AutoMIR: Effective Zero-Shot Medical Information Retrieval without Relevance Labels
Lei Li, Xiangxu Zhang, Xiao Zhou, Zheng Liu
2024-10-31
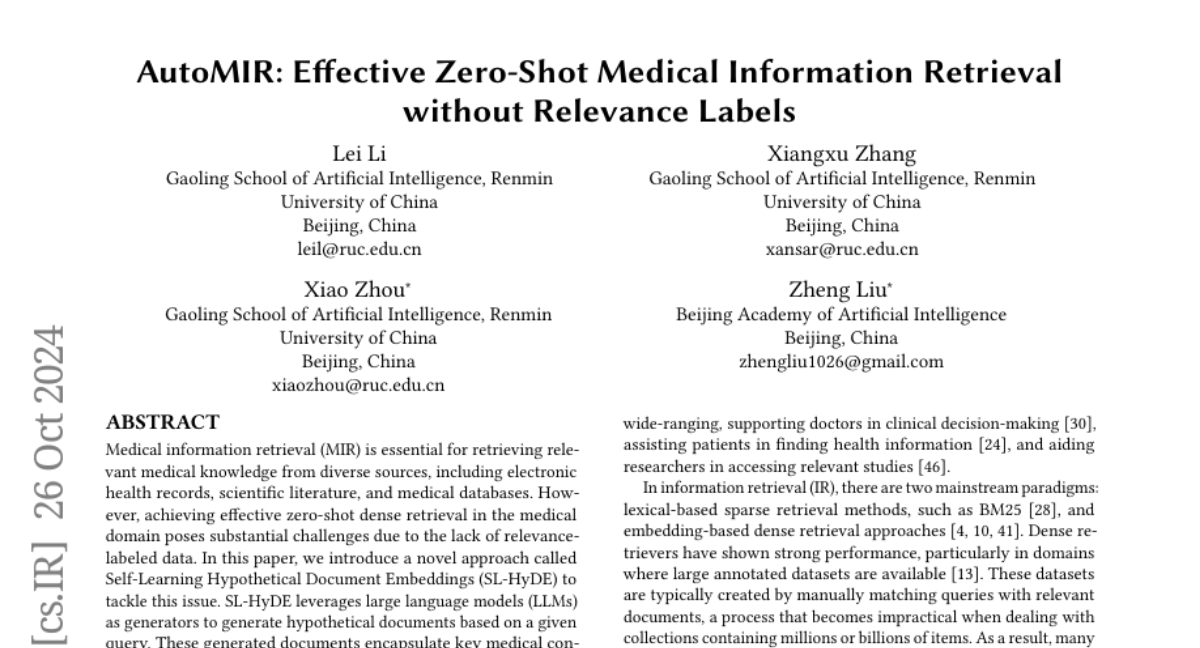
Summary
This paper presents AutoMIR, a new method for retrieving medical information without needing labeled data to determine what is relevant, using a technique called Self-Learning Hypothetical Document Embeddings (SL-HyDE).
What's the problem?
In the medical field, finding relevant information from various sources like health records and scientific literature is crucial. However, creating effective systems for retrieving this information is challenging because there often isn't labeled data that indicates which documents are relevant to specific queries. This lack of data makes it hard to train models that can accurately find the information users need.
What's the solution?
To address this challenge, the authors propose SL-HyDE, which uses large language models to generate hypothetical documents based on user queries. These documents contain important medical context that helps a retrieval system identify real, relevant documents. The method learns from unlabeled medical data, allowing it to improve over time without needing specific relevance labels. Additionally, they introduce the Chinese Medical Information Retrieval Benchmark (CMIRB) to evaluate how well different models perform in real-world medical scenarios.
Why it matters?
This research is important because it provides a way to improve how medical information is retrieved without relying on labeled data, which is often scarce in this field. By developing a system that can learn and adapt using existing data, AutoMIR can enhance the efficiency and accuracy of medical information retrieval, ultimately benefiting healthcare professionals and researchers who need quick access to reliable information.
Abstract
Medical information retrieval (MIR) is essential for retrieving relevant medical knowledge from diverse sources, including electronic health records, scientific literature, and medical databases. However, achieving effective zero-shot dense retrieval in the medical domain poses substantial challenges due to the lack of relevance-labeled data. In this paper, we introduce a novel approach called Self-Learning Hypothetical Document Embeddings (SL-HyDE) to tackle this issue. SL-HyDE leverages large language models (LLMs) as generators to generate hypothetical documents based on a given query. These generated documents encapsulate key medical context, guiding a dense retriever in identifying the most relevant documents. The self-learning framework progressively refines both pseudo-document generation and retrieval, utilizing unlabeled medical corpora without requiring any relevance-labeled data. Additionally, we present the Chinese Medical Information Retrieval Benchmark (CMIRB), a comprehensive evaluation framework grounded in real-world medical scenarios, encompassing five tasks and ten datasets. By benchmarking ten models on CMIRB, we establish a rigorous standard for evaluating medical information retrieval systems. Experimental results demonstrate that SL-HyDE significantly surpasses existing methods in retrieval accuracy while showcasing strong generalization and scalability across various LLM and retriever configurations. CMIRB data and evaluation code are publicly available at: https://github.com/CMIRB-benchmark/CMIRB.