μ-Bench: A Vision-Language Benchmark for Microscopy Understanding
Alejandro Lozano, Jeffrey Nirschl, James Burgess, Sanket Rajan Gupte, Yuhui Zhang, Alyssa Unell, Serena Yeung-Levy
2024-07-03
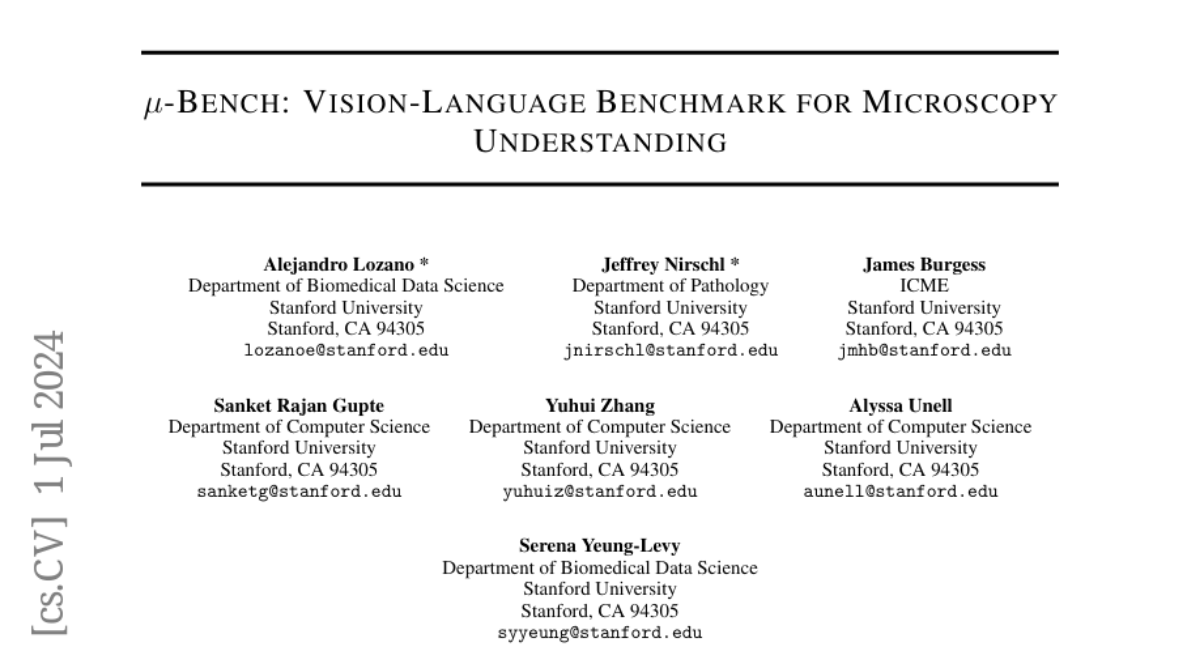
Summary
This paper talks about μ-Bench, a new benchmark designed to help evaluate how well vision-language models (VLMs) can understand and analyze images from microscopy in the fields of biology and pathology.
What's the problem?
The main problem is that while modern microscopy can produce huge amounts of detailed image data, there aren't enough standardized and diverse benchmarks to test how well VLMs can interpret these images. This lack of evaluation tools makes it difficult to improve these models and ensure they are effective for scientific research.
What's the solution?
To solve this issue, the authors created μ-Bench, which includes 22 specific tasks that cover various aspects of biomedical research using different types of microscopy (like electron and fluorescence). They tested several VLMs on this benchmark and found that many models struggled with even basic tasks, such as telling different microscopy techniques apart. They also discovered that specialized models trained on biomedical data sometimes performed worse than general models. To address the problem of 'catastrophic forgetting'—where models lose previously learned information when trained on new data—they suggested combining weights from both fine-tuned and pre-trained models to improve overall performance.
Why it matters?
This research is important because it provides a much-needed tool for evaluating and improving VLMs in microscopy. By creating a comprehensive benchmark, μ-Bench helps researchers identify weaknesses in current models and encourages the development of better tools for analyzing biological images, ultimately aiding scientific discovery.
Abstract
Recent advances in microscopy have enabled the rapid generation of terabytes of image data in cell biology and biomedical research. Vision-language models (VLMs) offer a promising solution for large-scale biological image analysis, enhancing researchers' efficiency, identifying new image biomarkers, and accelerating hypothesis generation and scientific discovery. However, there is a lack of standardized, diverse, and large-scale vision-language benchmarks to evaluate VLMs' perception and cognition capabilities in biological image understanding. To address this gap, we introduce {\mu}-Bench, an expert-curated benchmark encompassing 22 biomedical tasks across various scientific disciplines (biology, pathology), microscopy modalities (electron, fluorescence, light), scales (subcellular, cellular, tissue), and organisms in both normal and abnormal states. We evaluate state-of-the-art biomedical, pathology, and general VLMs on {\mu}-Bench and find that: i) current models struggle on all categories, even for basic tasks such as distinguishing microscopy modalities; ii) current specialist models fine-tuned on biomedical data often perform worse than generalist models; iii) fine-tuning in specific microscopy domains can cause catastrophic forgetting, eroding prior biomedical knowledge encoded in their base model. iv) weight interpolation between fine-tuned and pre-trained models offers one solution to forgetting and improves general performance across biomedical tasks. We release {\mu}-Bench under a permissive license to accelerate the research and development of microscopy foundation models.