BitStack: Fine-Grained Size Control for Compressed Large Language Models in Variable Memory Environments
Xinghao Wang, Pengyu Wang, Bo Wang, Dong Zhang, Yunhua Zhou, Xipeng Qiu
2024-11-01
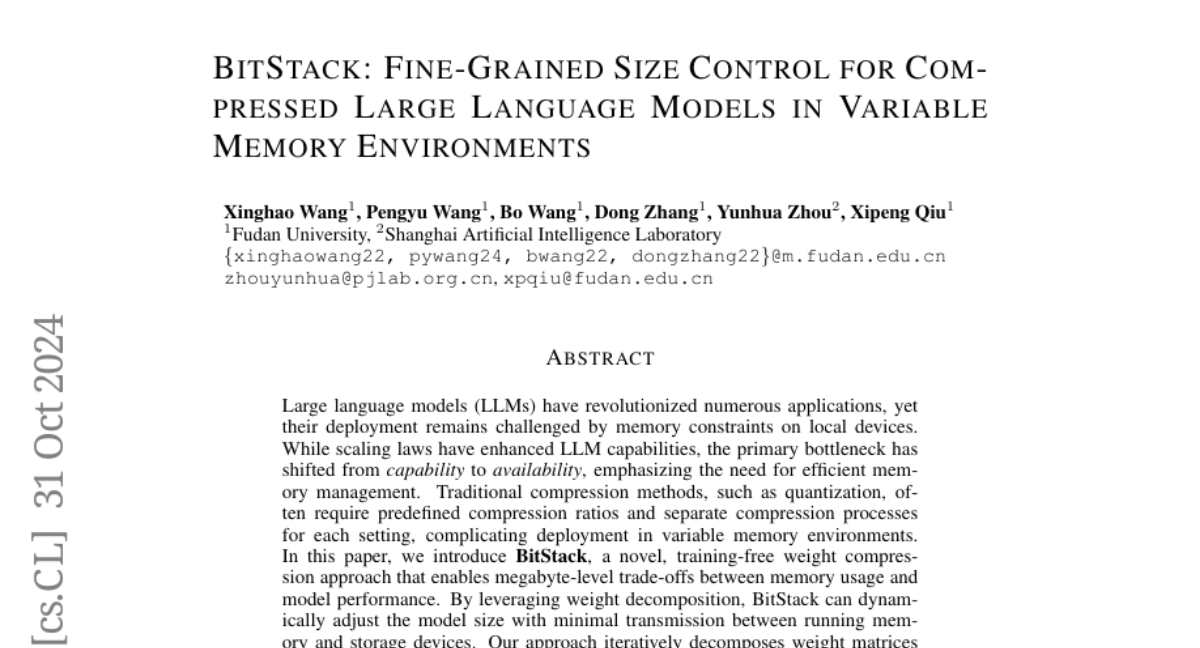
Summary
This paper presents BitStack, a new method for compressing large language models (LLMs) to fit better in devices with limited memory, allowing for flexible adjustments between memory use and model performance.
What's the problem?
Large language models are powerful but often too big to run on personal devices like laptops or smartphones due to their high memory requirements. Traditional methods for compressing these models can be rigid and require a lot of setup, making it hard to adapt them to different memory situations. This means that users might not be able to use the full capabilities of these models if their device doesn't have enough memory.
What's the solution?
BitStack introduces a more flexible approach to model compression that doesn't require extensive retraining. It breaks down the model into smaller parts called weight matrices, which can be adjusted based on how much memory is available at any given time. This allows the model to load only the necessary parts, optimizing performance without overloading the device's memory. The method can dynamically change how much of the model is loaded depending on the current memory situation, providing a balance between performance and resource use.
Why it matters?
This research is important because it makes it easier to deploy powerful language models on everyday devices, ensuring that more people can access advanced AI technology without needing expensive hardware. By allowing for better memory management, BitStack can help improve applications in various fields, such as personal assistants, educational tools, and more.
Abstract
Large language models (LLMs) have revolutionized numerous applications, yet their deployment remains challenged by memory constraints on local devices. While scaling laws have enhanced LLM capabilities, the primary bottleneck has shifted from capability to availability, emphasizing the need for efficient memory management. Traditional compression methods, such as quantization, often require predefined compression ratios and separate compression processes for each setting, complicating deployment in variable memory environments. In this paper, we introduce BitStack, a novel, training-free weight compression approach that enables megabyte-level trade-offs between memory usage and model performance. By leveraging weight decomposition, BitStack can dynamically adjust the model size with minimal transmission between running memory and storage devices. Our approach iteratively decomposes weight matrices while considering the significance of each parameter, resulting in an approximately 1-bit per parameter residual block in each decomposition iteration. These blocks are sorted and stacked in storage as basic transmission units, with different quantities loaded based on current memory availability. Extensive experiments across a wide range of tasks demonstrate that, despite offering fine-grained size control, BitStack consistently matches or surpasses strong quantization baselines, particularly at extreme compression ratios. To the best of our knowledge, this is the first decomposition-based method that effectively bridges the gap to practical compression techniques like quantization. Code is available at https://github.com/xinghaow99/BitStack.