BenchX: A Unified Benchmark Framework for Medical Vision-Language Pretraining on Chest X-Rays
Yang Zhou, Tan Li Hui Faith, Yanyu Xu, Sicong Leng, Xinxing Xu, Yong Liu, Rick Siow Mong Goh
2024-11-01
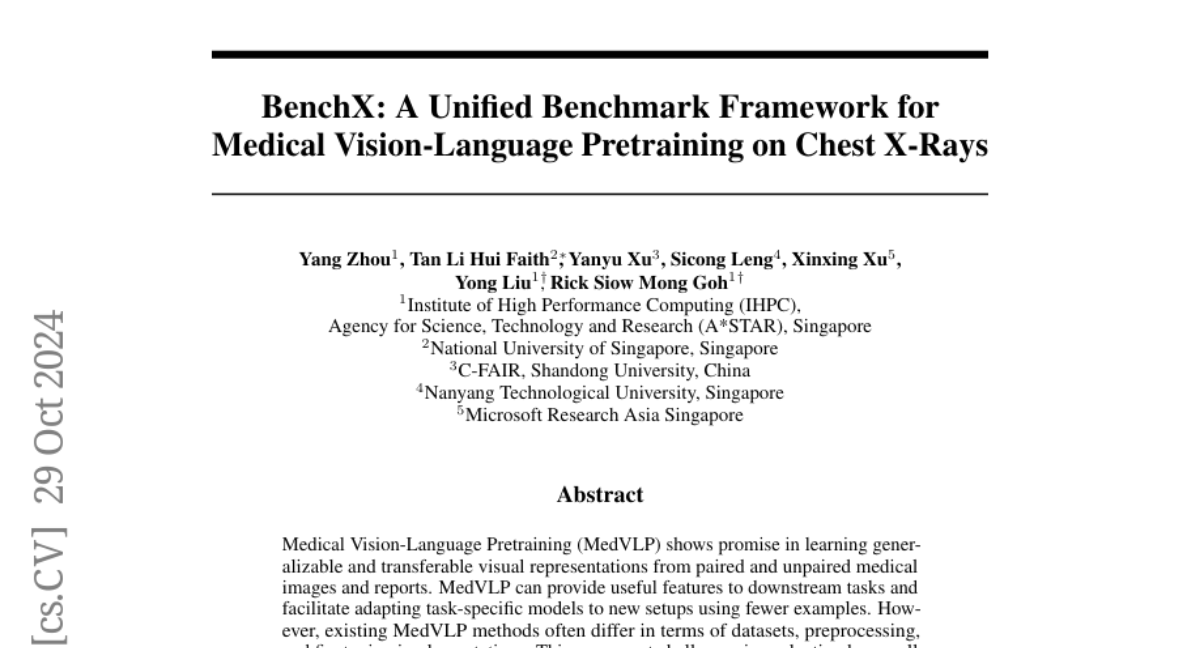
Summary
This paper introduces BenchX, a new framework designed to evaluate and compare different Medical Vision-Language Pretraining (MedVLP) methods using chest X-ray images. It aims to standardize how these methods are tested so that their effectiveness can be more easily measured.
What's the problem?
There are many different MedVLP methods available, but they often use different datasets and techniques for processing data. This makes it hard to know which method works best for various medical tasks, as there's no unified way to evaluate them.
What's the solution?
BenchX provides a comprehensive benchmarking framework that includes three main parts: a collection of datasets covering multiple medical tasks, standardized procedures for preparing and splitting data, and consistent protocols for fine-tuning models. This allows researchers to directly compare the performance of different MedVLP methods on the same tasks.
Why it matters?
This framework is important because it helps improve the reliability of MedVLP methods in medical applications. By providing a standardized way to measure performance, BenchX can lead to better models that assist in diagnosing diseases from X-ray images, ultimately improving patient care.
Abstract
Medical Vision-Language Pretraining (MedVLP) shows promise in learning generalizable and transferable visual representations from paired and unpaired medical images and reports. MedVLP can provide useful features to downstream tasks and facilitate adapting task-specific models to new setups using fewer examples. However, existing MedVLP methods often differ in terms of datasets, preprocessing, and finetuning implementations. This pose great challenges in evaluating how well a MedVLP method generalizes to various clinically-relevant tasks due to the lack of unified, standardized, and comprehensive benchmark. To fill this gap, we propose BenchX, a unified benchmark framework that enables head-to-head comparison and systematical analysis between MedVLP methods using public chest X-ray datasets. Specifically, BenchX is composed of three components: 1) Comprehensive datasets covering nine datasets and four medical tasks; 2) Benchmark suites to standardize data preprocessing, train-test splits, and parameter selection; 3) Unified finetuning protocols that accommodate heterogeneous MedVLP methods for consistent task adaptation in classification, segmentation, and report generation, respectively. Utilizing BenchX, we establish baselines for nine state-of-the-art MedVLP methods and found that the performance of some early MedVLP methods can be enhanced to surpass more recent ones, prompting a revisiting of the developments and conclusions from prior works in MedVLP. Our code are available at https://github.com/yangzhou12/BenchX.