BetterDepth: Plug-and-Play Diffusion Refiner for Zero-Shot Monocular Depth Estimation
Xiang Zhang, Bingxin Ke, Hayko Riemenschneider, Nando Metzger, Anton Obukhov, Markus Gross, Konrad Schindler, Christopher Schroers
2024-07-26
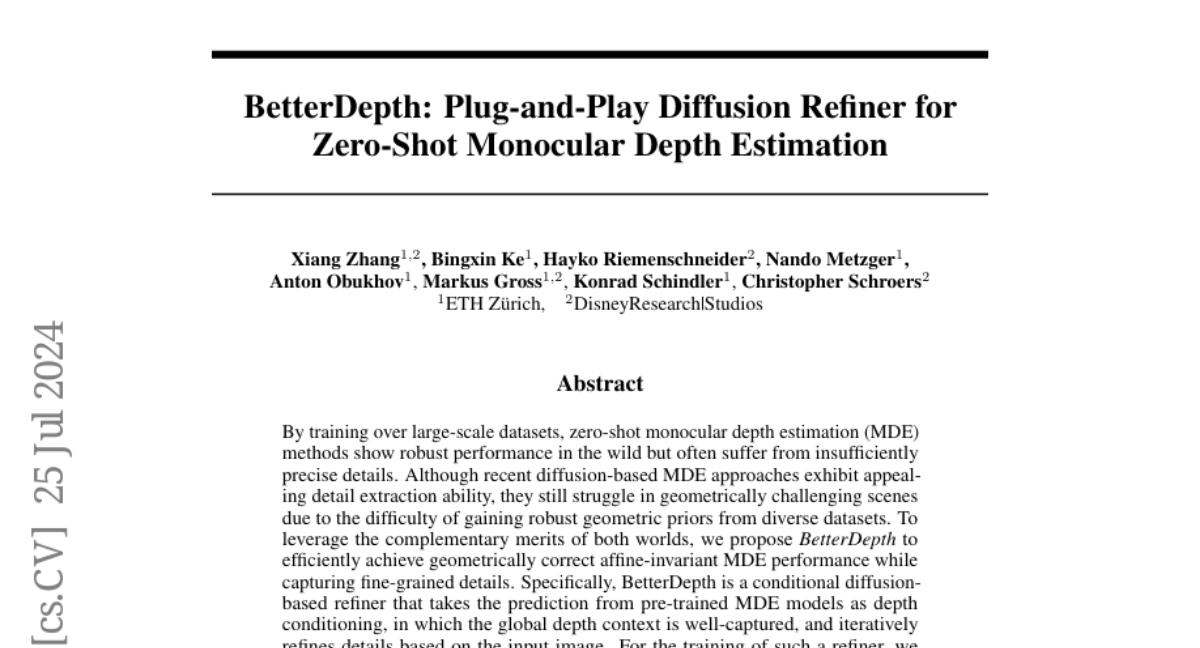
Summary
This paper introduces BetterDepth, a new method for estimating depth from single images using advanced AI techniques. It aims to improve the accuracy and detail of depth estimation, which is important for various applications in computer vision.
What's the problem?
Estimating depth from a single image (monocular depth estimation) can be challenging because existing methods often struggle to provide precise details, especially in complex scenes. While some newer methods show promise in capturing details, they still have difficulties with geometry in complicated environments, leading to less accurate depth information.
What's the solution?
BetterDepth combines the strengths of previous methods by using a conditional diffusion-based approach. It takes initial depth predictions from pre-trained models and refines them iteratively based on the input image. The researchers developed techniques called global pre-alignment and local patch masking to ensure that BetterDepth accurately captures details while staying true to the depth data. This method was trained efficiently on smaller synthetic datasets and shows excellent performance on various real-world datasets without needing extensive retraining.
Why it matters?
BetterDepth is significant because it enhances the ability of AI systems to understand and interpret images more accurately, which is crucial for applications like robotics, augmented reality, and autonomous vehicles. By improving how depth is estimated from single images, this research helps make technology smarter and more reliable in navigating and understanding our world.
Abstract
By training over large-scale datasets, zero-shot monocular depth estimation (MDE) methods show robust performance in the wild but often suffer from insufficiently precise details. Although recent diffusion-based MDE approaches exhibit appealing detail extraction ability, they still struggle in geometrically challenging scenes due to the difficulty of gaining robust geometric priors from diverse datasets. To leverage the complementary merits of both worlds, we propose BetterDepth to efficiently achieve geometrically correct affine-invariant MDE performance while capturing fine-grained details. Specifically, BetterDepth is a conditional diffusion-based refiner that takes the prediction from pre-trained MDE models as depth conditioning, in which the global depth context is well-captured, and iteratively refines details based on the input image. For the training of such a refiner, we propose global pre-alignment and local patch masking methods to ensure the faithfulness of BetterDepth to depth conditioning while learning to capture fine-grained scene details. By efficient training on small-scale synthetic datasets, BetterDepth achieves state-of-the-art zero-shot MDE performance on diverse public datasets and in-the-wild scenes. Moreover, BetterDepth can improve the performance of other MDE models in a plug-and-play manner without additional re-training.