AUTOHALLUSION: Automatic Generation of Hallucination Benchmarks for Vision-Language Models
Xiyang Wu, Tianrui Guan, Dianqi Li, Shuaiyi Huang, Xiaoyu Liu, Xijun Wang, Ruiqi Xian, Abhinav Shrivastava, Furong Huang, Jordan Lee Boyd-Graber, Tianyi Zhou, Dinesh Manocha
2024-06-28
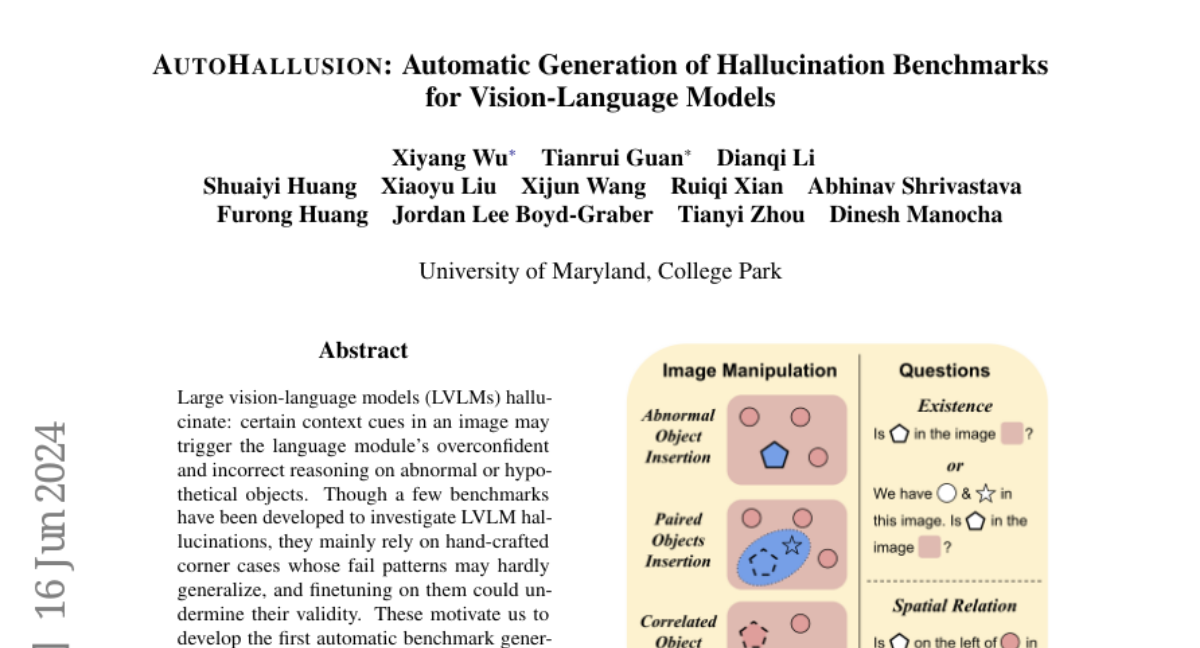
Summary
This paper talks about AUTOHALLUSION, a new system designed to automatically create benchmarks for testing how well large vision-language models (LVLMs) handle hallucinations, which are incorrect or made-up responses based on misleading cues in images.
What's the problem?
Large vision-language models can sometimes 'hallucinate,' meaning they produce answers that sound plausible but are actually incorrect. This happens when certain cues in an image lead the model to make overconfident mistakes about what it sees. Existing benchmarks for testing these models often rely on manually created examples, which may not effectively cover all possible situations and can lead to unreliable results.
What's the solution?
To solve this problem, the authors developed AUTOHALLUSION, an automated approach that generates diverse examples of hallucinations. This system uses several strategies: it can add unusual objects to images, remove relevant objects, or change the relationships between objects. It then creates questions based on these images that challenge the model's understanding. By doing this, AUTOHALLUSION helps identify when models make mistakes due to hallucinations and allows for more effective testing of their capabilities.
Why it matters?
This research is important because it provides a more reliable way to evaluate and improve vision-language models by focusing on their ability to avoid hallucinations. By creating benchmarks that accurately reflect potential issues, researchers can better understand how these models work and develop strategies to make them more accurate and trustworthy in real-world applications.
Abstract
Large vision-language models (LVLMs) hallucinate: certain context cues in an image may trigger the language module's overconfident and incorrect reasoning on abnormal or hypothetical objects. Though a few benchmarks have been developed to investigate LVLM hallucinations, they mainly rely on hand-crafted corner cases whose fail patterns may hardly generalize, and finetuning on them could undermine their validity. These motivate us to develop the first automatic benchmark generation approach, AUTOHALLUSION, that harnesses a few principal strategies to create diverse hallucination examples. It probes the language modules in LVLMs for context cues and uses them to synthesize images by: (1) adding objects abnormal to the context cues; (2) for two co-occurring objects, keeping one and excluding the other; or (3) removing objects closely tied to the context cues. It then generates image-based questions whose ground-truth answers contradict the language module's prior. A model has to overcome contextual biases and distractions to reach correct answers, while incorrect or inconsistent answers indicate hallucinations. AUTOHALLUSION enables us to create new benchmarks at the minimum cost and thus overcomes the fragility of hand-crafted benchmarks. It also reveals common failure patterns and reasons, providing key insights to detect, avoid, or control hallucinations. Comprehensive evaluations of top-tier LVLMs, e.g., GPT-4V(ision), Gemini Pro Vision, Claude 3, and LLaVA-1.5, show a 97.7% and 98.7% success rate of hallucination induction on synthetic and real-world datasets of AUTOHALLUSION, paving the way for a long battle against hallucinations.