Balancing Pipeline Parallelism with Vocabulary Parallelism
Man Tsung Yeung, Penghui Qi, Min Lin, Xinyi Wan
2024-11-11
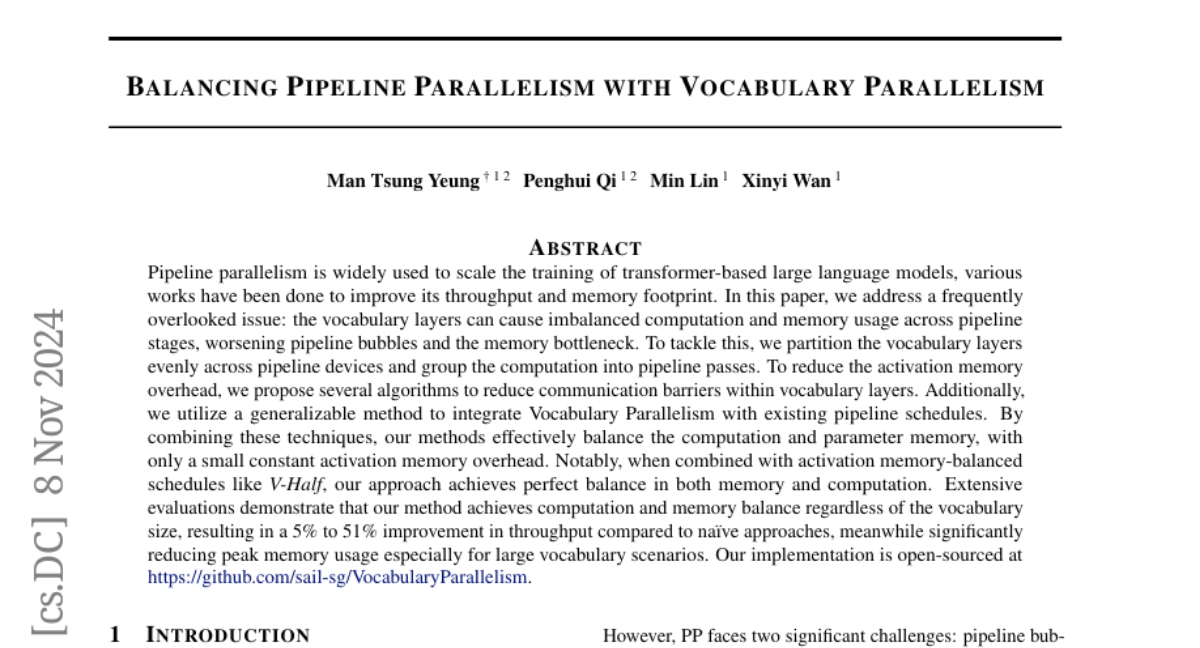
Summary
This paper discusses a new method called Vocabulary Parallelism that improves how large language models (LLMs) are trained by balancing the workload across different parts of the model.
What's the problem?
When training large language models using a technique called pipeline parallelism, there can be imbalances in how much work different parts of the model have to do. This imbalance can lead to wasted time and memory because some parts may be overloaded while others are underused. Specifically, the vocabulary layers, which handle words, can cause these issues and create bottlenecks in processing.
What's the solution?
To solve this problem, the authors propose a method that evenly distributes the vocabulary layers across all devices used in training. They also group computations into stages to minimize communication delays between these layers. By doing this, they ensure that both computation and memory usage are balanced, leading to significant improvements in training speed and efficiency. Their approach allows for better performance even when working with large vocabularies.
Why it matters?
This research is important because it enhances the efficiency of training large language models, which are crucial for many AI applications today. By improving how these models handle vocabulary and memory, the new method can help researchers and developers train larger models more quickly and effectively, ultimately leading to better AI systems.
Abstract
Pipeline parallelism is widely used to scale the training of transformer-based large language models, various works have been done to improve its throughput and memory footprint. In this paper, we address a frequently overlooked issue: the vocabulary layers can cause imbalanced computation and memory usage across pipeline stages, worsening pipeline bubbles and the memory bottleneck. To tackle this, we partition the vocabulary layers evenly across pipeline devices and group the computation into pipeline passes. To reduce the activation memory overhead, we propose several algorithms to reduce communication barriers within vocabulary layers. Additionally, we utilize a generalizable method to integrate Vocabulary Parallelism with existing pipeline schedules. By combining these techniques, our methods effectively balance the computation and parameter memory, with only a small constant activation memory overhead. Notably, when combined with activation memory-balanced schedules like V-Half, our approach achieves perfect balance in both memory and computation. Extensive evaluations demonstrate that our method achieves computation and memory balance regardless of the vocabulary size, resulting in a 5% to 51% improvement in throughput compared to naive approaches, meanwhile significantly reducing peak memory usage especially for large vocabulary scenarios. Our implementation is open-sourced at https://github.com/sail-sg/VocabularyParallelism .