AuroraCap: Efficient, Performant Video Detailed Captioning and a New Benchmark
Wenhao Chai, Enxin Song, Yilun Du, Chenlin Meng, Vashisht Madhavan, Omer Bar-Tal, Jeng-Neng Hwang, Saining Xie, Christopher D. Manning
2024-10-07
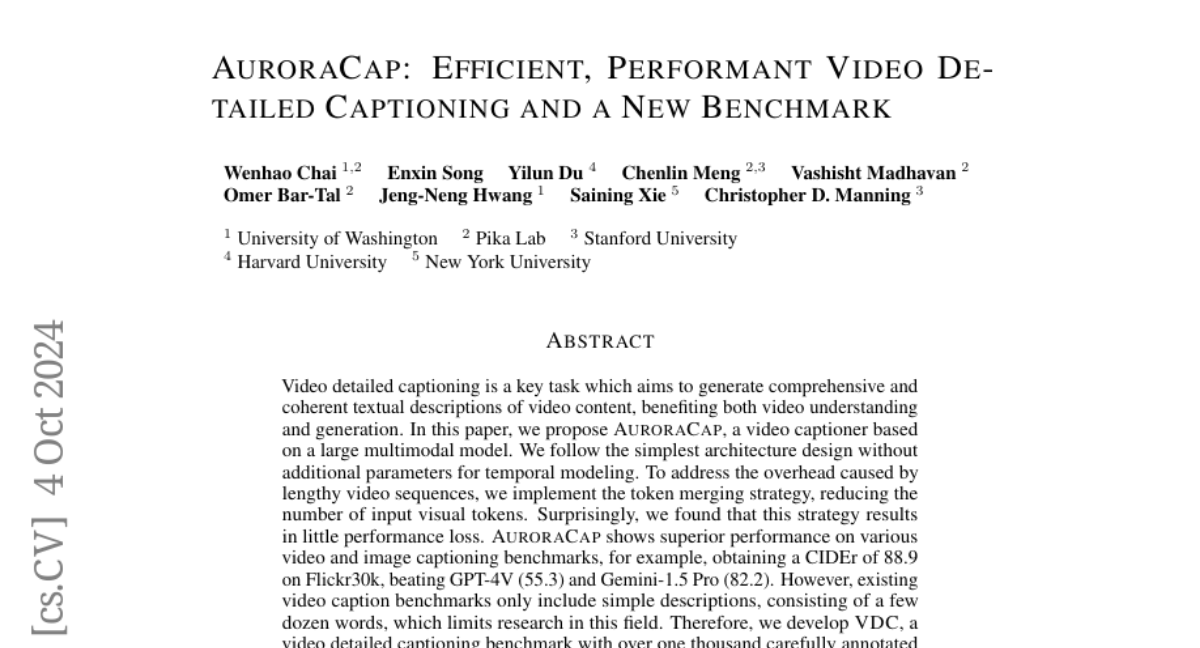
Summary
This paper introduces AuroraCap, a new model for generating detailed captions for videos, and presents a new benchmark for evaluating video captioning performance.
What's the problem?
Generating detailed and coherent captions for videos is challenging because existing methods often produce only simple descriptions that lack depth. This limits the ability to fully understand the content of videos, making it difficult to assess how well models can describe complex scenes and actions.
What's the solution?
To tackle this issue, the authors developed AuroraCap, which uses a large multimodal model to create more comprehensive video captions. They implemented a token merging strategy to reduce the number of visual tokens processed, which helps manage long video sequences without losing performance. Additionally, they created a new benchmark called VDC that includes over a thousand carefully annotated structured captions, allowing for better evaluation of video captioning models. They also introduced a new evaluation metric, VDCscore, which breaks down long captions into shorter question-answer pairs for more accurate assessment.
Why it matters?
This research is significant because it advances the field of video understanding by enabling models to generate more detailed captions. By providing a better evaluation framework and improving the quality of generated captions, AuroraCap can enhance applications such as video summarization, retrieval, and accessibility for people with visual impairments.
Abstract
Video detailed captioning is a key task which aims to generate comprehensive and coherent textual descriptions of video content, benefiting both video understanding and generation. In this paper, we propose AuroraCap, a video captioner based on a large multimodal model. We follow the simplest architecture design without additional parameters for temporal modeling. To address the overhead caused by lengthy video sequences, we implement the token merging strategy, reducing the number of input visual tokens. Surprisingly, we found that this strategy results in little performance loss. AuroraCap shows superior performance on various video and image captioning benchmarks, for example, obtaining a CIDEr of 88.9 on Flickr30k, beating GPT-4V (55.3) and Gemini-1.5 Pro (82.2). However, existing video caption benchmarks only include simple descriptions, consisting of a few dozen words, which limits research in this field. Therefore, we develop VDC, a video detailed captioning benchmark with over one thousand carefully annotated structured captions. In addition, we propose a new LLM-assisted metric VDCscore for bettering evaluation, which adopts a divide-and-conquer strategy to transform long caption evaluation into multiple short question-answer pairs. With the help of human Elo ranking, our experiments show that this benchmark better correlates with human judgments of video detailed captioning quality.