Attention Prompting on Image for Large Vision-Language Models
Runpeng Yu, Weihao Yu, Xinchao Wang
2024-09-25
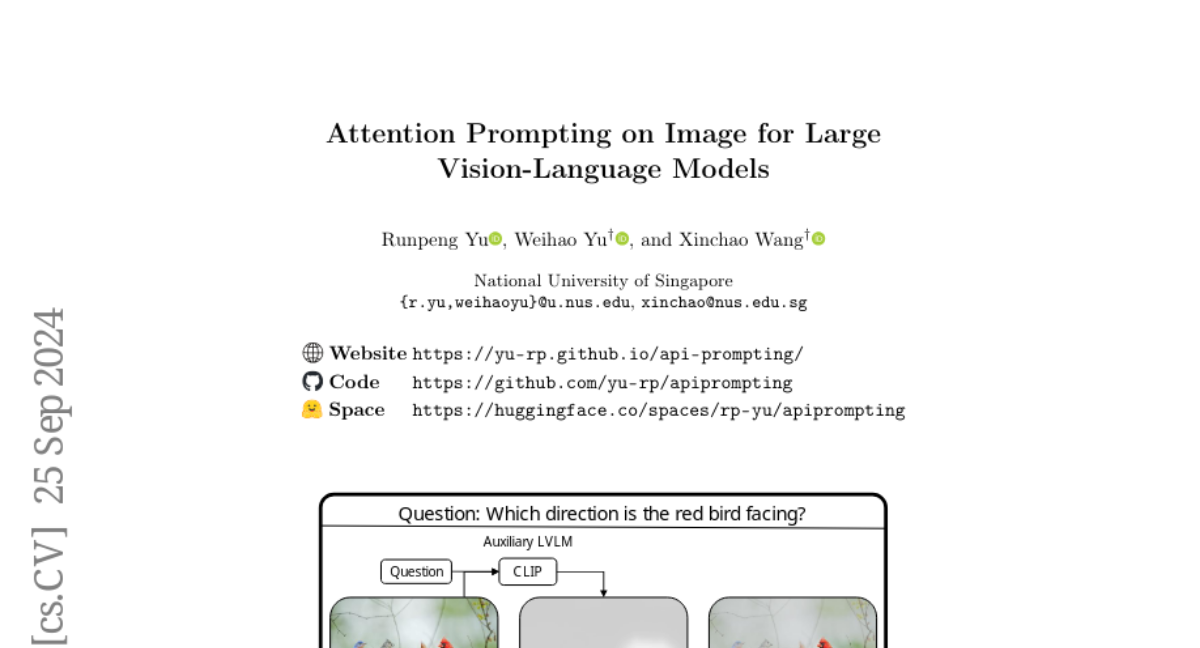
Summary
This paper introduces a new technique called Attention Prompting on Image, which enhances the performance of Large Vision-Language Models (LVLMs) by overlaying a heatmap based on text queries onto input images. This helps the model better understand and follow instructions related to the images.
What's the problem?
While LVLMs can process both images and text, previous methods for improving their performance focused only on the visual aspects of the images without considering the accompanying text instructions. This limitation made it difficult for the models to accurately complete tasks based on text prompts, reducing their effectiveness in various applications.
What's the solution?
To solve this problem, the researchers developed Attention Prompting on Image. This technique generates an attention heatmap that highlights important areas of an image based on a given text query. The heatmap is created using an auxiliary model like CLIP, which analyzes how relevant different parts of the image are to the text. By multiplying this heatmap with the original image, the model can focus on specific regions that matter for completing the task. Extensive testing showed that this method significantly improved the performance of LVLMs on various benchmarks.
Why it matters?
This research is important because it enhances how AI models understand and interact with visual and textual information together. By improving LVLMs' ability to follow text instructions through visual prompts, this technique can lead to better performance in applications like image captioning, visual question answering, and more. Overall, it pushes forward the capabilities of AI in understanding complex information.
Abstract
Compared with Large Language Models (LLMs), Large Vision-Language Models (LVLMs) can also accept images as input, thus showcasing more interesting emergent capabilities and demonstrating impressive performance on various vision-language tasks. Motivated by text prompting in LLMs, visual prompting has been explored to enhance LVLMs' capabilities of perceiving visual information. However, previous visual prompting techniques solely process visual inputs without considering text queries, limiting the models' ability to follow text instructions to complete tasks. To fill this gap, in this work, we propose a new prompting technique named Attention Prompting on Image, which just simply overlays a text-query-guided attention heatmap on the original input image and effectively enhances LVLM on various tasks. Specifically, we generate an attention heatmap for the input image dependent on the text query with an auxiliary model like CLIP. Then the heatmap simply multiplies the pixel values of the original image to obtain the actual input image for the LVLM. Extensive experiments on various vison-language benchmarks verify the effectiveness of our technique. For example, Attention Prompting on Image improves LLaVA-1.5 by 3.8% and 2.9% on MM-Vet and LLaVA-Wild benchmarks, respectively.