Baichuan-Omni-1.5 Technical Report
Yadong Li, Jun Liu, Tao Zhang, Tao Zhang, Song Chen, Tianpeng Li, Zehuan Li, Lijun Liu, Lingfeng Ming, Guosheng Dong, Da Pan, Chong Li, Yuanbo Fang, Dongdong Kuang, Mingrui Wang, Chenglin Zhu, Youwei Zhang, Hongyu Guo, Fengyu Zhang, Yuran Wang, Bowen Ding, Wei Song
2025-01-28
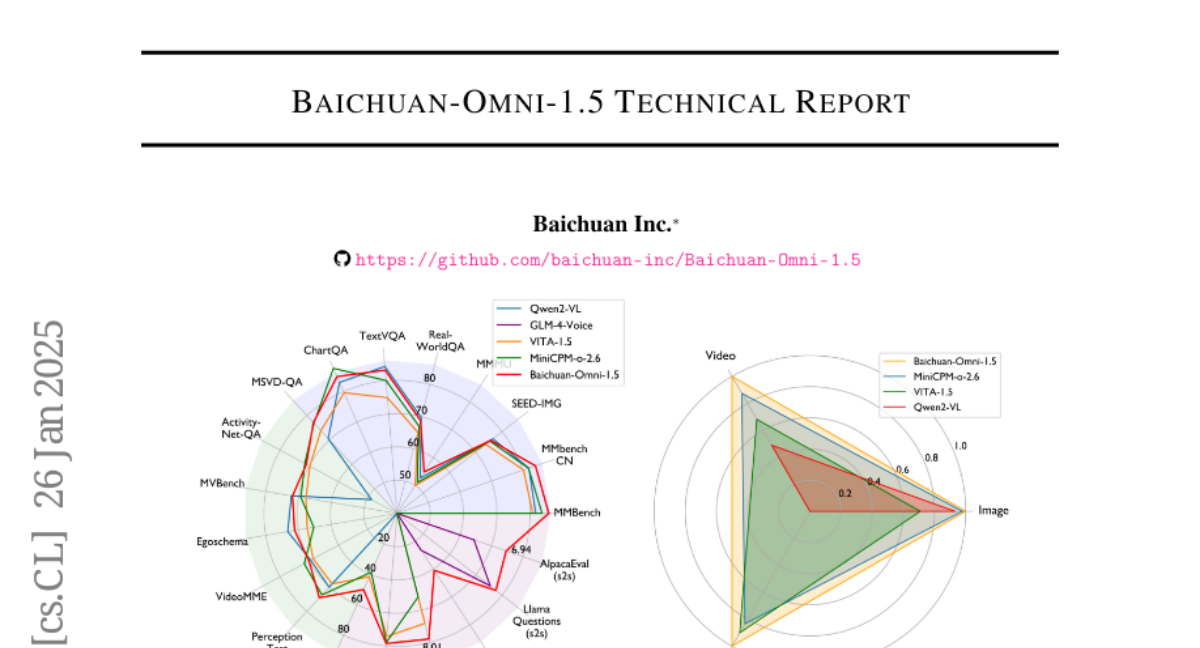
Summary
This paper talks about Qwen2.5-1M, a new series of AI language models that can handle incredibly long texts of up to 1 million words or symbols at once. These models are much better at understanding and working with long pieces of information compared to their older versions.
What's the problem?
Previous AI language models were limited in how much text they could process at one time, usually around 128,000 words. This made it hard for them to understand very long documents or conversations, which is important for tasks like analyzing entire books or long legal documents.
What's the solution?
The researchers created Qwen2.5-1M by using special training techniques. They made fake long texts for the AI to practice on, slowly increased the length of texts during training, and used a step-by-step approach to fine-tune the model. They also created a new way for the AI to quickly read and understand long texts without using too much computer power. This includes tricks like only paying attention to the most important parts of the text and breaking long texts into smaller chunks.
Why it matters?
This research matters because it allows AI to work with much longer pieces of text, which opens up new possibilities for how we can use AI in real-world situations. For example, it could help lawyers review long legal documents, assist researchers in analyzing entire scientific papers, or help writers create longer, more coherent stories. The fact that the researchers are sharing their work openly also means that other scientists and companies can build on this technology, potentially leading to even more advanced AI systems in the future.
Abstract
We introduce Baichuan-Omni-1.5, an omni-modal model that not only has omni-modal understanding capabilities but also provides end-to-end audio generation capabilities. To achieve fluent and high-quality interaction across modalities without compromising the capabilities of any modality, we prioritized optimizing three key aspects. First, we establish a comprehensive data cleaning and synthesis pipeline for multimodal data, obtaining about 500B high-quality data (text, audio, and vision). Second, an audio-tokenizer (Baichuan-Audio-Tokenizer) has been designed to capture both semantic and acoustic information from audio, enabling seamless integration and enhanced compatibility with MLLM. Lastly, we designed a multi-stage training strategy that progressively integrates multimodal alignment and multitask fine-tuning, ensuring effective synergy across all modalities. Baichuan-Omni-1.5 leads contemporary models (including GPT4o-mini and MiniCPM-o 2.6) in terms of comprehensive omni-modal capabilities. Notably, it achieves results comparable to leading models such as Qwen2-VL-72B across various multimodal medical benchmarks.