researchers created Atlas-Chat by gathering existing Darija language resources and developing new datasets through manual and synthetic methods. They also translated English instructions into Darija to ensure high-quality training. The models, Atlas-Chat-9B and Atlas-Chat-2B, were fine-tuned on this dataset, allowing them to follow instructions in Darija and perform various natural language processing tasks effectively. The results showed that these models outperformed other specialized Arabic models by a significant margin.
Why it matters?
This research is important because it addresses the gap in AI capabilities for low-resource languages and dialects. By developing Atlas-Chat, the researchers hope to improve communication technologies for millions of Darija speakers and inspire similar efforts for other underrepresented languages, promoting inclusivity in AI development.
Abstract
We introduce Atlas-Chat, the first-ever collection of large language models specifically developed for dialectal Arabic. Focusing on Moroccan Arabic, also known as Darija, we construct our instruction dataset by consolidating existing Darija language resources, creating novel datasets both manually and synthetically, and translating English instructions with stringent quality control. Atlas-Chat-9B and 2B models, fine-tuned on the dataset, exhibit superior ability in following Darija instructions and performing standard NLP tasks. Notably, our models outperform both state-of-the-art and Arabic-specialized LLMs like LLaMa, Jais, and AceGPT, e.g., achieving a 13% performance boost over a larger 13B model on DarijaMMLU, in our newly introduced evaluation suite for Darija covering both discriminative and generative tasks. Furthermore, we perform an experimental analysis of various fine-tuning strategies and base model choices to determine optimal configurations. All our resources are publicly accessible, and we believe our work offers comprehensive design methodologies of instruction-tuning for low-resource language variants, which are often neglected in favor of data-rich languages by contemporary LLMs.