Bielik 7B v0.1: A Polish Language Model -- Development, Insights, and Evaluation
Krzysztof Ociepa, Łukasz Flis, Krzysztof Wróbel, Adrian Gwoździej, Remigiusz Kinas
2024-10-29
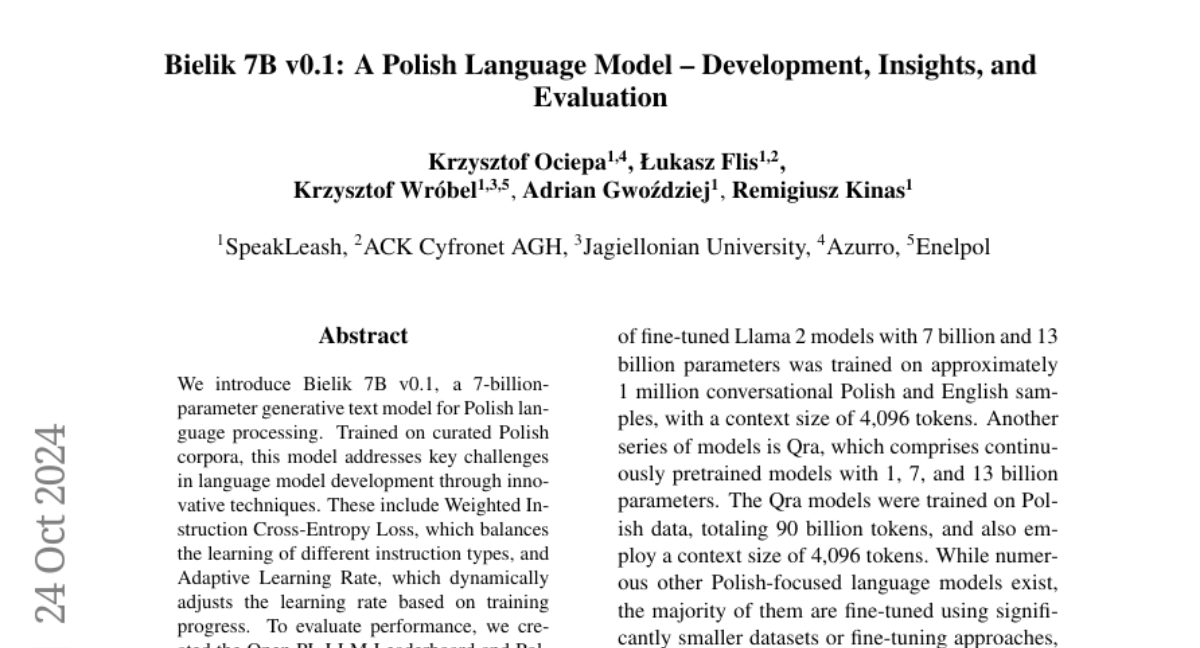
Summary
This paper introduces Bielik 7B v0.1, a new Polish language model designed to improve natural language processing tasks by utilizing advanced training techniques.
What's the problem?
Developing effective language models for Polish has been challenging due to the need for large amounts of high-quality training data and sophisticated methods to handle various language tasks. Existing models often struggle with performance in certain areas, such as reasoning and role-playing, which limits their usefulness in real-world applications.
What's the solution?
The authors present Bielik 7B v0.1, a generative text model with 7 billion parameters that has been specifically trained on curated Polish text data. They implement innovative techniques like Weighted Instruction Cross-Entropy Loss to balance different types of instructions during training and an Adaptive Learning Rate that adjusts as the model learns. To evaluate its performance, they created the Open PL LLM Leaderboard and Polish MT-Bench, which assess various natural language processing tasks. The results show that Bielik 7B v0.1 significantly outperforms previous models, especially in reasoning and role-playing tasks.
Why it matters?
This research is important because it represents a significant advancement in AI for the Polish language, providing a powerful tool for applications like chatbots, translation services, and other linguistic technologies. By setting new benchmarks in performance, Bielik 7B v0.1 can help improve how AI understands and generates Polish text.
Abstract
We introduce Bielik 7B v0.1, a 7-billion-parameter generative text model for Polish language processing. Trained on curated Polish corpora, this model addresses key challenges in language model development through innovative techniques. These include Weighted Instruction Cross-Entropy Loss, which balances the learning of different instruction types, and Adaptive Learning Rate, which dynamically adjusts the learning rate based on training progress. To evaluate performance, we created the Open PL LLM Leaderboard and Polish MT-Bench, novel frameworks assessing various NLP tasks and conversational abilities. Bielik 7B v0.1 demonstrates significant improvements, achieving a 9 percentage point increase in average score compared to Mistral-7B-v0.1 on the RAG Reader task. It also excels in the Polish MT-Bench, particularly in Reasoning (6.15/10) and Role-playing (7.83/10) categories. This model represents a substantial advancement in Polish language AI, offering a powerful tool for diverse linguistic applications and setting new benchmarks in the field.