Autoregressive Speech Synthesis without Vector Quantization
Lingwei Meng, Long Zhou, Shujie Liu, Sanyuan Chen, Bing Han, Shujie Hu, Yanqing Liu, Jinyu Li, Sheng Zhao, Xixin Wu, Helen Meng, Furu Wei
2024-07-13
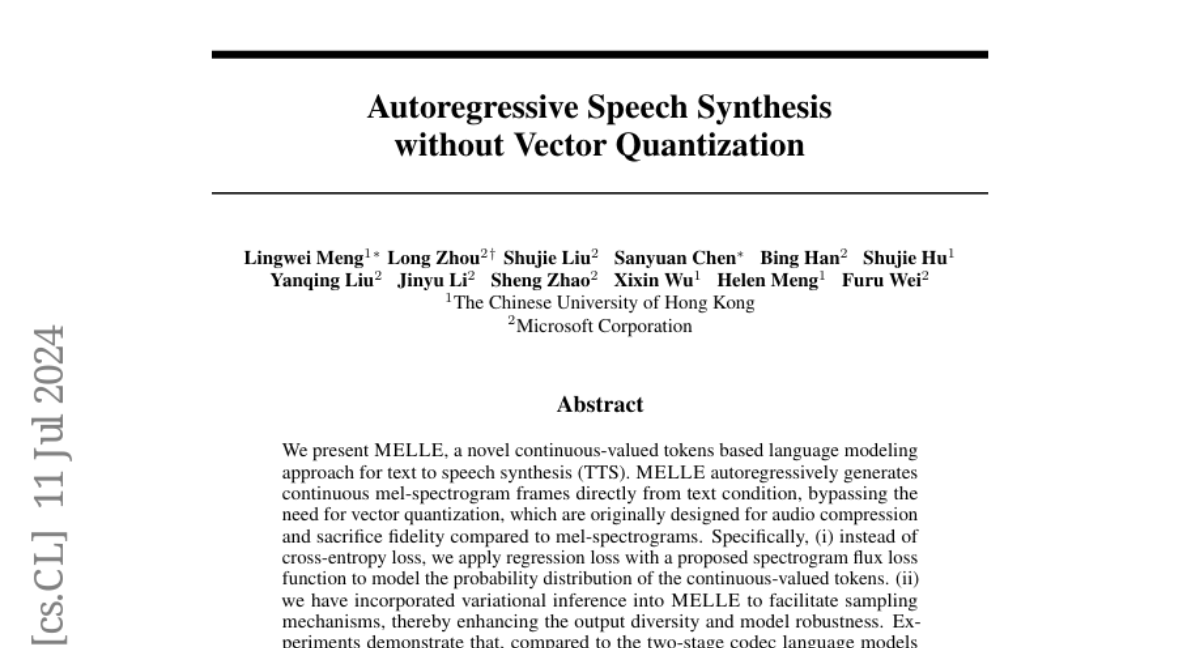
Summary
This paper introduces MELLE, a new method for converting text into speech that uses continuous-valued tokens instead of traditional methods. This approach aims to improve the quality and efficiency of text-to-speech synthesis.
What's the problem?
Many existing text-to-speech systems rely on a process called vector quantization, which compresses audio data but can reduce the quality of the sound. This can lead to issues where the generated speech sounds less natural or clear. Additionally, traditional methods often use complex two-step processes that can be inefficient and may not produce the best results.
What's the solution?
MELLE solves these problems by generating continuous mel-spectrogram frames directly from text input without needing vector quantization. It uses a new type of loss function called regression loss, along with a special technique called spectrogram flux loss, to better model how speech should sound. MELLE also incorporates variational inference, which helps improve the variety and robustness of the generated speech. This single-stage approach simplifies the process and enhances performance compared to older two-stage models like VALL-E.
Why it matters?
This research is important because it represents a significant advancement in text-to-speech technology. By improving how machines generate speech from text, MELLE can lead to more natural-sounding voices in applications such as virtual assistants, audiobooks, and accessibility tools for people with disabilities. The ability to produce high-quality speech more efficiently can enhance user experiences across various platforms.
Abstract
We present MELLE, a novel continuous-valued tokens based language modeling approach for text to speech synthesis (TTS). MELLE autoregressively generates continuous mel-spectrogram frames directly from text condition, bypassing the need for vector quantization, which are originally designed for audio compression and sacrifice fidelity compared to mel-spectrograms. Specifically, (i) instead of cross-entropy loss, we apply regression loss with a proposed spectrogram flux loss function to model the probability distribution of the continuous-valued tokens. (ii) we have incorporated variational inference into MELLE to facilitate sampling mechanisms, thereby enhancing the output diversity and model robustness. Experiments demonstrate that, compared to the two-stage codec language models VALL-E and its variants, the single-stage MELLE mitigates robustness issues by avoiding the inherent flaws of sampling discrete codes, achieves superior performance across multiple metrics, and, most importantly, offers a more streamlined paradigm. See https://aka.ms/melle for demos of our work.