BAM! Just Like That: Simple and Efficient Parameter Upcycling for Mixture of Experts
Qizhen Zhang, Nikolas Gritsch, Dwaraknath Gnaneshwar, Simon Guo, David Cairuz, Bharat Venkitesh, Jakob Foerster, Phil Blunsom, Sebastian Ruder, Ahmet Ustun, Acyr Locatelli
2024-08-16
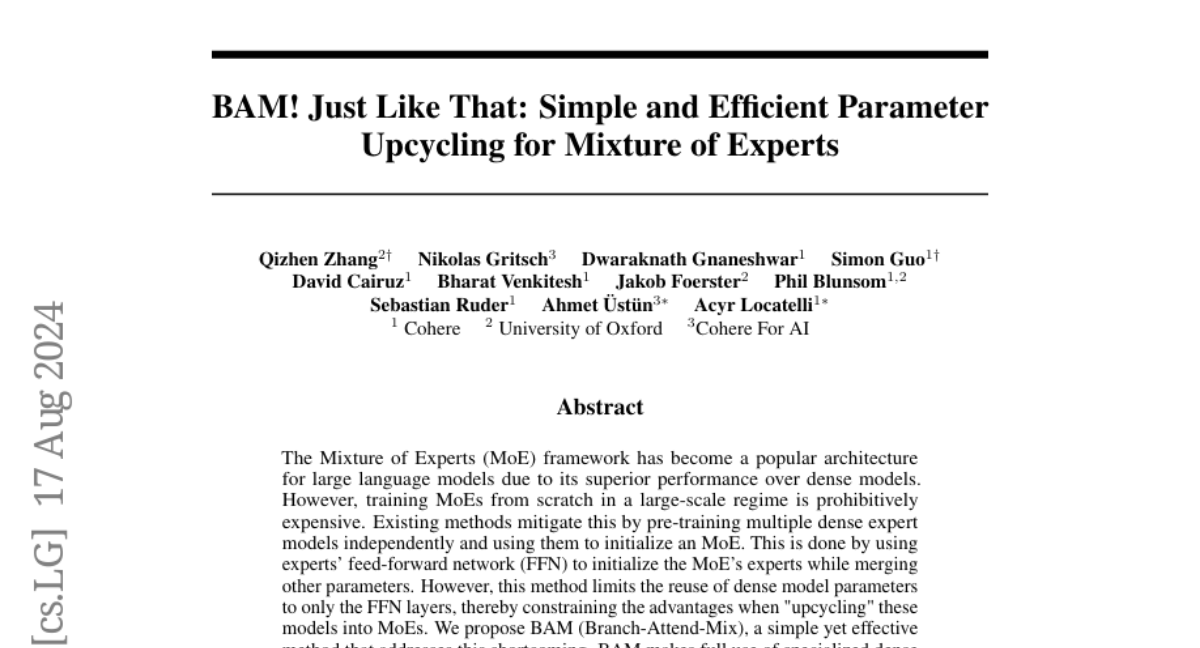
Summary
This paper presents BAM (Branch-Attend-Mix), a new method for improving the training of large language models called Mixture of Experts (MoE) by efficiently reusing parameters from existing dense models.
What's the problem?
Training large language models from scratch can be very expensive and time-consuming. Current methods to create MoE models often only reuse a small part of the information from dense models, which limits their effectiveness and increases costs.
What's the solution?
BAM addresses this issue by fully utilizing the parameters from dense models, not just for initializing some layers but also for the attention mechanisms. It introduces two strategies for using these attention parameters: one that uses all attention parameters for better performance, and another that shares certain parameters across experts to improve efficiency. Additionally, BAM allows different parts of the model to be computed at the same time, speeding up the process.
Why it matters?
This research is important because it makes training large language models more efficient and cost-effective. By improving how these models are built and trained, we can achieve better performance in various applications like natural language processing, making AI tools more powerful and accessible.
Abstract
The Mixture of Experts (MoE) framework has become a popular architecture for large language models due to its superior performance over dense models. However, training MoEs from scratch in a large-scale regime is prohibitively expensive. Existing methods mitigate this by pre-training multiple dense expert models independently and using them to initialize an MoE. This is done by using experts' feed-forward network (FFN) to initialize the MoE's experts while merging other parameters. However, this method limits the reuse of dense model parameters to only the FFN layers, thereby constraining the advantages when "upcycling" these models into MoEs. We propose BAM (Branch-Attend-Mix), a simple yet effective method that addresses this shortcoming. BAM makes full use of specialized dense models by not only using their FFN to initialize the MoE layers but also leveraging experts' attention parameters fully by initializing them into a soft-variant of Mixture of Attention (MoA) layers. We explore two methods for upcycling attention parameters: 1) initializing separate attention experts from dense models including all attention parameters for the best model performance; and 2) sharing key and value parameters across all experts to facilitate for better inference efficiency. To further improve efficiency, we adopt a parallel attention transformer architecture to MoEs, which allows the attention experts and FFN experts to be computed concurrently. Our experiments on seed models ranging from 590 million to 2 billion parameters demonstrate that BAM surpasses baselines in both perplexity and downstream task performance, within the same computational and data constraints.