BiGym: A Demo-Driven Mobile Bi-Manual Manipulation Benchmark
Nikita Chernyadev, Nicholas Backshall, Xiao Ma, Yunfan Lu, Younggyo Seo, Stephen James
2024-07-11
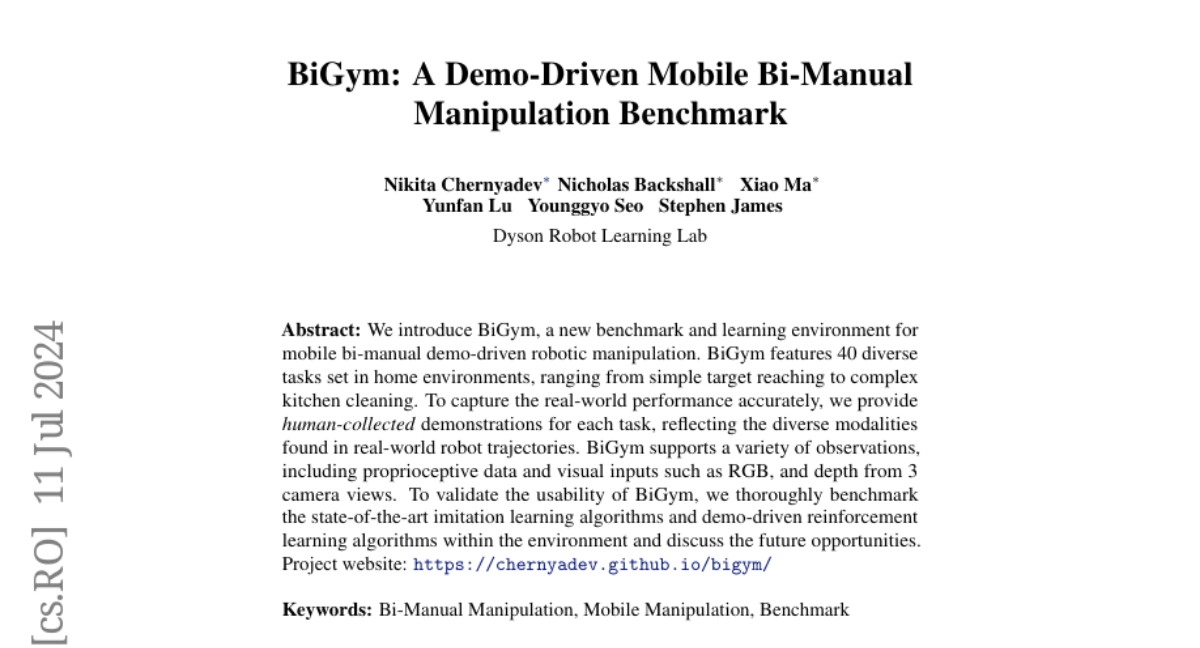
Summary
This paper introduces BiGym, a new testing and learning platform designed for robots that use both hands to perform tasks. It includes 40 different tasks that robots can learn to do in home settings, such as reaching for objects or cleaning the kitchen.
What's the problem?
Robots often struggle with learning how to manipulate objects in realistic environments because existing benchmarks either focus on simple, single-arm tasks or rely on artificial demonstrations that don't reflect real-world scenarios. This limits their ability to learn effectively and perform complex actions that require using both hands.
What's the solution?
BiGym addresses this problem by providing a variety of tasks that involve real human demonstrations, capturing how people naturally perform these actions. The platform supports different types of observations, such as visual data from cameras and information about the robot's movements. By using this realistic data, BiGym helps researchers evaluate and improve both imitation learning (where robots learn by copying humans) and reinforcement learning (where robots learn through trial and error).
Why it matters?
This research is important because it creates a more effective way for robots to learn complex tasks in environments similar to those they will encounter in the real world. By improving how robots can manipulate objects using both hands, BiGym can lead to advancements in robotics that enhance automation in everyday tasks, making robots more useful in homes and other settings.
Abstract
We introduce BiGym, a new benchmark and learning environment for mobile bi-manual demo-driven robotic manipulation. BiGym features 40 diverse tasks set in home environments, ranging from simple target reaching to complex kitchen cleaning. To capture the real-world performance accurately, we provide human-collected demonstrations for each task, reflecting the diverse modalities found in real-world robot trajectories. BiGym supports a variety of observations, including proprioceptive data and visual inputs such as RGB, and depth from 3 camera views. To validate the usability of BiGym, we thoroughly benchmark the state-of-the-art imitation learning algorithms and demo-driven reinforcement learning algorithms within the environment and discuss the future opportunities.