Baichuan Alignment Technical Report
Mingan Lin, Fan Yang, Yanjun Shen, Haoze Sun, Tianpeng Li, Tao Zhang, Chenzheng Zhu, Tao Zhang, Miao Zheng, Xu Li, Yijie Zhou, Mingyang Chen, Yanzhao Qin, Youquan Li, Hao Liang, Fei Li, Yadong Li, Mang Wang, Guosheng Dong, Kun Fang, Jianhua Xu, Bin Cui
2024-10-22
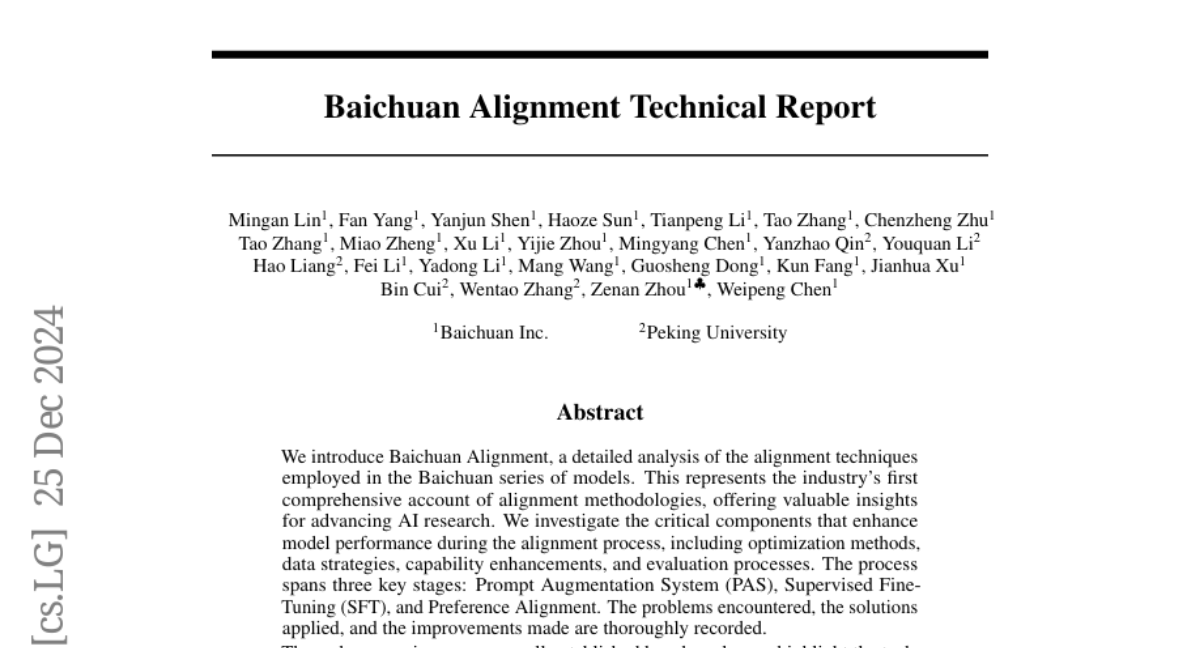
Summary
This paper discusses Baichuan Alignment, a comprehensive analysis of the techniques used to improve the performance of the Baichuan series of AI models, focusing on how these models can be better aligned with user needs.
What's the problem?
As AI models become more advanced, it is crucial to ensure they perform well and align with human preferences. However, existing methods for aligning these models often lack detailed documentation and understanding, making it difficult for researchers to replicate or build upon previous work. This can lead to inconsistencies in how well models perform across different tasks.
What's the solution?
The authors present Baichuan Alignment, which outlines a structured approach to aligning AI models through three main stages: Prompt Augmentation System (PAS), Supervised Fine-Tuning (SFT), and Preference Alignment. They analyze various components that enhance model performance, such as optimization methods and data strategies. The report includes comparisons with established benchmarks to demonstrate the advancements achieved through these alignment techniques, showing significant improvements in user experience and model capabilities.
Why it matters?
This research is important because it provides a clear framework for improving AI model alignment, which can lead to better performance in real-world applications. By sharing their findings and methodologies openly, the authors aim to foster collaboration within the AI community, helping others develop more effective and reliable AI systems that meet user needs.
Abstract
We introduce Baichuan Alignment, a detailed analysis of the alignment techniques employed in the Baichuan series of models. This represents the industry's first comprehensive account of alignment methodologies, offering valuable insights for advancing AI research. We investigate the critical components that enhance model performance during the alignment process, including optimization methods, data strategies, capability enhancements, and evaluation processes. The process spans three key stages: Prompt Augmentation System (PAS), Supervised Fine-Tuning (SFT), and Preference Alignment. The problems encountered, the solutions applied, and the improvements made are thoroughly recorded. Through comparisons across well-established benchmarks, we highlight the technological advancements enabled by Baichuan Alignment. Baichuan-Instruct is an internal model, while Qwen2-Nova-72B and Llama3-PBM-Nova-70B are instruct versions of the Qwen2-72B and Llama-3-70B base models, optimized through Baichuan Alignment. Baichuan-Instruct demonstrates significant improvements in core capabilities, with user experience gains ranging from 17% to 28%, and performs exceptionally well on specialized benchmarks. In open-source benchmark evaluations, both Qwen2-Nova-72B and Llama3-PBM-Nova-70B consistently outperform their respective official instruct versions across nearly all datasets. This report aims to clarify the key technologies behind the alignment process, fostering a deeper understanding within the community. Llama3-PBM-Nova-70B model is available at https://huggingface.co/PKU-Baichuan-MLSystemLab/Llama3-PBM-Nova-70B.