Auxiliary-Loss-Free Load Balancing Strategy for Mixture-of-Experts
Lean Wang, Huazuo Gao, Chenggang Zhao, Xu Sun, Damai Dai
2024-08-29
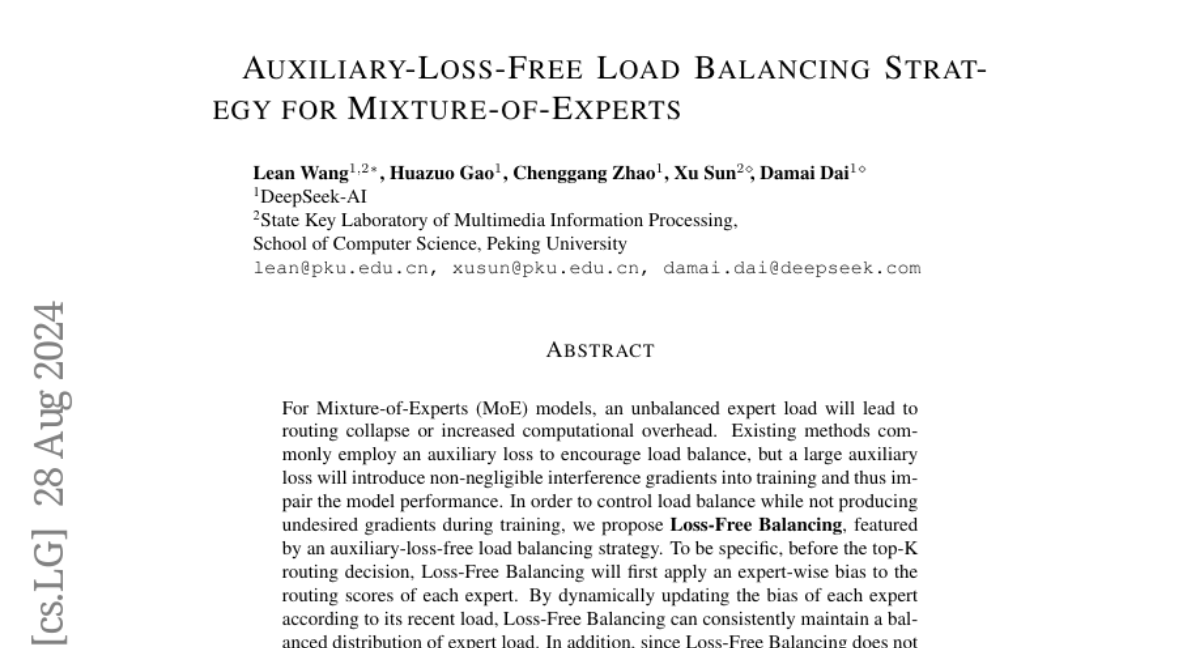
Summary
This paper discusses a new strategy called Loss-Free Balancing for managing the workload of Mixture-of-Experts (MoE) models, which helps improve their efficiency without negatively affecting performance.
What's the problem?
In MoE models, if some experts handle too many tasks while others are underused, it can lead to problems like routing collapse (where the model fails to effectively direct requests to the right experts) and increased computational costs. Existing methods often use an auxiliary loss to encourage a balanced workload among experts, but this can introduce unwanted complications that hurt the model's performance.
What's the solution?
The authors propose Loss-Free Balancing, which avoids using auxiliary losses that can interfere with training. Instead, this method adjusts the routing scores of each expert based on how much work they have recently handled. By dynamically updating these scores, Loss-Free Balancing keeps the workload balanced among experts without creating negative effects on training. This approach has been tested on MoE models and has shown to improve both performance and load balance compared to traditional methods.
Why it matters?
This research is important because it enhances the efficiency of MoE models, allowing them to perform better while using fewer resources. By improving how these models manage their workload, Loss-Free Balancing can lead to faster and more effective AI systems, which is crucial for applications that rely on complex computations.
Abstract
For Mixture-of-Experts (MoE) models, an unbalanced expert load will lead to routing collapse or increased computational overhead. Existing methods commonly employ an auxiliary loss to encourage load balance, but a large auxiliary loss will introduce non-negligible interference gradients into training and thus impair the model performance. In order to control load balance while not producing undesired gradients during training, we propose Loss-Free Balancing, featured by an auxiliary-loss-free load balancing strategy. To be specific, before the top-K routing decision, Loss-Free Balancing will first apply an expert-wise bias to the routing scores of each expert. By dynamically updating the bias of each expert according to its recent load, Loss-Free Balancing can consistently maintain a balanced distribution of expert load. In addition, since Loss-Free Balancing does not produce any interference gradients, it also elevates the upper bound of model performance gained from MoE training. We validate the performance of Loss-Free Balancing on MoE models with up to 3B parameters trained on up to 200B tokens. Experimental results show that Loss-Free Balancing achieves both better performance and better load balance compared with traditional auxiliary-loss-controlled load balancing strategies.