beeFormer: Bridging the Gap Between Semantic and Interaction Similarity in Recommender Systems
Vojtěch Vančura, Pavel Kordík, Milan Straka
2024-09-17
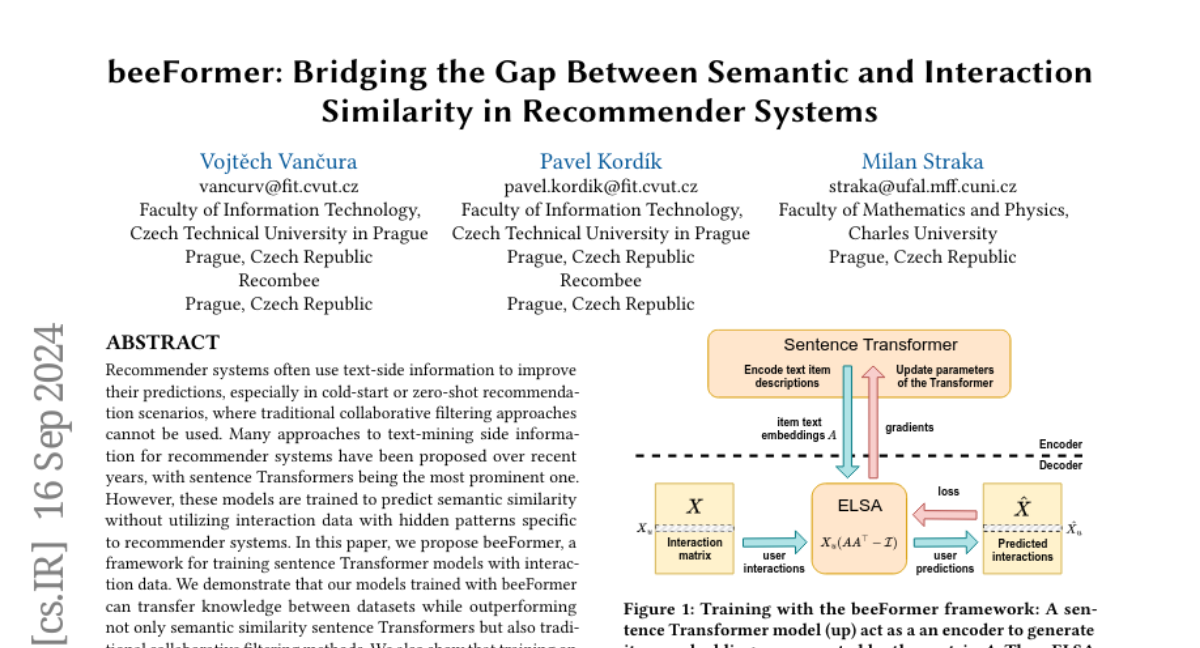
Summary
This paper introduces beeFormer, a new framework that improves recommender systems by combining semantic information (what items are about) with interaction data (how users have interacted with items).
What's the problem?
Recommender systems often struggle when they have little or no interaction data, especially for new items. Traditional methods rely on either the content of the items (like descriptions) or past user interactions, but they don't effectively combine both types of information, leading to less accurate recommendations.
What's the solution?
beeFormer addresses this issue by training sentence Transformer models using both text descriptions and user interaction data. It allows the model to learn from different datasets and understand how items are related not just by their content but also by how users interact with them. This means that even new items without prior interactions can be recommended effectively. The framework also uses advanced techniques to manage large datasets efficiently.
Why it matters?
This research is significant because it enhances the ability of recommender systems to provide accurate suggestions, even in situations where data is limited. By bridging the gap between what items are and how users interact with them, beeFormer can help improve user experiences in various applications like online shopping, streaming services, and content recommendations.
Abstract
Recommender systems often use text-side information to improve their predictions, especially in cold-start or zero-shot recommendation scenarios, where traditional collaborative filtering approaches cannot be used. Many approaches to text-mining side information for recommender systems have been proposed over recent years, with sentence Transformers being the most prominent one. However, these models are trained to predict semantic similarity without utilizing interaction data with hidden patterns specific to recommender systems. In this paper, we propose beeFormer, a framework for training sentence Transformer models with interaction data. We demonstrate that our models trained with beeFormer can transfer knowledge between datasets while outperforming not only semantic similarity sentence Transformers but also traditional collaborative filtering methods. We also show that training on multiple datasets from different domains accumulates knowledge in a single model, unlocking the possibility of training universal, domain-agnostic sentence Transformer models to mine text representations for recommender systems. We release the source code, trained models, and additional details allowing replication of our experiments at https://github.com/recombee/beeformer.